A Rigorous Review on an Ensemble Based Data Stream Drift Classification Methods
R. C. Samant1 , D. M. Thakore2
Section:Review Paper, Product Type: Journal Paper
Volume-7 ,
Issue-5 , Page no. 380-385, May-2019
CrossRef-DOI: https://doi.org/10.26438/ijcse/v7i5.380385
Online published on May 31, 2019
Copyright © R. C. Samant, D. M. Thakore . This is an open access article distributed under the Creative Commons Attribution License, which permits unrestricted use, distribution, and reproduction in any medium, provided the original work is properly cited.
View this paper at Google Scholar | DPI Digital Library
How to Cite this Paper
- IEEE Citation
- MLA Citation
- APA Citation
- BibTex Citation
- RIS Citation
IEEE Style Citation: R. C. Samant, D. M. Thakore, “A Rigorous Review on an Ensemble Based Data Stream Drift Classification Methods,” International Journal of Computer Sciences and Engineering, Vol.7, Issue.5, pp.380-385, 2019.
MLA Style Citation: R. C. Samant, D. M. Thakore "A Rigorous Review on an Ensemble Based Data Stream Drift Classification Methods." International Journal of Computer Sciences and Engineering 7.5 (2019): 380-385.
APA Style Citation: R. C. Samant, D. M. Thakore, (2019). A Rigorous Review on an Ensemble Based Data Stream Drift Classification Methods. International Journal of Computer Sciences and Engineering, 7(5), 380-385.
BibTex Style Citation:
@article{Samant_2019,
author = {R. C. Samant, D. M. Thakore},
title = {A Rigorous Review on an Ensemble Based Data Stream Drift Classification Methods},
journal = {International Journal of Computer Sciences and Engineering},
issue_date = {5 2019},
volume = {7},
Issue = {5},
month = {5},
year = {2019},
issn = {2347-2693},
pages = {380-385},
url = {https://www.ijcseonline.org/full_paper_view.php?paper_id=4252},
doi = {https://doi.org/10.26438/ijcse/v7i5.380385}
publisher = {IJCSE, Indore, INDIA},
}
RIS Style Citation:
TY - JOUR
DO = {https://doi.org/10.26438/ijcse/v7i5.380385}
UR - https://www.ijcseonline.org/full_paper_view.php?paper_id=4252
TI - A Rigorous Review on an Ensemble Based Data Stream Drift Classification Methods
T2 - International Journal of Computer Sciences and Engineering
AU - R. C. Samant, D. M. Thakore
PY - 2019
DA - 2019/05/31
PB - IJCSE, Indore, INDIA
SP - 380-385
IS - 5
VL - 7
SN - 2347-2693
ER -
![]() |
![]() |
![]() |
280 | 183 downloads | 99 downloads |
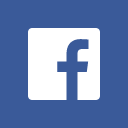
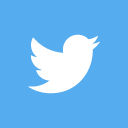
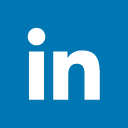
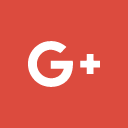
Abstract
incurrent era of big data, thedata analytics has become more challenging issue. Data get mined for finding facts as well as to predict impact of various activities which is used everywhere in the life. Mining processes like classification and clustering becomes more crucial in case of dynamic data streams. As the nature of data stream is temporal there is always a difference in the concepts which causes a concept drift. This concept drift affects the reliability of classifiers and clustering methods. Classification is important technique in data mining, which has been applied with various modifications to handle concept drift issue. Data stream classification is different from normal classification process as it has restriction of time, memory size and speed of processing along with accuracy. This article presents a review of remarkable recent ensemble based classifiers, which are designed to detect concept drift based on the different way of data stream processing.
Key-Words / Index Term
Ensemble Learning, Data Stream Mining, Concept Drift
References
[1] https://searchdatamanagement.techtarget.com/definition/big-data.
[2] Read, J., Bifet, A., Holmes, G., & Pfahringer, B. “Efficient multi-label classification for evolving data streams.” Technical Report University of Waikato, Department of Computer Science, 2010.
[3] I. Khan, J.Z. Huang, K. Ivanov. ”Incremental density-based ensemble clustering over evolving data streams.” Neurocomputing, 191, 34-43, 2016.
[4] I. Zliobaite, A. Bifet, B. Pfahringer, G. Holmes, “Active learning with drifting streaming data”, IEEE Transactions on Neural Networks & Learning Systems, 25, 27-39,2014.
