Detection With Firefly Algorithm (FA) Based Feature Selection Forautism Spectrum Disorder (ASD) and Machine Learning Classification
R. Rajeswari1 , R.S. Padma Priya2
Section:Research Paper, Product Type: Journal Paper
Volume-7 ,
Issue-5 , Page no. 992-998, May-2019
CrossRef-DOI: https://doi.org/10.26438/ijcse/v7i5.992998
Online published on May 31, 2019
Copyright © R. Rajeswari, R.S. Padma Priya . This is an open access article distributed under the Creative Commons Attribution License, which permits unrestricted use, distribution, and reproduction in any medium, provided the original work is properly cited.
View this paper at Google Scholar | DPI Digital Library
How to Cite this Paper
- IEEE Citation
- MLA Citation
- APA Citation
- BibTex Citation
- RIS Citation
IEEE Style Citation: R. Rajeswari, R.S. Padma Priya, “Detection With Firefly Algorithm (FA) Based Feature Selection Forautism Spectrum Disorder (ASD) and Machine Learning Classification,” International Journal of Computer Sciences and Engineering, Vol.7, Issue.5, pp.992-998, 2019.
MLA Style Citation: R. Rajeswari, R.S. Padma Priya "Detection With Firefly Algorithm (FA) Based Feature Selection Forautism Spectrum Disorder (ASD) and Machine Learning Classification." International Journal of Computer Sciences and Engineering 7.5 (2019): 992-998.
APA Style Citation: R. Rajeswari, R.S. Padma Priya, (2019). Detection With Firefly Algorithm (FA) Based Feature Selection Forautism Spectrum Disorder (ASD) and Machine Learning Classification. International Journal of Computer Sciences and Engineering, 7(5), 992-998.
BibTex Style Citation:
@article{Rajeswari_2019,
author = {R. Rajeswari, R.S. Padma Priya},
title = {Detection With Firefly Algorithm (FA) Based Feature Selection Forautism Spectrum Disorder (ASD) and Machine Learning Classification},
journal = {International Journal of Computer Sciences and Engineering},
issue_date = {5 2019},
volume = {7},
Issue = {5},
month = {5},
year = {2019},
issn = {2347-2693},
pages = {992-998},
url = {https://www.ijcseonline.org/full_paper_view.php?paper_id=4351},
doi = {https://doi.org/10.26438/ijcse/v7i5.992998}
publisher = {IJCSE, Indore, INDIA},
}
RIS Style Citation:
TY - JOUR
DO = {https://doi.org/10.26438/ijcse/v7i5.992998}
UR - https://www.ijcseonline.org/full_paper_view.php?paper_id=4351
TI - Detection With Firefly Algorithm (FA) Based Feature Selection Forautism Spectrum Disorder (ASD) and Machine Learning Classification
T2 - International Journal of Computer Sciences and Engineering
AU - R. Rajeswari, R.S. Padma Priya
PY - 2019
DA - 2019/05/31
PB - IJCSE, Indore, INDIA
SP - 992-998
IS - 5
VL - 7
SN - 2347-2693
ER -
![]() |
![]() |
![]() |
283 | 172 downloads | 113 downloads |
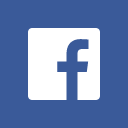
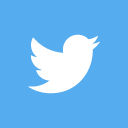
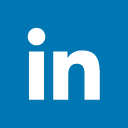
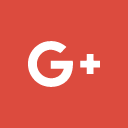
Abstract
Autism Spectrum Disorders (ASDs) are difficult to classify, however it needs with the purpose of physicians have correct training and knowledge. At the instant on the other hand ASD is classified much afterward than is essentially potential. Early on identification of ASD increases the overall mind health of the child. Consequently, in the direction of advantage autism patients through increasing their right to use toward treatments such as early involvement, plan toward begin a robust data mining methods used for autism classification by using detection and feature selection algorithms depending on information from ASD patients. The basic aim of this work is toward choose best optimal features toward conquer the learning problem and to go faster the knowledge ability of autistic children. In order to carryout optimal feature selection process, Firefly Algorithm (FA) is introduced which chooses the features from the ASD screening procedure. In this work, an accurate FA method is introduced for identifying the most important and choosing a best ASD feature subset. The feature selection algorithm is performed depending on the wrapper method, i.e. the FA and the Support Vector Machine (SVM) is developed for ASD classification correspondingly. The proposed SVM classifier might considerably shorten and abbreviate the step of ASD analysis. The results demonstrated that the proposed SVM with FA classifier has provided an improved classification results depending on the chosen features. The experimental results of that FA based feature selection performing better when compared to other algorithms.
Key-Words / Index Term
Autism Spectrum Disorders (ASDs), Firefly Algorithm (FA), feature selection, Support Vector Machine (SVM), Medical forms, Classification
References
[1] Autism and Developmental Disabilities Monitoring Network Surveillance Year 2010 Principal Investigators, 2014. Prevalence of autism spectrum disorder among children aged 8 years—autism and developmental disabilities monitoring network, 11 sites, United States, 2010. Morbidity and Mortality Weekly Report: Surveillance Summaries, Vol.63, Issue. 2, pp.1-21, 2010.
