Secured MapReduce Based K-Means Clustering In Big Data Framework
D. Saidulu1 , V. Devasekhar2 , V. Swathi3
Section:Research Paper, Product Type: Journal Paper
Volume-7 ,
Issue-5 , Page no. 1427-1430, May-2019
CrossRef-DOI: https://doi.org/10.26438/ijcse/v7i5.14271430
Online published on May 31, 2019
Copyright © D. Saidulu, V. Devasekhar, V. Swathi . This is an open access article distributed under the Creative Commons Attribution License, which permits unrestricted use, distribution, and reproduction in any medium, provided the original work is properly cited.
View this paper at Google Scholar | DPI Digital Library
How to Cite this Paper
- IEEE Citation
- MLA Citation
- APA Citation
- BibTex Citation
- RIS Citation
IEEE Style Citation: D. Saidulu, V. Devasekhar, V. Swathi, “Secured MapReduce Based K-Means Clustering In Big Data Framework,” International Journal of Computer Sciences and Engineering, Vol.7, Issue.5, pp.1427-1430, 2019.
MLA Style Citation: D. Saidulu, V. Devasekhar, V. Swathi "Secured MapReduce Based K-Means Clustering In Big Data Framework." International Journal of Computer Sciences and Engineering 7.5 (2019): 1427-1430.
APA Style Citation: D. Saidulu, V. Devasekhar, V. Swathi, (2019). Secured MapReduce Based K-Means Clustering In Big Data Framework. International Journal of Computer Sciences and Engineering, 7(5), 1427-1430.
BibTex Style Citation:
@article{Saidulu_2019,
author = {D. Saidulu, V. Devasekhar, V. Swathi},
title = {Secured MapReduce Based K-Means Clustering In Big Data Framework},
journal = {International Journal of Computer Sciences and Engineering},
issue_date = {5 2019},
volume = {7},
Issue = {5},
month = {5},
year = {2019},
issn = {2347-2693},
pages = {1427-1430},
url = {https://www.ijcseonline.org/full_paper_view.php?paper_id=4424},
doi = {https://doi.org/10.26438/ijcse/v7i5.14271430}
publisher = {IJCSE, Indore, INDIA},
}
RIS Style Citation:
TY - JOUR
DO = {https://doi.org/10.26438/ijcse/v7i5.14271430}
UR - https://www.ijcseonline.org/full_paper_view.php?paper_id=4424
TI - Secured MapReduce Based K-Means Clustering In Big Data Framework
T2 - International Journal of Computer Sciences and Engineering
AU - D. Saidulu, V. Devasekhar, V. Swathi
PY - 2019
DA - 2019/05/31
PB - IJCSE, Indore, INDIA
SP - 1427-1430
IS - 5
VL - 7
SN - 2347-2693
ER -
![]() |
![]() |
![]() |
237 | 220 downloads | 87 downloads |
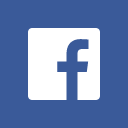
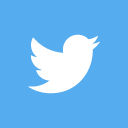
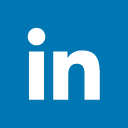
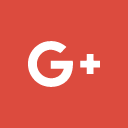
Abstract
Clustering is a significant task of research in data mining and analysis of statistics, which is initiate in many areas, including health care, social networking, image analysis, object recognition, etc. volume, quantity and speed. To effectively manage large-scale data sets and clusters, public cloud infrastructure plays an important role in both performance and economy. However, the use of public cloud services inevitably involves confidentiality issues. Indeed, not only a large scale of data mining applications but also deals with sensitive data such as personal healthcare information, location data, financial data, etc. In this paper we proposed Novel Secured MapReduce Based K-Means Clustering in Big Data Framework. This scheme achieves clustering speed and accuracy that are comparable to the K-means clustering without privacy protection. Furthermore we design securely integrated MapReduce framework and make it extremely suitable for parallelized processing in cloud computing environment.
Key-Words / Index Term
K-means clustering, Data encryption, Privacy-preserving, MapReduce
References
[1] J. Vaidya and C. Clifton, “Privacy-preserving k-means clustering over vertically partitioned data,” in ACM SIGKDD, 2003, pp. 206–215.
[2] C. Su, J. Zhou, F. Bao, T. Takagi, and K. Sakurai, “Two-party privacypreserving agglomerative document clustering,” in ISPEC. SpringerVerlag, 2007, pp. 193 – 208.
[3] G. Jagannathan and R. Wright, “Privacy-preserving distributed k-means clustering over arbitrarily partitioned data,” in ACM SIGKDD, 2005, pp. 593–599.
[4] P. Bunn and R. Ostrovsky, “Secure two-party k-means clustering,” in ACM CCS, 2007, pp. 486–497.
[5] C. Gentry, “Fully homomorphic encryption using ideal lattices,” in ACM STOC, 2009, pp. 169–178.
[6] C. Gentry and S. Halevi, “Implementing gentry’s
fully-homomorphicencryptionscheme,”in EUROCRYPT. Springer, 2011, pp. 129–148.
[7] R. Agrawal and R. Srikant, “Privacy preserving data mining,” in ACM SIGMOD, vol. 29, 2000, pp. 439–450.
[8] Y. Lindell and B. Pinkas, “Privacy preserving data mining,” in Journal of Cryptology, vol. 15, 2002, pp. 177 – 206.