Spatio-Temporal Neural Network Approach for Location Prediction: State-of-the-Art, Challenges and Future Directions
N. Venkata Subba Reddy1 , D. S. R. Murthy2
Section:Review Paper, Product Type: Journal Paper
Volume-7 ,
Issue-5 , Page no. 1057-1067, May-2019
CrossRef-DOI: https://doi.org/10.26438/ijcse/v7i5.10571067
Online published on May 31, 2019
Copyright © N. Venkata Subba Reddy, D. S. R. Murthy . This is an open access article distributed under the Creative Commons Attribution License, which permits unrestricted use, distribution, and reproduction in any medium, provided the original work is properly cited.
View this paper at Google Scholar | DPI Digital Library
How to Cite this Paper
- IEEE Citation
- MLA Citation
- APA Citation
- BibTex Citation
- RIS Citation
IEEE Style Citation: N. Venkata Subba Reddy, D. S. R. Murthy, “Spatio-Temporal Neural Network Approach for Location Prediction: State-of-the-Art, Challenges and Future Directions,” International Journal of Computer Sciences and Engineering, Vol.7, Issue.5, pp.1057-1067, 2019.
MLA Style Citation: N. Venkata Subba Reddy, D. S. R. Murthy "Spatio-Temporal Neural Network Approach for Location Prediction: State-of-the-Art, Challenges and Future Directions." International Journal of Computer Sciences and Engineering 7.5 (2019): 1057-1067.
APA Style Citation: N. Venkata Subba Reddy, D. S. R. Murthy, (2019). Spatio-Temporal Neural Network Approach for Location Prediction: State-of-the-Art, Challenges and Future Directions. International Journal of Computer Sciences and Engineering, 7(5), 1057-1067.
BibTex Style Citation:
@article{Reddy_2019,
author = {N. Venkata Subba Reddy, D. S. R. Murthy},
title = {Spatio-Temporal Neural Network Approach for Location Prediction: State-of-the-Art, Challenges and Future Directions},
journal = {International Journal of Computer Sciences and Engineering},
issue_date = {5 2019},
volume = {7},
Issue = {5},
month = {5},
year = {2019},
issn = {2347-2693},
pages = {1057-1067},
url = {https://www.ijcseonline.org/full_paper_view.php?paper_id=4362},
doi = {https://doi.org/10.26438/ijcse/v7i5.10571067}
publisher = {IJCSE, Indore, INDIA},
}
RIS Style Citation:
TY - JOUR
DO = {https://doi.org/10.26438/ijcse/v7i5.10571067}
UR - https://www.ijcseonline.org/full_paper_view.php?paper_id=4362
TI - Spatio-Temporal Neural Network Approach for Location Prediction: State-of-the-Art, Challenges and Future Directions
T2 - International Journal of Computer Sciences and Engineering
AU - N. Venkata Subba Reddy, D. S. R. Murthy
PY - 2019
DA - 2019/05/31
PB - IJCSE, Indore, INDIA
SP - 1057-1067
IS - 5
VL - 7
SN - 2347-2693
ER -
![]() |
![]() |
![]() |
192 | 227 downloads | 116 downloads |
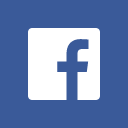
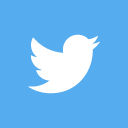
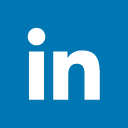
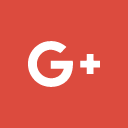
Abstract
Nowadays, the huge set of spatio-temporal data (STD)are increasingly collected and utilized in different domains that include social sciences, epidemiology, mobile health, climate science, neuroscience, transportation and Earth sciences. Compared to relational data, the STD is different for that the researcher developed computational techniques in the data mining community. The process of extracting implicit knowledge and unknown information, structures in spatio-temporal (ST)dataset , patterns that are not explicitly stored and spatio-temporal relationships is called Spatio-temporal data mining (STDM). As one of information mining procedures, data prediction approach is widely utilized toward forecast the unknown future on the basis of hidden patterns in the past and current data. To obtain ST forecasting, few of them developed analysis tools like spatial information are prolonged to temporal dimension (TD)as well as the time series extended to the spatial dimension (SD) or joined linearly as a ST combination. But, such kind of linear combination of TD and SD is a generalization of difficult ST relations which is present in complex geographical occurrences. The present study, reviews the traditional STDM approach, tools, pattern mining approach and data analysis. On the basis of data mining issues, this literature has been classified into four main categories: trajectory mining approach, clustering, pattern mining, predictive learning and location prediction. We discourse the different forms of STDM issues in each of these groups.