[5] https://en.wikipedia.org/wiki/Concept_drift 09-22-2018.
[6] Zliobaite I.”Learning under concept drift: an overview.” Technical report faculty of mathematics and informatics, Vilnius University, Vilnius, Lithuania, 2009.
[7] Wang H., FanW., Yu P. and Han J. “Mining Concept-Drifting Data Streams using Ensemble Classifiers.” in the 9th ACM International Conference on Knowledge Discovery and Data Mining (SIGKDD), Washington DC, USA, 2003.
[8] D. Brzezinski, J. Stefanowski. “Reacting to different types of concept drift: the accuracy updated ensemble algorithm.” IEEE Trans. Neural Netw. Learn. Syst. 25 (1) 81–94, 2014.
[9] D. Brzezinski , J. Stefanowski. “Prequential AUC: properties of the area under the ROC curve for data streams with concept drift.” in Knowledge and Information Systems 52(2):531-562 • January 2017.
[10] Heitor Murilo Gomes, Jean Paul Barddal, And Fabr´Icio Enembreck.”A Survey on Ensemble Learning for Data Stream Classification.”ACM Computing Surveys, Vol. 50, No. 2, Article 23, March 2017.
[11] N.C. Oza and S. Russell. “Online bagging and boosting.”, Proceedings of the Eighth International Workshop on Artificial Intelligence and Statistics (AIS- TATS’01), Morgan Kaufmann, Key West, USA, p. 105-112,2001.
[12] Nikunj C. Oza and Stuart Russell. ‘‘Experimental Comparisons of Online and Batch Versions of Bagging and Boosting.” The Seventh ACM SIGKDD International Conference on Knowledge Discovery and Data Mining, San Francisco, CA, USA, pp. 359-364, August 2001.
[13] Bifet, A., Holmes, G., Pfahringer, B., Kirkby, R., Gavald`a, R.: New ensemble methods for evolving data streams. In: KDD, pp. 139–148 2009.
[14] A. Bifet, G. Holmes, and B. Pfahringer, “Leveraging bagging for evolving data streams,” in Proc. ECML/PKDD, I, pp. 135–150,2010.
[15] S. G. T. de Carvalho Santos, P. M. G. Júnior, G. D. dos Santos Silva, and R. S. M. de Barros, “Speeding up recovery from concept drifts,” in Proc. Eur. Conf. Mach. Learn. Princ. Pract. Knowl. Discovery Databases, pp. 179–194,2014.
[16] R. S. M. de Barros, S. G. T. de Carvalho Santos, P. M. G. Júnior, “A boosting-like online learning ensemble,” in Proc. IEEE IJCNN, Jul. 2016, pp. 1871–1878.
[17] I. Frías-Blanco, A. Verdecia-Cabrera, A. Ortiz-Díaz, and A. Carvalho, “Fast adaptive stacking of ensembles,” in Proc. ACM Symp. Appl. Comput., 2016, pp. 929–934.
[18] I. Fr´ıas, J. del Campo, G. Ramos, R. Morales, A. Ortiz, and Y. Caballero. “Online and non-parametric drift detection methods based on Hoeffding’s bounds.” IEEE Transactions on Knowledge and Data Engineering, 14(3):810–823, 2015.
[19] J. Gama, P. Rodrigues, and G. Castillo. “Evaluating Algorithms that Learn from Data Streams.” In Proc. 2009 ACM Symposium on Applied Computing, pages 1496–1500, 2009.
[20] Jicheng Shan , Hang Zhang, Weike Liu, and Qingbao Liu. “Online Active Learning Ensemble Framework for Drifted Data Streams.”, in Proc. IEEE Transactions On Neural Networks And Learning Systems, 2018, pp.1 – 13.
[21] W. N. Street and Y. Kim. “A streaming ensemble algorithm (sea) for large-scale classification.” ACM KDD Conference, pages 377–382, 2001.
[22] J. R. Quinlan. C4.5: programs for machine learning. Morgan Kaufmann, San Mateo, CA, 1993.
[23] Jeremy Z. Kolter and Marcus A. Maloof. “Using additive expert ensembles to cope with concept drift.” In Proceedings of the 22nd International Conference on Machine Learning (ICML’05). ACM, New York, NY, 2005, 449–456.
