Energy Demand Forecasting: A Review on Methodologies and Technique
Diksha Rai1 , Vandan Tewari2
Section:Review Paper, Product Type: Journal Paper
Volume-7 ,
Issue-5 , Page no. 215-218, May-2019
CrossRef-DOI: https://doi.org/10.26438/ijcse/v7i5.215218
Online published on May 31, 2019
Copyright © Diksha Rai, Vandan Tewari . This is an open access article distributed under the Creative Commons Attribution License, which permits unrestricted use, distribution, and reproduction in any medium, provided the original work is properly cited.
View this paper at Google Scholar | DPI Digital Library
How to Cite this Paper
- IEEE Citation
- MLA Citation
- APA Citation
- BibTex Citation
- RIS Citation
IEEE Style Citation: Diksha Rai, Vandan Tewari, “Energy Demand Forecasting: A Review on Methodologies and Technique,” International Journal of Computer Sciences and Engineering, Vol.7, Issue.5, pp.215-218, 2019.
MLA Style Citation: Diksha Rai, Vandan Tewari "Energy Demand Forecasting: A Review on Methodologies and Technique." International Journal of Computer Sciences and Engineering 7.5 (2019): 215-218.
APA Style Citation: Diksha Rai, Vandan Tewari, (2019). Energy Demand Forecasting: A Review on Methodologies and Technique. International Journal of Computer Sciences and Engineering, 7(5), 215-218.
BibTex Style Citation:
@article{Rai_2019,
author = {Diksha Rai, Vandan Tewari},
title = {Energy Demand Forecasting: A Review on Methodologies and Technique},
journal = {International Journal of Computer Sciences and Engineering},
issue_date = {5 2019},
volume = {7},
Issue = {5},
month = {5},
year = {2019},
issn = {2347-2693},
pages = {215-218},
url = {https://www.ijcseonline.org/full_paper_view.php?paper_id=4225},
doi = {https://doi.org/10.26438/ijcse/v7i5.215218}
publisher = {IJCSE, Indore, INDIA},
}
RIS Style Citation:
TY - JOUR
DO = {https://doi.org/10.26438/ijcse/v7i5.215218}
UR - https://www.ijcseonline.org/full_paper_view.php?paper_id=4225
TI - Energy Demand Forecasting: A Review on Methodologies and Technique
T2 - International Journal of Computer Sciences and Engineering
AU - Diksha Rai, Vandan Tewari
PY - 2019
DA - 2019/05/31
PB - IJCSE, Indore, INDIA
SP - 215-218
IS - 5
VL - 7
SN - 2347-2693
ER -
![]() |
![]() |
![]() |
320 | 330 downloads | 155 downloads |
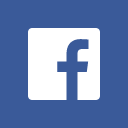
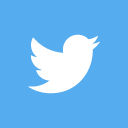
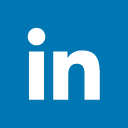
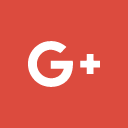
Abstract
Nowadays, a country’s economy development is estimated from tracking light from space at night. Therefore, electricity has become a major factor in determining national economy. Accurate models have become necessary to help electricity companies to forecast load in advance so that electricity is always present in every corner of a country. In this paper, we have made an attempt to review electricity load forecasting techniques. This review paper overviews the existing electricity demands prediction approaches such as traditional approaches, statistical approaches and machine learning based approaches. It further presents the pros and cons of various techniques. It also presents the challenges of this predictive analysis.
Key-Words / Index Term
Prediction, smart grid, artificial neural network, short-term load forecasting
References
[1] H. S. Hippert, C. E. Pedreira, and C. R. Souza, “Neural Networks for Short-Term Load Forecasting: A review and Evaluation”, IEEE Trans. Power Syst., vol. 16, no. 1, pp. 44-51, 2001.
[2] Box GEP, Jenkins GM, “Time series analysis, forecasting and control”, Holden-Day, San Francisco, CA, 1970.
[3] Hamid R. Khosravani, Maria Del Mar Castilla, Manuel Berenguel, Antonio E. Ruano and Pedro M. Ferreira, “A comparison of Energy Consumption Prediction model based on Neural Networks of a Bioclimatic Building”, Energies, 2016.
[4] Kangji Li, Chenglei Hu, Guohai Liu, Wenping Xue, “Building electricity consumption prediction using optimized artificial neural networks and principle component analysis”, Energy and Buildings, vol. 108, 2015 pp 106-113.
[5] Jihoon Moon, Jinwoong Park, Eenjun Hwang, Sanghoon Jun, “Forecasting power consumption for higher educational institutions based on machine learning”, Springer, 2017.
[6] S. Saravanan, S. Kannan and C. Thangaraj, “Forecasting India’s Electricity Demand Using Artificial Neural Network”, IEEE- International Conference on Advances in Engineering, Science And Management (ICAESM-2012), March 30, 31, 2012.
[7] Rodrigo F. Berriel, Andre Teixeira Lopes, Alexandre Rodrigues, Flavio Miguel Varejao, Thiago Oliveira-Santos, “Monthly Energy Consumption Forecast: A Deep Learning Approach”, International Joint Conference on Neural Networks, 2017.
[8] Wei Yu, Dou An David Griffith, Qingyu Yang and Guobin Xu, “Towards Statistical Modelling and Machine Learning Based Energy Usage Forecasting in Smart Grid”, ACM, 2015.
[9] Shu Fan and Rob J Hyndman, “Short-term load forecasting based on a semi-parametric additive model”, IEEE Transactions on Power Systems, 2010.
[10] Vincent Thouvenot, Audrey Pichavant, Yannig Goude, Anestis Antoniadis and Jean-Michel Poggi, “Electricity Forecasting using Multi-Stage Estimators of Nonlinear Additive Models”, IEEE Transactions on Power Systems, vol. 31, no. 5, September 2016.