A Novel Automated CNN Based Lung Cancer Prediction Technique (CNNLCPT) for CT scan images
M. Muthuraman1 , S. Ravichandran2
Section:Research Paper, Product Type: Journal Paper
Volume-7 ,
Issue-5 , Page no. 1440-1444, May-2019
CrossRef-DOI: https://doi.org/10.26438/ijcse/v7i5.14401444
Online published on May 31, 2019
Copyright © M. Muthuraman, S. Ravichandran . This is an open access article distributed under the Creative Commons Attribution License, which permits unrestricted use, distribution, and reproduction in any medium, provided the original work is properly cited.
View this paper at Google Scholar | DPI Digital Library
How to Cite this Paper
- IEEE Citation
- MLA Citation
- APA Citation
- BibTex Citation
- RIS Citation
IEEE Style Citation: M. Muthuraman, S. Ravichandran, “A Novel Automated CNN Based Lung Cancer Prediction Technique (CNNLCPT) for CT scan images,” International Journal of Computer Sciences and Engineering, Vol.7, Issue.5, pp.1440-1444, 2019.
MLA Style Citation: M. Muthuraman, S. Ravichandran "A Novel Automated CNN Based Lung Cancer Prediction Technique (CNNLCPT) for CT scan images." International Journal of Computer Sciences and Engineering 7.5 (2019): 1440-1444.
APA Style Citation: M. Muthuraman, S. Ravichandran, (2019). A Novel Automated CNN Based Lung Cancer Prediction Technique (CNNLCPT) for CT scan images. International Journal of Computer Sciences and Engineering, 7(5), 1440-1444.
BibTex Style Citation:
@article{Muthuraman_2019,
author = {M. Muthuraman, S. Ravichandran},
title = {A Novel Automated CNN Based Lung Cancer Prediction Technique (CNNLCPT) for CT scan images},
journal = {International Journal of Computer Sciences and Engineering},
issue_date = {5 2019},
volume = {7},
Issue = {5},
month = {5},
year = {2019},
issn = {2347-2693},
pages = {1440-1444},
url = {https://www.ijcseonline.org/full_paper_view.php?paper_id=4427},
doi = {https://doi.org/10.26438/ijcse/v7i5.14401444}
publisher = {IJCSE, Indore, INDIA},
}
RIS Style Citation:
TY - JOUR
DO = {https://doi.org/10.26438/ijcse/v7i5.14401444}
UR - https://www.ijcseonline.org/full_paper_view.php?paper_id=4427
TI - A Novel Automated CNN Based Lung Cancer Prediction Technique (CNNLCPT) for CT scan images
T2 - International Journal of Computer Sciences and Engineering
AU - M. Muthuraman, S. Ravichandran
PY - 2019
DA - 2019/05/31
PB - IJCSE, Indore, INDIA
SP - 1440-1444
IS - 5
VL - 7
SN - 2347-2693
ER -
![]() |
![]() |
![]() |
245 | 198 downloads | 93 downloads |
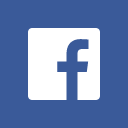
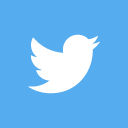
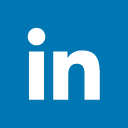
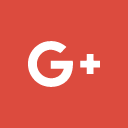
Abstract
There are many incidents of Lung Cancer in the globe. This Cancer is curable if diagnosed early stage, where screening plays an important role in prevention of the disease. Computed Tomography (CT) scans can provide medical information, but its access is limited in rural areas. Computer-aided diagnosis (CAD) which can assist in screening of cancer from medical images can also provide help to doctors in remote areas. Previous studies have promoted and proposed CAD based systems for predicting lung cancer. Their findings have laid the foundation of promise lung cancer diagnosis using the deep learning approaches. This paper proposes and demonstrates a novel Automated CNN Based Lung Cancer Prediction Technique (CNNLCPT), a set of unique steps in image processing for predicting lung cancer from medical CT scans. The accuracy of predictions is also demonstrated in the paper.
Key-Words / Index Term
Automation, Deep Learning, Image processing, Lung cancer, Prediction
References
[1] W. H. Organization, “Cancer,” 2018. [Online]. Available: http: //www.who.int/mediacentre/factsheets/fs297/en/
[2] U. Kauczor, and H. Hatabu, “Standard-dose vs. low-dose ct protocols in the evaluation of localized lung lesions: Capability for lesion characterizationilead study,” European Journal of Radiology Open, vol. 3, pp. 67 – 73, 2016.
[3] Kumar V, Gu Y, Basu S, Berglund A, Eschrich SA, Schabath MB, Forster K, Aerts HJ, Dekker A, Fenstermacher D and Goldgof DB: Radiomics: the process and the challenges. Magnetic resonance imaging, 30(9), pp.1234–1248. (2012)
[4] M. T. Islam, M. A. Aowal, A. T. Minhaz, and K. Ashraf, “Abnormality detection and localization in chest x-rays using deep convolutional neural networks,” vol. abs/1705.09850, 2017
[5] X. Wang, Y. Peng, L. Lu, Z. Lu, M. Bagheri, and R. M. Summers, “Chestx-ray8: Hospital-scale chest x-ray database and benchmarks on weakly-supervised classification and localization of common thorax diseases,” in The IEEE Conference on Computer Vision and Pattern Recognition (CVPR), July 2017.
[6] Hussein S, Gillies R, Cao K, Song Q and Bagci U: Tumornet: Lung nodule characterization using multi-view convolutional neural network with gaussian process. In Biomedical Imaging (ISBI 2017), 2017 IEEE 14th International Symposium on, pp. 1007–1010. IEEE (2017)
[7] Song Q, Zhao L, Luo X and Dou X: Using Deep Learning for Classification of Lung Nodules on Computed Tomography Images. Journal of healthcare engineering (2017) Proc Int Jt Conf Neural Netw. Author manuscript; available in PMC 2018 November 13.
[8] Jiang H, Ma H, Qian W, Wei G, Zhao X and Gao M: A novel pixel value space statistics map of the pulmonary nodule for classification in computerized tomography images. In Engineering in Medicine and Biology
[9] A. K. Jain, Fundamentals of Digital Image Processing. Englewood Cliffs, NJ: Prentice-Hall, 1989.
[10] Z. Zhou, Y. Jiang, Y. Yang, S.Chen “Lung Cancer Cell Identification Based on Artificial Neural Network Ensembles”, Artificial Intelligence in Medicine, 2002, vol.24, no.1, pp.25-36. @Elsevier
[11] A. Kalousis, J. Prados, M. Hilario, “Stability of Feature Selection Algorithms: a study on high dimensional spaces,” Knowledge and information System, vol. 12, no. 1, pp. 95-116, 2007.
[12] Bartlett EC, Walsh SL, Hardavella G, et al. Interobserver Variation in Characterisation of Incidentally-Detected Pulmonary Nodules: An International, Multicenter Study. Available online: http://4wcti.org/2017/SS5-3.cgi
[13] Zinovev D, Feigenbaum J, Furst J, et al. Probabilistic lung nodule classification with belief decision trees. Conf Proc IEEE Eng Med Biol Soc 2011;2011:4493-8.
[14] Ciompi F, Chung K, van Riel SJ, et al. Towards automatic pulmonary nodule management in lung cancer screening with deep learning. Sci Rep 2017;7:46479.
[15] Aerts HJ, Velazquez ER, Leijenaar RT, et al. Decoding tumour phenotype by noninvasive imaging using a quantitative radiomics approach. Nat Commun 2014;5:4006.