Key-Words / Index Term
Spatio-Temporal Data, Trajectory Clustering and Mining Approach, Location Prediction, Trajectory Pattern Analysis
References
[1] De Almeida, V.T. & Güting, R.H. (2005). Indexing the Trajectories of Moving Objects in Networks*. Geoinformatica. [Online]. 9 (1). Pp. 33–60. Available from: http://link.springer.com/10.1007/s10707-004-5621-7.
[2] Altche, F. & de La Fortelle, A. (2017). An LSTM network for highway trajectory prediction. In: 2017 IEEE 20th International Conference on Intelligent Transportation Systems (ITSC). [Online]. October 2017, IEEE, pp. 353–359. Available from: http://ieeexplore.ieee.org/document/8317913/.
[3] Aryal, A.M. & Sujing Wang (2017). Discovery of patterns in spatio-temporal data using clustering techniques. In: 2017 2nd International Conference on Image, Vision and Computing (ICIVC). [Online]. June 2017, IEEE, pp. 990–995. Available from: http://ieeexplore.ieee.org/document/7984703/.
[4] Bahadori, M.T., Yu, Q. (Rose) & Liu, Y. (2014). Fast Multivariate Spatio-temporal Analysis via Low Rank Tensor Learning. Nips. [Online]. Pp. 1–9. Available from: https://pdfs.semanticscholar.org/82e5/c9a1ccb59b7ca6952d1f2741881c3a15ab57.pdf.
[5] Bao, J., Zheng, Y. & Mokbel, M.F. (2013). Location-based and preference-aware recommendation using sparse geo-social networking data. [Online]. Pp. 199. Available from: https://www.microsoft.com/en-us/research/wp-content/uploads/2016/02/locationrecommendation.pdf.
[6] Bengio, Y. (2010). Neural net language models. Scholarpedia.
[7] Bock, J., Beemelmanns, T., Klösges, M. & Kotte, J. (2017). Self-learning Trajectory Prediction with Recurrent Neural Networks at Intelligent Intersections. [Online]. (Vehits). Pp. 346–351. Available from: https://www.scitepress.org/papers/2017/63740/63740.pdf.
[8] Bogorny, V., Kuijpers, B. & Alvares, L.O. (2009). ST‐DMQL: A Semantic Trajectory Data Mining Query Language. International Journal of Geographical Information Science. [Online]. 23 (10). Pp. 1245–1276. Available from: http://www.tandfonline.com/doi/abs/10.1080/13658810802231449.
[9] Bolstad, P. (2005). Gis Fundamentals: A First Text on Geographic Information Systems. [Online]. Eider Press. Available from: https://books.google.co.in/books?Id=cfheaaaayaaj.
[10] Cao, H., Mamoulis, N. & Cheung, D.W. (2007). Discovery of Periodic Patterns in Spatiotemporal Sequences. IEEE Transactions on Knowledge and Data Engineering. 19 (4). Pp. 453–467.
[11] Catlett, C., Cesario, E., Talia, D. & Vinci, A. (2018). A Data-Driven Approach for Spatio-Temporal Crime Predictions in Smart Cities. In: 2018 IEEE International Conference on Smart Computing (SMARTCOMP). [Online]. June 2018, IEEE, pp. 17–24. Available from: https://ieeexplore.ieee.org/document/8421327/.
[12] Chen, L., Lv, M., Ye, Q., Chen, G. & Woodward, J. (2011). A personal route prediction system based on trajectory data mining. Information Sciences. [Online]. 181 (7). Pp. 1264–1284. Available from: https://linkinghub.elsevier.com/retrieve/pii/S0020025510005888.
[13] Cheng, T. & Adepeju, M. (2014). Modifiable temporal unit problem (MTUP) and its effect on space-time cluster detection. Plos ONE.
[14] Elhorst, J.P. (2003). Specification and estimation of spatial panel data models. International Regional Science Review.
[15] Ester, M., Kriegel, H.-P., Jorg, S. & Xu, X. (1996). A Density-Based Clustering Algorithms for Discovering Clusters. Kdd. [Online]. 96 (34). Pp. 226–231. Available from: https://www.aaai.org/Papers/KDD/1996/KDD96-037.pdf.
[16] Ganguly, A.R. & Steinhaeuser, K. (2008). Data Mining for Climate Change and Impacts. In: 2008 IEEE International Conference on Data Mining Workshops. [Online]. December 2008, IEEE, pp. 385–394. Available from: http://ieeexplore.ieee.org/document/4733959/.
