Analysis of Tumor Detection Methods for Mammogram Images
S. Bhadra1
Section:Research Paper, Product Type: Journal Paper
Volume-7 ,
Issue-5 , Page no. 431-435, May-2019
CrossRef-DOI: https://doi.org/10.26438/ijcse/v7i5.431435
Online published on May 31, 2019
Copyright © S. Bhadra . This is an open access article distributed under the Creative Commons Attribution License, which permits unrestricted use, distribution, and reproduction in any medium, provided the original work is properly cited.
View this paper at Google Scholar | DPI Digital Library
How to Cite this Paper
- IEEE Citation
- MLA Citation
- APA Citation
- BibTex Citation
- RIS Citation
IEEE Style Citation: S. Bhadra, “Analysis of Tumor Detection Methods for Mammogram Images,” International Journal of Computer Sciences and Engineering, Vol.7, Issue.5, pp.431-435, 2019.
MLA Style Citation: S. Bhadra "Analysis of Tumor Detection Methods for Mammogram Images." International Journal of Computer Sciences and Engineering 7.5 (2019): 431-435.
APA Style Citation: S. Bhadra, (2019). Analysis of Tumor Detection Methods for Mammogram Images. International Journal of Computer Sciences and Engineering, 7(5), 431-435.
BibTex Style Citation:
@article{Bhadra_2019,
author = {S. Bhadra},
title = {Analysis of Tumor Detection Methods for Mammogram Images},
journal = {International Journal of Computer Sciences and Engineering},
issue_date = {5 2019},
volume = {7},
Issue = {5},
month = {5},
year = {2019},
issn = {2347-2693},
pages = {431-435},
url = {https://www.ijcseonline.org/full_paper_view.php?paper_id=4260},
doi = {https://doi.org/10.26438/ijcse/v7i5.431435}
publisher = {IJCSE, Indore, INDIA},
}
RIS Style Citation:
TY - JOUR
DO = {https://doi.org/10.26438/ijcse/v7i5.431435}
UR - https://www.ijcseonline.org/full_paper_view.php?paper_id=4260
TI - Analysis of Tumor Detection Methods for Mammogram Images
T2 - International Journal of Computer Sciences and Engineering
AU - S. Bhadra
PY - 2019
DA - 2019/05/31
PB - IJCSE, Indore, INDIA
SP - 431-435
IS - 5
VL - 7
SN - 2347-2693
ER -
![]() |
![]() |
![]() |
359 | 219 downloads | 101 downloads |
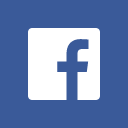
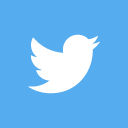
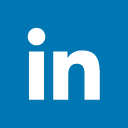
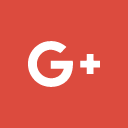
Abstract
Breast cancer remains the important reason of death among woman in the world. Early detection is essential to improve breast cancer diagnosis. Mammography is the reliable and best existing tool for investigation of breast cancer in its early stage. Understanding the mass region information of cancerous lesions in a mammogram is important for detection of the tumor region and its segmentation. In this paper, Maximum Mean and Least Variance method and Otsu method is implemented and then compared the results to find the suitable technique among them for detection and segmentation of tumor region.
Key-Words / Index Term
Breast cancer detection, Mammograms, Smoothing, Segmentation, Enhancement, Masses, Microcalcification
References
[1] J.S.L. Jasmine, S. Baskaran, A.Govardhan, “An Automated Mass Classification System in Digital Mammograms using Contourlet Transform and Support Vector Machine”, International Journal of Computer Applications, vol. 31, pp. 54-61, October 2011.
[2] H. Hahn, “ Wavelet Transforms For Detecting Microcalcification in Mammography”, 1995
[3] R. Kamath, K.S. Mahajan, L. Ashok, T.S. Sanal, “A Study on Risk Factors of Breast Cancer Among Patients Attending the Tertiary Care Hospital, in Udupi District”, Indian Journal of Community Medicine, 2013, pp. 95–99.
[4] M. M. Eltoukhy, I. Faye, B. B. Samir, “A comparison of wavelet and curvelet for breast cancer diagnosis in digital mammogram”, Computers in Biology and Medicine, 2010, pp. 384–391.
[5] K. Hu, X. Gao, F. Li, “Detection of Suspicious Lesions by Adaptive Thresholding Based on Multiresolution Analysis in Mammograms”, IEEE transactions on instrumentation and measurement, vol. 60, pp. 462-472, February 2011.
[6] H.D.Cheng, X. Cai, X. Chen, L. Hu, X. Lou, “Computer-aided detection and classifcation of microcalcifcations in mammograms: a survey”, Pattern Recognition, vol. 36, 2003, pp. 2967–2991.
[7] M. M. Eltoukhy, I. Faye, B. B. Samir ,“A statistical based feature extraction method for breast cancer diagnosis in digital mammogram using multiresolution representation”, Computers in Biology and Medicine , vol. 42, 2012, pp. 123–128
[8] A. K. Singh, B. Gupta, “A Novel Approach for Breast Cancer Detection and Segmentation in a Mammogram”, Procedia Computer Science , vol. 54, 2015, pp. 676 – 682.
[9] N. Al- Najdawi, M. Biltawi, S, Tedmori, “Mammogram Image Visual Enhancement, Mass Segmentation and Classification”, Applied Soft Computing , vol. 35, 2015, pp. 175-185.
[10] http://breast-cancer.ca/mass-chars/
[11] A. A. Rani, G. Rajagopal ,A. Jagadeeswaran , “Bi-Histogram Equalization with Brightness Preservation Using Contras Enhancement”, International Journal of Basics and Applied Sciences.
[12] S. Nimkar, S. Shrivastava and S, Varghese, “Contrast Enhancement and Brightness Preservation using Multi- Decomposition Histogram Equalization”, Signal & Image Processing : An International Journal (SIPIJ), vol.4, June 2013.
[13] J. A. Stark, “Adaptive Image Contrast Enhancement Using Generalization of Histogram Equalization”, IEEE transaction on image processing, vol.9, pp. 889-896, May 2000.
[14] A. Papadopoulos, D.I. Fotiadis, L. Costaridou, “Improvement of microcalcification cluster detection in mammography utilizing image enhancement techniques.”, Computers in Biology and Medicine, vol. 38, pp. 1045–1055, 2008.
[15] D. L. Phamy, C. Xu, J. L. Prince, “A Survey of Current Methods in Medical Image Segmentation”, Annual review of biomedical engineering, vol. 2, pp. 315-337, 2000.
[16] A. R. Dominguez, A. K. Nandi, “Detection of masses in mammograms via statistically based enhancement, multilevel-thresholding segmentation, and region selection”, Computerized Medical Imaging and Graphics, vol. 32, 2008, pp. 304–315.
[17] M. Al-Bayati, A. El-Zaart, “Mammogram Images Thresholding for Breast Cancer Detection Using Different Thresholding Methods”, vol. 2, 2013, pp. 72-77.
[18] R. C. Gonzalez, R. E. Woods, “Digital Image Processing”, Third Edition, PEARSON.
[19] P.L. Rosin, E. Ioannidis, “Evaluation of Global Image Thresholding for Change Detection”, Pattern Recognition Letters, vol 14, 2003, pp. 2345-2356.
[20] D.C. Pereira, R. P. Ramos, M.Z. Do.Nascimento, “Segmentation and Detection of Breast Cancer in Mammograms Combining Wavelets Analysis and Genetic Algorithms”, Computer methods and programs in biomedicine, vol. 1, 2014, pp. 88-101.