Glucose Level Prediction of LIBREPRO CGM Sensor Data Using Machine Learning Algorithm for Enhanced Diabetes Mellitus Management
Rekha Phadke1 , Varsha Prasad2 , H C Nagaraj3 , K P Nagesh4
Section:Research Paper, Product Type: Journal Paper
Volume-7 ,
Issue-5 , Page no. 1571-1582, May-2019
CrossRef-DOI: https://doi.org/10.26438/ijcse/v7i5.15711582
Online published on May 31, 2019
Copyright © Rekha Phadke, Varsha Prasad, H C Nagaraj, K P Nagesh . This is an open access article distributed under the Creative Commons Attribution License, which permits unrestricted use, distribution, and reproduction in any medium, provided the original work is properly cited.
View this paper at Google Scholar | DPI Digital Library
How to Cite this Paper
- IEEE Citation
- MLA Citation
- APA Citation
- BibTex Citation
- RIS Citation
IEEE Style Citation: Rekha Phadke, Varsha Prasad, H C Nagaraj, K P Nagesh, “Glucose Level Prediction of LIBREPRO CGM Sensor Data Using Machine Learning Algorithm for Enhanced Diabetes Mellitus Management,” International Journal of Computer Sciences and Engineering, Vol.7, Issue.5, pp.1571-1582, 2019.
MLA Style Citation: Rekha Phadke, Varsha Prasad, H C Nagaraj, K P Nagesh "Glucose Level Prediction of LIBREPRO CGM Sensor Data Using Machine Learning Algorithm for Enhanced Diabetes Mellitus Management." International Journal of Computer Sciences and Engineering 7.5 (2019): 1571-1582.
APA Style Citation: Rekha Phadke, Varsha Prasad, H C Nagaraj, K P Nagesh, (2019). Glucose Level Prediction of LIBREPRO CGM Sensor Data Using Machine Learning Algorithm for Enhanced Diabetes Mellitus Management. International Journal of Computer Sciences and Engineering, 7(5), 1571-1582.
BibTex Style Citation:
@article{Phadke_2019,
author = {Rekha Phadke, Varsha Prasad, H C Nagaraj, K P Nagesh},
title = {Glucose Level Prediction of LIBREPRO CGM Sensor Data Using Machine Learning Algorithm for Enhanced Diabetes Mellitus Management},
journal = {International Journal of Computer Sciences and Engineering},
issue_date = {5 2019},
volume = {7},
Issue = {5},
month = {5},
year = {2019},
issn = {2347-2693},
pages = {1571-1582},
url = {https://www.ijcseonline.org/full_paper_view.php?paper_id=4452},
doi = {https://doi.org/10.26438/ijcse/v7i5.15711582}
publisher = {IJCSE, Indore, INDIA},
}
RIS Style Citation:
TY - JOUR
DO = {https://doi.org/10.26438/ijcse/v7i5.15711582}
UR - https://www.ijcseonline.org/full_paper_view.php?paper_id=4452
TI - Glucose Level Prediction of LIBREPRO CGM Sensor Data Using Machine Learning Algorithm for Enhanced Diabetes Mellitus Management
T2 - International Journal of Computer Sciences and Engineering
AU - Rekha Phadke, Varsha Prasad, H C Nagaraj, K P Nagesh
PY - 2019
DA - 2019/05/31
PB - IJCSE, Indore, INDIA
SP - 1571-1582
IS - 5
VL - 7
SN - 2347-2693
ER -
![]() |
![]() |
![]() |
2486 | 256 downloads | 93 downloads |
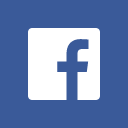
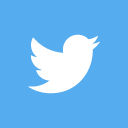
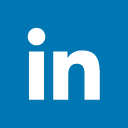
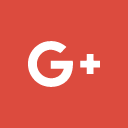
Abstract
"India now carries 20 percent of the global burden of diabetes. There is an immense need and progress to be made to identify the possible fluctuation of blood glucose before hand with minimal errors and thereby enabling proactive decision making. As per statistics one in 15 people in UK have diabetes, including one million people who have type 2, but haven`t been diagnosed. In this paper, focus is to use data science(An interdisciplinary field that uses skills from various fields such as statistics machine learning, artificial intelligence, visualization etc. ) algorithms like time series machine learning to derive meaningful and appropriate information from large volumes of blood glucose level and related data for precise forecasting of upcoming blood glucose level fluctuations. Not only can the patient and physician be informed beforehand, to avert complications, but it also aids in predicting response to certain medications with ease. In this case, time series machine learning algorithm is implemented on 15 days LIBRREPRO Continuous Glucose Monitoring (CGM) Sensor dataset of 10 different patients. A comparison of performance evaluation metrics of the different time series machine learning algorithms is drawn. Simple exponential smoothing(SES) Algorithm, with alpha and beta of 0.99 provided the least Root Mean Square Error (RMSE) of 7.98mg/dL for 15-minute prediction, 19.47mg/dL for 30-minute prediction. The Theil’s U coefficient was 0.12 for 15-minute, 0.39 for 30-minute prediction.