[17] Giannotti, F., Nanni, M. & Pedreschi, D. (2006). Efficient Mining of Temporally Annotated Sequences. In: Proceedings of the 2006 SIAM International Conference on Data Mining. [Online]. 20 April 2006, Philadelphia, PA: Society for Industrial and Applied Mathematics, pp. 348–359. Available from: https://epubs.siam.org/doi/10.1137/1.9781611972764.31.
[18] Ignaccolo, R., Mateu, J. & Giraldo, R. (2014). Kriging with external drift for functional data for air quality monitoring. Stochastic Environmental Research and Risk Assessment.
[19] Janaki, V.R.; P.V.K.; V. (2016). Mining of Outlier Temporal Patterns. In: 2016 International Conference on Engineering & MIS (ICEMIS). 2016, Agadir, Morocco: IEEE Comput. Soc. Press.
[20] Jeung, H., Liu, Q., Shen, H.T. & Zhou, X. (2008). A Hybrid Prediction Model for Moving Objects. In: 2008 IEEE 24th International Conference on Data Engineering. [Online]. April 2008, IEEE, pp. 70–79. Available from: http://ieeexplore.ieee.org/document/4497415/.
[21] Jeung, H., Shen, H.T. & Zhou, X. (2007). Mining Trajectory Patterns Using Hidden Markov Models. In: Data Warehousing and Knowledge Discovery. [Online]. Berlin, Heidelberg: Springer Berlin Heidelberg, pp. 470–480. Available from: http://link.springer.com/10.1007/978-3-540-74553-2_44.
[22] Kurashima, T., Iwata, T., Irie, G. & Fujimura, K. (2010). Travel route recommendation using geotags in photo sharing sites. Pp. 579.
[23] Lathia, N., Hailes, S. & Capra, L. (2009). Temporal collaborative filtering with adaptive neighbourhoods. [Online]. University College London. Available from: http://www0.cs.ucl.ac.uk/staff/n.lathia/papers/lathia_sigir09.pdf.
[24] Lei, P.-R., Li, S.-C. & Peng, W.-C. (2012). QS-STT: quadsection clustering and spatial-temporal trajectory model for location prediction. [Online]. Available from: https://www.researchgate.net/publication/232703396_QS-STT_quadsection_clustering_and_spatial-temporal_trajectory_model_for_location_prediction.
[25] Lei, P.R., Shen, T.J., Peng, W.C. & Su, I.J. (2011). Exploring spatial-temporal trajectory model for location prediction. In: Proceedings - IEEE International Conference on Mobile Data Management. 2011.
[26] Li, J., Qin, Q., Han, J., Tang, L.-A. & Lei, K.H. (2015). Mining Trajectory Data and Geotagged Data in Social Media for Road Map Inference. Transactions in GIS. [Online]. 19 (1). Pp. 1–18. Available from: http://doi.wiley.com/10.1111/tgis.12072.
[27] Li, X., Yu, C., Ju, L., Qin, J., Zhang, Y., Dou, L. & Yuqing, S. (2018). Position prediction system based on spatio-temporal regularity of object mobility. Information Systems. [Online]. 75. Pp. 43–55. Available from: https://linkinghub.elsevier.com/retrieve/pii/S0306437917304726.
[28] Li, Z., Chen, J. & Baltsavias, E. (2008). Advances in Photogrammetry, Remote Sensing and Spatial Information Sciences: 2008 ISPRS Congress Book. ISPRS Book Series. [Online]. CRC Press. Available from:https://books.google.co.in/books?Id=-fqm3buoejcc.
[29] Li, Z., Ding, B., Han, J., Kays, R. & Nye, P. (2010).Mining periodic behaviors for moving objects. [Online]. University of Illinois at Urbana-Champaign. Available https://faculty.ist.psu.edu/jessieli/Publications/KDD10-zli-periodmovobj.pdf.
[30] Li, Z., Han, J., Ding, B. & Kays, R. (2012). Mining periodic behaviors of object movements for animal and biological sustainability studies. Data Mining and Knowledge Discovery. [Online]. 24 (2). Pp. 355–386. Available from: http://link.springer.com/10.1007/s10618-011-0227-9.
[31] Liu, Q., Wu, S., Wang, L. & Tan, T. (2017). Predicting the Next Location : A Recurrent Model with Spatial and Temporal Contexts. Aaai. [Online]. Pp. 194–200. Available from: https://www.aaai.org/ocs/index.php/AAAI/AAAI16/paper/view/11900/11583