Artificial Neural Network Model for Prediction of Latent Heat Flux over Bay of Bengal
Kanchan. V. Shende1 , Vishal S. Shirsat2 , M. R. Ramesh Kumar3 , K.V. Kale4
Section:Research Paper, Product Type: Journal Paper
Volume-7 ,
Issue-5 , Page no. 901-905, May-2019
CrossRef-DOI: https://doi.org/10.26438/ijcse/v7i5.901905
Online published on May 31, 2019
Copyright © Kanchan. V. Shende, Vishal S. Shirsat, M. R. Ramesh Kumar, K.V. Kale . This is an open access article distributed under the Creative Commons Attribution License, which permits unrestricted use, distribution, and reproduction in any medium, provided the original work is properly cited.
View this paper at Google Scholar | DPI Digital Library
How to Cite this Paper
- IEEE Citation
- MLA Citation
- APA Citation
- BibTex Citation
- RIS Citation
IEEE Style Citation: Kanchan. V. Shende, Vishal S. Shirsat, M. R. Ramesh Kumar, K.V. Kale, “Artificial Neural Network Model for Prediction of Latent Heat Flux over Bay of Bengal,” International Journal of Computer Sciences and Engineering, Vol.7, Issue.5, pp.901-905, 2019.
MLA Style Citation: Kanchan. V. Shende, Vishal S. Shirsat, M. R. Ramesh Kumar, K.V. Kale "Artificial Neural Network Model for Prediction of Latent Heat Flux over Bay of Bengal." International Journal of Computer Sciences and Engineering 7.5 (2019): 901-905.
APA Style Citation: Kanchan. V. Shende, Vishal S. Shirsat, M. R. Ramesh Kumar, K.V. Kale, (2019). Artificial Neural Network Model for Prediction of Latent Heat Flux over Bay of Bengal. International Journal of Computer Sciences and Engineering, 7(5), 901-905.
BibTex Style Citation:
@article{Shende_2019,
author = {Kanchan. V. Shende, Vishal S. Shirsat, M. R. Ramesh Kumar, K.V. Kale},
title = {Artificial Neural Network Model for Prediction of Latent Heat Flux over Bay of Bengal},
journal = {International Journal of Computer Sciences and Engineering},
issue_date = {5 2019},
volume = {7},
Issue = {5},
month = {5},
year = {2019},
issn = {2347-2693},
pages = {901-905},
url = {https://www.ijcseonline.org/full_paper_view.php?paper_id=4335},
doi = {https://doi.org/10.26438/ijcse/v7i5.901905}
publisher = {IJCSE, Indore, INDIA},
}
RIS Style Citation:
TY - JOUR
DO = {https://doi.org/10.26438/ijcse/v7i5.901905}
UR - https://www.ijcseonline.org/full_paper_view.php?paper_id=4335
TI - Artificial Neural Network Model for Prediction of Latent Heat Flux over Bay of Bengal
T2 - International Journal of Computer Sciences and Engineering
AU - Kanchan. V. Shende, Vishal S. Shirsat, M. R. Ramesh Kumar, K.V. Kale
PY - 2019
DA - 2019/05/31
PB - IJCSE, Indore, INDIA
SP - 901-905
IS - 5
VL - 7
SN - 2347-2693
ER -
![]() |
![]() |
![]() |
343 | 218 downloads | 161 downloads |
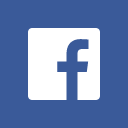
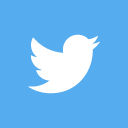
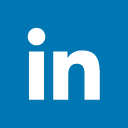
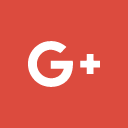
Abstract
Latent Heat Flux is the flux of heat from the Earth’s surface to the atmosphere that is associated with evaporation of water at the surface and subsequent condensation of water vapour in the troposphere. It is key component of global water and energy cycles. It also responsible for to maintain the salinity budget of the ocean surface. Prediction of Latent Heat Flux is essential for understanding ocean-atmospheric interaction process which helps to explore the climate change condition, global warming conditions and also monitor the atmospheric conditions. Ocean and atmosphere data is a non-linear type of data to extract the more information from non-linear data, Artificial Neural Network gives a better solution. Among the Indian Ocean only Bay of Bengal having a numerous significant features. Changes in a Bay of Bengal responsible for climate change condition, precipitation and cyclone formation etc., In present analysis used an ocean parameters such as Wind Speed at 10m above sea surface and Sea Surface Temperature for the prediction of Latent Heat Flux over the latitude 80°E: 100°E and longitude 0-25°N for Bay of Bengal from 2011 to 2015. To achieve the objective use a Feed-forward Neural Network model with Levenberg-Marquardt training algorithm. Performance of model is calculate using performance analysis parameters such as Correlation Coefficient and Room Mean Square Error. The result of Correlation Coefficient and Root Mean Square Error indicates that proposed neural network model gives a better prediction of Latent Heat Flux.
