Applications of Machine Learning Techniques on Prediction of Children’s various health problems: A Survey
A. Meharaj Begum1
Section:Survey Paper, Product Type: Journal Paper
Volume-7 ,
Issue-5 , Page no. 1166-1176, May-2019
CrossRef-DOI: https://doi.org/10.26438/ijcse/v7i5.11661176
Online published on May 31, 2019
Copyright © A. Meharaj Begum . This is an open access article distributed under the Creative Commons Attribution License, which permits unrestricted use, distribution, and reproduction in any medium, provided the original work is properly cited.
View this paper at Google Scholar | DPI Digital Library
How to Cite this Paper
- IEEE Citation
- MLA Citation
- APA Citation
- BibTex Citation
- RIS Citation
IEEE Style Citation: A. Meharaj Begum, “Applications of Machine Learning Techniques on Prediction of Children’s various health problems: A Survey,” International Journal of Computer Sciences and Engineering, Vol.7, Issue.5, pp.1166-1176, 2019.
MLA Style Citation: A. Meharaj Begum "Applications of Machine Learning Techniques on Prediction of Children’s various health problems: A Survey." International Journal of Computer Sciences and Engineering 7.5 (2019): 1166-1176.
APA Style Citation: A. Meharaj Begum, (2019). Applications of Machine Learning Techniques on Prediction of Children’s various health problems: A Survey. International Journal of Computer Sciences and Engineering, 7(5), 1166-1176.
BibTex Style Citation:
@article{Begum_2019,
author = {A. Meharaj Begum},
title = {Applications of Machine Learning Techniques on Prediction of Children’s various health problems: A Survey},
journal = {International Journal of Computer Sciences and Engineering},
issue_date = {5 2019},
volume = {7},
Issue = {5},
month = {5},
year = {2019},
issn = {2347-2693},
pages = {1166-1176},
url = {https://www.ijcseonline.org/full_paper_view.php?paper_id=4380},
doi = {https://doi.org/10.26438/ijcse/v7i5.11661176}
publisher = {IJCSE, Indore, INDIA},
}
RIS Style Citation:
TY - JOUR
DO = {https://doi.org/10.26438/ijcse/v7i5.11661176}
UR - https://www.ijcseonline.org/full_paper_view.php?paper_id=4380
TI - Applications of Machine Learning Techniques on Prediction of Children’s various health problems: A Survey
T2 - International Journal of Computer Sciences and Engineering
AU - A. Meharaj Begum
PY - 2019
DA - 2019/05/31
PB - IJCSE, Indore, INDIA
SP - 1166-1176
IS - 5
VL - 7
SN - 2347-2693
ER -
![]() |
![]() |
![]() |
267 | 264 downloads | 101 downloads |
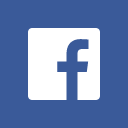
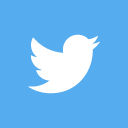
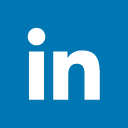
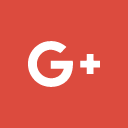
Abstract
Early diagnosis of children’s health problems helps the professionals to treat it at an earlier stage and improves their quality of life. The life skills of the young minds are the best investment to build the healthy society of the Country. Researchers across various countries are interested to predict health problems related to children with respect to parenting style, hereditary health issues, food habits and physical activities. Machine learning plays a vital role in analyzing and predicting the hidden facts in the data we collected. The main objective of this study is to present an overview of many machine learning techniques such as Support Vector Machines, Naive Bayes classifier, K-Nearest Neighbor, Decision Tree, K-means algorithm and perform a comparative analysis of their accuracy and help the researchers to choose best algorithm on prediction of Children’s various health problems such as Early Childhood Obesity, Anxiety Disorders, Attention Deficit Hyperactive Disorder, Mental Health Problems, Child Post Traumatic Stress, Autism Spectrum Disorder and Insulin Resistance in Children. This survey paper can lead to develop innovative and efficient algorithms on prediction of children’s health problems to improve their quality of life in a better way.
Key-Words / Index Term
Machine Learning Algorithms, Prediction, Children’s Health Problems
References
[1]. Dugan, T. M., Mukhopadhyay, S., Carroll, A., & Downs, S. (2015). Machine learning techniques for prediction of early childhood obesity. Applied clinical informatics, 6(03), 506-520.
[2]. Theodore, K., Ioannis, L., Panagiotis, P., & Emmanouil, P. (2018). Reviewing machine learning techniques for predicting anxiety disorders.
[3]. Shah, A. (2015). Predicting ‘Attention Deficit Hyperactive Disorder ‘using large scale child data set.
[4]. Sumathi, M. R., & Poorna, B. (2016). Prediction of mental health problems among children using machine learning techniques. International Journal of Advanced Computer Science and Applications, 7(1), 552-557.
