Role of Document Clustering For Forensic Analysis Investigation System
Vilas V Pichad1 , Sachin N Deshmukh2
Section:Review Paper, Product Type: Journal Paper
Volume-3 ,
Issue-3 , Page no. 116-120, Mar-2015
Online published on Mar 31, 2015
Copyright © Vilas V Pichad , Sachin N Deshmukh . This is an open access article distributed under the Creative Commons Attribution License, which permits unrestricted use, distribution, and reproduction in any medium, provided the original work is properly cited.
View this paper at Google Scholar | DPI Digital Library
How to Cite this Paper
- IEEE Citation
- MLA Citation
- APA Citation
- BibTex Citation
- RIS Citation
IEEE Style Citation: Vilas V Pichad , Sachin N Deshmukh, “Role of Document Clustering For Forensic Analysis Investigation System,” International Journal of Computer Sciences and Engineering, Vol.3, Issue.3, pp.116-120, 2015.
MLA Style Citation: Vilas V Pichad , Sachin N Deshmukh "Role of Document Clustering For Forensic Analysis Investigation System." International Journal of Computer Sciences and Engineering 3.3 (2015): 116-120.
APA Style Citation: Vilas V Pichad , Sachin N Deshmukh, (2015). Role of Document Clustering For Forensic Analysis Investigation System. International Journal of Computer Sciences and Engineering, 3(3), 116-120.
BibTex Style Citation:
@article{Pichad_2015,
author = { Vilas V Pichad , Sachin N Deshmukh},
title = {Role of Document Clustering For Forensic Analysis Investigation System},
journal = {International Journal of Computer Sciences and Engineering},
issue_date = {3 2015},
volume = {3},
Issue = {3},
month = {3},
year = {2015},
issn = {2347-2693},
pages = {116-120},
url = {https://www.ijcseonline.org/full_paper_view.php?paper_id=433},
publisher = {IJCSE, Indore, INDIA},
}
RIS Style Citation:
TY - JOUR
UR - https://www.ijcseonline.org/full_paper_view.php?paper_id=433
TI - Role of Document Clustering For Forensic Analysis Investigation System
T2 - International Journal of Computer Sciences and Engineering
AU - Vilas V Pichad , Sachin N Deshmukh
PY - 2015
DA - 2015/03/31
PB - IJCSE, Indore, INDIA
SP - 116-120
IS - 3
VL - 3
SN - 2347-2693
ER -
![]() |
![]() |
![]() |
2460 | 2338 downloads | 2505 downloads |
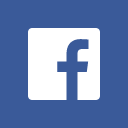
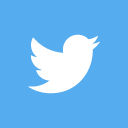
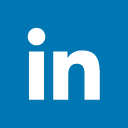
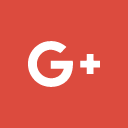
Abstract
Today’s digital word technologies information in computer world, there is extremely large increase in crime like money laundering, unauthorized access, ethical hacking, fraud detection in different domain etc. So, investigation of such cases deserve a lot more important, in this forensic investigation computer devices plays a major role. In Digital forensic analysis seized digital devices can provide precious information and evidences about facts and/or individuals on which the investigational activity is performed. In this paper we proposed document clustering algorithms to digital forensic analysis of computers seized devices in police investigations. In the Digital forensic analysis of investigation, used total six famous partition (k-means, k-medoids and CSPA) algorithm and hierarchical (Single Link, Complete Link and Average Link) document clustering algorithms are used. This is applied datasets for five real-world investigation cases conducted by the Brazilian Federal Police Department. Also two validity index are used to find out how many clusters are formed. This experiments show that the Average Link and Complete Link algorithms provide the best results for the application domain. This reviews different existing text clustering and Document clustering multithreading methods is used with computer forensic analysis.
Key-Words / Index Term
Digital Forensic Analysis, Document Clustering, Text Clustering,Multithreading
References
[1] Filipe daCruz, Nassif and Eduardo Raul Hruschka, “Document Clustering for Forensic Analysis: An Approach for Improving Computer Inspection” IEEE, Transactions on information forensics and security, VOL 8.No. 1, January 2013.
[2] A. K. Jain, R. C. Dupes, Algorithms for Clustering Data. Englewood Cliffs, NJ: Prentice-Hall, 1988.
[3] L. Kaufman, P. Rousseeuw, Finding Groups in Gata: An Introduction to Cluster Analysis. Hoboken, NJ: Wiley-Interscience, 1990.
[4] R. Xu, D. C.Wunsch, II, Clustering. Hoboken, NJ: Wiley/IEEE Press, 2009.
[5] A. Strehl and J. Ghosh, “Cluster ensembles: A knowledge reuse framework for combining multiple partitions,” J. Mach. Learning Res., vol. 3, pp. 583–617, 2002.
[6] B. K. L. Fei, J. H. P. Eloff, H. S. Venter, and. S. Oliver, “Exploring forensic data with self-organizing maps,” in Proc.IFIP Int. Conf. Digital Forensics, 2005, pp. 113–123.
[7] N. L. Beebe and J. G. Clark, “Digital forensic text string searching: Improving information retrieval effectiveness by thematically clustering search results,” Digital Investigation, Elsevier, vol. 4, no. 1, pp. 49–54, 2007.
[8] F. Iqbal, H. Binsalleeh, B. C. M. Fung, and M. Debbabi, “Mining write prints from anonymous e-mails for forensic investigation,” Digital Investigation, Elsevier, vol. 7, no. 1–2, pp. 56–64, 2010.
[9] R. Hadjidj, M. Debbabi, H. Lounis, F. Iqbal, A. Szporer, and D. Benredjem, “Towards an integrated e-mail forensic analysis framework, “Digital Investigation, Elsevier, vol. 5, no. 3–4, pp. 124–137, 2009.
[10] L. Liu, J. Kang, J. Yu, and Z. Wang, “A comparative study on unsupervised feature selection methods for text clustering,” in Proc. IEEE Int. Conf. Natural Language Processing and Knowledge Engineering, 2005, pp. 597–601.
[11] L. Vendramin, R. J. G. B. Campello, and E. R. Hruschka, “Relative clustering validity criteria: A comparative overview,” Statist. Anal. Data Mining, vol. 3, pp. 209–235, 2010.
[12] Jensen, J.H., Ellis, D., Christensen, M.G., Jensen, and S.H.: Evaluation distance measures between Gaussian mixture models of mfccs. Proc. Int. Conf. on Music Info. Retrieval ISMIR-07 Vienna, Austria pp. 107–108 (October, 2007)
[13] C. M. Bishop, Pattern Recognition and Machine Learning. New York: Springer-Verlag, 2006.