[2] Iossifov, I, O’roak, BJ, Sanders, SJ, Ronemus, M, Krumm, N, Levy, D, Stessman, HA, Witherspoon, KT, Vives, L Patterson, KE and Smith, JD, “The contribution of de novo coding mutations to autism spectrum disorder”, Nature, Vol.515, Issue. 7526, pp.216–221,2014.
[3] De Rubeis, S, He, X, Goldberg, AP, Poultney, CS, Samocha, K, Cicek, AE, Kou, Y, Liu, L, Fromer, M, Walker, S and Singh, T, “Synaptic, transcriptional and chromatin genes disrupted in autism”, Nature, Vol.515, Issue. 7526, pp. 209–215,2014.
[4] Myers, SM, and Johnson CP, “American Academy of Pediatrics Council on Children With Disabilities”, Management of children with autism spectrum disorders, Pediatrics. Vol.120, Issue.5, pp.1162–1182, 2007.
[5] Daniels, AM and Mandell DS, “Explaining differences in age at autism spectrum disorder diagnosis: a critical review”, Autism, Vol. 18, Issue. 5, pp.583–597, 2014.
[6] Developmental Disabilities Monitoring Network Surveillance Year 2010 Principal Investigators, Centers for Disease Control and Prevention (CDC). Prevalence of autism spectrum disorder among children aged 8 years—autism and developmental disabilities monitoring network, 11 sites, United States, 2010. MMWR Surveill Summ, Vol.63, Issue. 2, pp.1–21, 2014.
[7] Shattuck, PT and Grosse SD, “Issues related to the diagnosis and treatment of autism spectrum disorders”, Ment Retard Dev Disabil Res Rev, Vol.13, Issue.2, pp.129–135, 2007.
[8] Wallace, KS and Rogers SJ, “Intervening in infancy: implications for autism spectrum disorders”, J Child Psychol Psychiatry, Vol. 51, Issue.12, pp.1300–1320, 2010.
[9] Hsu, HH, Hsieh, CW and Lu, MD, “Hybrid feature selection by combining filters and wrappers”, Expert Systems with Applications, Vol.38, Issue.7, pp.8144-8150, 2011.
[10] Gutlein, M, Frank, E, Hall, M and Karwath, A, “Large-scale attribute selection using wrappers”, IEEE Symposium on Computational Intelligence and Data Mining, 2009 (CIDM`09), pp. 332-339, 2009.
[11] Saeys, Y, Inza, I and Larrañaga, P, “A review of feature selection techniques in bioinformatics”, bioinformatics, Vol.23, Issue.19, pp.2507-2517, 2007.
[12] Chandrashekar, G and Sahin, F, “A survey on feature selection methods”, Computers & Electrical Engineering, Vol.40, Issue. 1, pp.16-28, 2014.
[13] Akyol, K , and Karaci, A, “A Study on Autistic Spectrum Disorder for Children Based on Feature Selection and Fuzzy Rule”, ICELIS, pp. 804-807, year.
[14] Alzubi, R, Ramzan, N and Alzoubi, H, “Hybrid feature selection method for autism spectrum disorder SNPs”, In IEEE Conference on Computational Intelligence in Bioinformatics and Computational Biology (CIBCB) ,pp. 1-7, 2017.
[15] Heinsfeld, AS, Franco, AR, Craddock, RC, Buchweitz, A and Meneguzzi, F, “Identification of autism spectrum disorder using deep learning and the ABIDE dataset”, NeuroImage: Clinical, Vol.17, pp.16-23, 2018.
[16] Kosmicki, JA, Sochat, V, Duda, M and Wall, DP, “Searching for a minimal set of behaviors for autism detection through feature selection-based machine learning”, Translational psychiatry, Vol.5, Issue. 2, pp.e514, 2015.
[17] Bi, XA, Wang, Y, Shu, Q, Sun, Q and Xu, Q, “Classification of autism spectrum disorder using random support vector machine cluster”, Frontiers in genetics, Vol.9, Issue. 18, pp.1-10, 2018.
[18] Cheplygina, V, Tax, DM, Loog, M and Feragen, A, “Network-guided group feature selection for classification of autism spectrum disorder”, In International Workshop on Machine Learning in Medical Imaging, pp. 190-197, 2014.
[19] Thabtah, F, “Autism Spectrum Disorder Screening: Machine Learning Adaptation and DSM-5 Fulfillment”, In Proceedings of the 1st International Conference on Medical and Health Informatics 2017 , pp. 1-6, 2017.
[20] X.-S. Yang, “Nature-Inspired Metaheuristic Algorithms”, Luniver Press, 2008.
[21] Zeng, ZQ, Yu, HB, Xu, HR, Xie, YQ and Gao, J, “Fast training support vector machines using parallel sequential minimal optimization”, In 2008 3rd international conference on intelligent system and knowledge engineering , Vol. 1, pp. 997-1001, 2008.
[22] Chang, CC and Lin, CJ, “LIBSVM: a library for support vector machines”, ACM transactions on intelligent systems and technology (TIST), Vol. 2, Issue. 3, pp.27, 2011.
[23] Thabtah, F, “Machine learning in autistic spectrum disorder behavioral research: A review and ways forward”, Informatics for Health and Social Care, pp.1-20, 2018.