Key-Words / Index Term
Glucose Prediction, Machine Learning, SES, MA, RMSE, Theil’s U, LIBREPRO, CGM Sensor, Data Science, Time Series Forecasting, Moving Window Walk Forward Validation
References
[1] Centers for Disease Control and Prevention. National Diabetes Fact Sheet: National Estimates and General Information on Diabetes and Prediabetes in the United States, 2011. Atlanta, GA, U.S. Department of Health and Human Services, Centers for Disease Control and Prevention, 2011
[2] International Diabetes Federetion, IDF diabetes atlas.Technical report, 2013.
[3] K. Plis, r. j. shubrook and F. schwartz, "A machine learning apprroach to predicting blood glucose levels for diabetes management," Modern artificial intelligence for health analytics, pp. 1-14, 2014.
[4] G. Sparacino, F. Zanderigo, S. corazza, A. Maran, A. FAcchinetti and C. Cobelli, "Glucose concentration can be predicted ahead in time from continuous glucose monitoring sensor time series," Biomedical engineering IEEE Transactions, pp. 931-937, 2007.
[5] M. Jensen, T. F. Cristensen, L. Tarnow, E. Seto, M. Johansen and O. Hejlesen, "Real time hypoglycemia detection from continuous glucose monitoring data of subjects with type 1 diabetes," Diabetes technology and theraupeutics 15(7), 2013.
[6] C. Marling, M. wiley, R. Buneseu, J. Shudrook and F. Schwartz, "Emerging Applications for intelligent diabetes management," AI MAgazine, vol. 67, 2012.
[7] K. Polat and S. Gunes, "An expert system approach based on principle component analysis and adaptive neuro fuzzy inference system to diagonisis of diabetes disease," Science Direct, pp. 702-710, 2007.
[8] S. Polat, K. Gunes and A. Arslan, "A cascade learning system for classification of diabetes disease:Generalised disciminant analysis and least squared support vector machine," Expert systems with applications, pp. 482-487, 2008.
[9] G.Baghdadi and A. Nasrabadi, "Controlling blood levels in diabetics by neural network predictor," Engineering in medicine and biology society, pp. 3216-3219, August 2007.
[10] C.Zecchin, A. Facchinetti, G. Sparacino, G. D. Nicolao and C. Cobelli, "Neural network incorporating meal information improves accuracy of shorttime predictions of glucose concentration," IEEE Transactions on Biomedical Engineering, 2012.
[11] T. e. al., "Artificial neural network for blood glucose level prediction," in International conference on smart,monitored and controlled cities, 2017.
[12] S. Pappada, B. D. Cameron, P. M. Rosman, R. E. Bourey, T. J. Papadimos, W. Olorunto and M. J. Borst, "Neural Network based real time prediction of glucose in patients with insulin dependent diabetes," Diabetes technology and Therapeutics, pp. 135-141, 2011.
[13] MeriyanEren-Orukulu, A. Cinar, L. Quinn and D. smith, "Estimation of future glucose concentrations with subject specific recursive linear models," Diabetes technology and Theraupetics, pp. 243-253, 2009.
[14] V.Petridis, A. KehagiasL, PetrouA, BakirtzisS, Kiartzish, PanagiotouN and Masalaris, "A bayesian multiple models combination method for time series prediction," Journal of Intelligence and robotic systems, vol. 31, no. 1-3, pp. 69-89, may 2001.
[15] R. J, S. Rajaraman, A. Gribok and K. W. ward, "Predictive monitoring for improved management of glucose levels," Journal of diabetes science and technology, pp. 478-486.
[16] Lynn Kennedy, Adam Brown, Äbotts freestyle librepro professional CGM system.
[17] Freestyle Libre 14 days users manual
[18] P. HAmmond, Interpreting the ambulance glucose profile, vol. 16, 2016.
[19] Rob J Hyndman, George Athanasopoulous, "Forecasting principles and practice" 2nd edition may 2018.
[20] Zbikowski and Kamil, "Using Volume weighted support vector machines with walk forward testing and feature selection for the purpose of creating stock trading strategy," Elsevier,Expert systems with applications, vol. 42, 2014.
[21] S. Makridakis and S. C. Wheelright, "Forecasting methods and applications," 2018.
[22] GalitScmueli and K. C. Lichtendahl Jr, "Practical time series forecasting with R-A hands on guide," 2016.
[23] R. J. Hyndman, "Forecasting: Principles and Practice-Exponential Smoothing II".
[24] R. Hyndman and A. Muhammad, "Some Nonlinear Exponential Smoothing models are unstable," 2006.
[25] C. Marling and C. Bunescu, "The OhioT1DM dataset for blood glucose level prediction," in III International workshop on knowledge discovery in healthcare data, Stockholm,Sweden, July 13 2018.