[5]. Usta, M. B., Karabekiroglu, K., Sahin, B., Aydin, M., Bozkurt, A., Karaosman, T., ... & Sahin, İ. (2018). Use of machine learning methods in prediction of short-term outcome in autism spectrum disorders. Psychiatry and Clinical Psychopharmacology, 1-6.
[6]. Alarifi, H. S., & Young, G. S. (2018). Using Multiple Machine Learning Algorithms to Predict Autism in Children. In Proceedings on the International Conference on Artificial Intelligence (ICAI) (pp. 464-467). The Steering Committee of the World Congress in Computer Science, Computer Engineering and Applied Computing (World Comp).
[7]. Saxe, G. N., Ma, S., Ren, J., & Aliferis, C. (2017). Machine learning methods to predict child posttraumatic stress: a proof of concept study. BMC psychiatry, 17(1), 223.
[8]. Hall, A. J., Hussain, A., & Shaikh, M. G. (2016, November). Predicting insulin resistance in children using a machine-learning-based clinical decision support system. In International Conference on Brain Inspired Cognitive Systems (pp. 274-283). Springer, Cham.
[9]. Caicedo-Torres, W., Paternina, Á., & Pinzón, H. (2016, November). Machine learning models for early dengue severity prediction. In Ibero-American Conference on Artificial Intelligence (pp. 247-258). Springer, Cham.
[10]. Islam, M. R., Kabir, M. A., Ahmed, A., Kamal, A. R. M., Wang, H., & Ulhaq, A. (2018). Depression detection from social network data using machine learning techniques. Health information science and systems, 6(1), 8.
[11]. Yildirim, P., Majnarić, L., Ekmekci, O. I., & Holzinger, A. (2013, September). On the prediction of clusters for adverse reactions and allergies on antibiotics for children to improve biomedical decision making. In International Conference on Availability, Reliability, and Security (pp. 431-445). Springer, Berlin, Heidelberg.
[12]. Oliveira, A. L. I., Baldisserotto, C., & Baldisserotto, J. (2005, November). A comparative study on machine learning techniques for prediction of success of dental implants. In Mexican International Conference on Artificial Intelligence (pp. 939-948). Springer, Berlin, Heidelberg.
[13]. Dwivedi, A. K. (2018). Performance evaluation of different machine learning techniques for prediction of heart disease. Neural Computing and Applications, 1-9.
[14]. Hassan, M. R., Al-Insaif, S., Hossain, M. I., & Kamruzzaman, J. A machine learning approach for prediction of pregnancy outcome following IVF treatment. Neural Computing and Applications, 1-15.
[15]. Jaafar, J., Atwell, E., Johnson, O., Clamp, S., & Ahmad, W. A. W. (2013). Evaluation of Machine Learning Techniques in Predicting Acute Coronary Syndrome Outcome. In Research and Development in Intelligent Systems XXX (pp. 321-333). Springer, Cham.
[16]. Dyne, M., Tsatsoulis, C., & Thorp, J. (1994, September). Using inductive machine learning, expert systems and case based reasoning to predict preterm delivery in pregnant women. In Database and Expert Systems Applications.
[17]. Husain, A., & Khan, M. H. (2018, April). Early Diabetes Prediction Using Voting Based Ensemble Learning. In International Conference on Advances in Computing and Data Sciences (pp. 95-103). Springer, Singapore.
[18]. Belciug, S. (2016). Machine learning solutions in computer-aided medical diagnosis. In Machine Learning for Health Informatics (pp. 289-302). Springer, Cham.
[19]. Fernandez-Granero, M. A., Sanchez-Morillo, D., Lopez-Gordo, M. A., & Leon, A. (2015, June). A machine learning approach to prediction of exacerbations of chronic obstructive pulmonary disease. In International Work-Conference on the Interplay Between Natural and Artificial Computation (pp. 305-311). Springer, Cham.
[20]. Martínez-Velasco, A., Zenteno, J. C., Martínez-Villaseñor, L., Miralles-Pechúan, L., Pérez-Ortiz, A., & Estrada-Mena, F. J. (2016). Machine learning method to establish the connection between age related macular degeneration and some genetic variations. In Ubiquitous Computing and Ambient Intelligence (pp. 28-39). Springer, Cham.
[21]. Shankle, W. R., Mani, S., Pazzani, M. J., & Smyth, P. (1997). Dementia screening with machine learning methods. In Intelligent Data Analysis in Medicine and Pharmacology (pp. 149-165). Springer, Boston, MA.
[22]. S. JabeenBegum., B. Swaathi April (2019). A Survey for identifying Parkinson’s disease by Binary Bat Algorithm in International Journal of Scientific Research in Computer Science and Engineering Vol.7, Issue.2, pp.17-23,