[24] Kolter, M.A. Maloof. “Dynamic weighted majority: an ensemble method for drifting concepts.” Journal of Machine Learning Research 8 (2007) 2755-2790.
[25] P. E. Utgoff, N. C. Berkman, and J. A. Clouse.”Decision tree induction based on efficient tree restructuring.” Machine Learning, 29:5–44, 1997.
[26] Gregory Ditzler, Michael D. Muhlbaier, and Robi Polikar. Incremental learning of new classes in unbalanced datasets: Learn++.UDNC. In Multiple Classifier Systems. Lecture Notes in Computer Science, Vol. 5997. Springer, Berlin, 33–42, 2010.
[27] Magdalena Deckert. “Batch weighted ensemble for mining data streams with concept drift.” In Foundations of Intelligent Systems. Springer, 290–299, 2011.
[28] Ryan Elwell and Robi Polikar. “Incremental learning of concept drift in nonstationary environments.” IEEE Trans. Neur. Netw. 22, 10 (2011), 1517–1531, 2011.
[29] Leandro L. Minku and Xin Yao. “ DDD: A new ensemble approach for dealing with concept drift. “ IEEE Trans. Knowl. Data Eng. 24, 4, 2012.
[30] M. Baena-Garcia, J. Del Campo-_ Avila, R. Fidalgo, and A. Bifet, “Early Drift Detection Method,” Proc. Fourth ECML PKDD Int. Workshop Knowledge Discovery from Data Streams (IWKDDS ’06),pp. 77-86, 2006.
[31] Kyosuke Nishida, Koichiro Yamauchi, and Takashi Omori Ace: Adaptive classifiers-ensemble system for concept-drifting environments. In Multiple Classifier Systems. Springer, 176–185, 2005.
[32] Mohammad M. Masud, Jing Gao, Latifur Khan, Jiawei Han, and Bhavani Thuraisingham. “A practical approach to classify evolving data streams: Training with limited amount of labeled data.” In 8th IEEE International Conference on Data Mining, 2008 (ICDM’08). IEEE, 929–934, 2008.
[33] Ioannis Katakis, Grigorios Tsoumakas, and Ioannis Vlahavas. “Tracking recurring contexts using ensemble classifiers: An application to email filtering.” Knowl. Inf. Syst. 22, 3,371–391, 2010.
[34] MohammadM.Masud, Qing Chen, Jing Gao, Latifur Khan, Jiawei Han, and Bhavani Thuraisingham. “Classification and novel class detection of data streams in a dynamic feature space.” In Machine Learningand Knowledge Discovery in Databases. Springer, 337–352. 2010.
[35] Peng Zhang, Xingquan Zhu, Yong Shi, Li Guo, and XindongWu.” Robust ensemble learning for mining noisy data streams.” Dec. Supp. Syst. 50, 2 469–479, 2011.
[36] Ghazal Jaber, Antoine Cornu´ejols, and Philippe Tarroux. “ Online learning: Searching for the best forgetting strategy under concept drift.” In Neural Information Processing. Springer, 400–408, 2013.
[37] Ghazal Jaber, Antoine Cornu´ejols, and Philippe Tarroux. A new on-line learning method for coping with recurring concepts: The ADACC system. In Neural Information Processing. Springer, 595–604. 2013.
[38] Dariusz Brzezinski and Jerzy Stefanowski. 2014. Combining block-based and online methods in learning ensembles from concept drifting data streams. Inf. Sci. 265,50–67, 2014.
[39] Alina Beygelzimer, Satyen Kale, and Haipeng Luo. “Optimal and adaptive algorithms for online boosting.” 2323–2331, 2015.
[40] Yoav Freund, Robert E. Schapire,” Experiments with a new boosting algorithm”, In ICML,Vol. 96. 148–156. 1996.
[41] Pratap Shinde; M. D. Ingle, "Streaming Data Clustering using Incremental Affine Propagation Clustering Approach", International Journal of Science and Research (IJSR), https://www.ijsr.net/search_index_results_paperid.php?id=SUB156911, Volume 4 Issue 7, July 2015, 2209 – 2212.
[42] Spraha Kamriya; Vandana Kate, "Live Data Stream Classification for Reducing Query Processing Time: Design and Analysis", International Journal of Science and Research (IJSR), https://www.ijsr.net/search_index_results_paperid.php?id=ART20174017, Volume 6 Issue 6, June 2017, 1711 – 1716.