Key-Words / Index Term
Latent Heat Flux, Bay of Bengal, Sea Surface Temperature, Wind Speed, Artificial Neural Network
References
[1] S. Karmakar, S. Coubey, P. Mishra, “Appropriateness of Neural Networks in Climate Prediction and Interpolations: A Comprehensive Literature Review”, International Journal of Applied Information Systems, Vol.10, Issue.10, pp.33-54, 2016.
[2] A. S. Gandhi, S. D’Souza, N. B. Arjun, “Prediction of daily sea surface temperature using Artificial Neural Networks”, International Journal of Remote Sensing and Remote Sensing Letters, Vol.39, Issue.12, pp.1-25, 2018.
[3] C. Amrender Kumar, C. Chattopadhyay, A. K. Mishra, A. K. Jain, “Neural network based prediction models for evaporation” Mausam, Vol.67, Issue.2, pp.389-396, 2016.
[4] M. Narvekar, P. Fargose, “Daily Weather Forecasting using Artificial Neural Network” International Journal of Computer Applications, Vol.121, Issue.22, pp.9-13, 2015.
[5] A. Erdil, E. Arcaklioglu, “The prediction of meteorological variables using artificial neural network”, Neural Comput & Applic, Vol.22. pp. 1677-1683, 2013.
[6] A. J. Litta, S. M. Idicula, U. C. Mohanty, “Artificial Neural Network Model in Prediction of Metrological Parameters during Premonsoon Thunderstorms”, International Journal of Atmospheric Sciences, Vol.2013, pp.1-15, 2013.
[7] L. Bodri, V. Cermak, “Prediction of extreme precipitation using a neural network: application to summer flood occurrence in Moravia”, Advances in Engineering Software, Vol.31, Issue.5, pp.311-321, 2000.
[8] K. C. Luk, J. E. Ball, A. Sharma, “A study of optical model lag and spatial inputs to artificial neural network for rainfall forecasting”, Journal of Hydrology, Vol.227, pp.56-65, 2000.
[9] I. Maqsood, M. R. Khan, A. Abraham, “An ensemble of neural networks for weather forecasting”, Neural Computing and Applications, Vol.13, Issue.2, pp.112-122, 2004.
[10] S. Chaudhuri, S. Chattopadhyay, “Neuro-computing based short range prediction of some meteorological parameters during pre-monsoon season”, Soft Computing, Vol.9, Issue.5, pp.349-354, 2005.
[11] C. Cheng, K. Chau, Y. Sun, J. Lin, 2, “Long-term prediction of discharges in manwan reserviour using artificial neural network models”, Advances in Neural Networks, Vol.3498, pp.1040-1045, 2005.
[12] K. W. Chau, C. L. Wu, Y. S. Li, “Comparison of several flood forecasting models in Yangtze River”, Journal of Hydrologic Engineering, Vol.10, Issue.6, pp.485-491, 2005.
[13] N. Muttil, K. Chau, “Neural network and genetic programming for modelling coastal algal blooms” International Journal of Environment and pollution, Vol.28, Issue.3-4, pp.223-238, 2006.
[14] C. L. Wu, K. W. Chau, Y. S. Li, “Predicting monthly streamflow using data-driven models coupled with data-preprocessing techniques”, Water Resource Research Vol.45, Issue.8, pp.1-23, 2009.
[15] B. Praveen Kumar, J. Vialard, M. Lengaigne, V. S. N. Murty, M. J. McPhaden, “TropFlux: air-sea fluxes for the global tropical oceans-description and evaluation”, Climate Dynamics, Vol.38, pp.1521-1543, 2012.
[16] B. Praveen Kumar, J. Vialard, M. Lengaigne, V. S. N. Murty, M. J. McPhaden, M. F. Cronin, F. Pinsard, K. G. Reddy, “TropFlux wind stresses over the tropical oceans: evaluation and comparison with other products”, Climate Dynamics, Vol.40, pp. 2049-2071, 2013.