Factors Influencing Infection Source Identification in Complex Networks: An Empirical Study
Syed Shafat Ali1 , Syed Afzal Murtaza Rizvi2
Section:Research Paper, Product Type: Journal Paper
Volume-7 ,
Issue-5 , Page no. 1791-1804, May-2019
CrossRef-DOI: https://doi.org/10.26438/ijcse/v7i5.17911804
Online published on May 31, 2019
Copyright © Syed Shafat Ali, Syed Afzal Murtaza Rizvi . This is an open access article distributed under the Creative Commons Attribution License, which permits unrestricted use, distribution, and reproduction in any medium, provided the original work is properly cited.
View this paper at Google Scholar | DPI Digital Library
How to Cite this Paper
- IEEE Citation
- MLA Citation
- APA Citation
- BibTex Citation
- RIS Citation
IEEE Style Citation: Syed Shafat Ali, Syed Afzal Murtaza Rizvi, “Factors Influencing Infection Source Identification in Complex Networks: An Empirical Study,” International Journal of Computer Sciences and Engineering, Vol.7, Issue.5, pp.1791-1804, 2019.
MLA Style Citation: Syed Shafat Ali, Syed Afzal Murtaza Rizvi "Factors Influencing Infection Source Identification in Complex Networks: An Empirical Study." International Journal of Computer Sciences and Engineering 7.5 (2019): 1791-1804.
APA Style Citation: Syed Shafat Ali, Syed Afzal Murtaza Rizvi, (2019). Factors Influencing Infection Source Identification in Complex Networks: An Empirical Study. International Journal of Computer Sciences and Engineering, 7(5), 1791-1804.
BibTex Style Citation:
@article{Ali_2019,
author = {Syed Shafat Ali, Syed Afzal Murtaza Rizvi},
title = {Factors Influencing Infection Source Identification in Complex Networks: An Empirical Study},
journal = {International Journal of Computer Sciences and Engineering},
issue_date = {5 2019},
volume = {7},
Issue = {5},
month = {5},
year = {2019},
issn = {2347-2693},
pages = {1791-1804},
url = {https://www.ijcseonline.org/full_paper_view.php?paper_id=4489},
doi = {https://doi.org/10.26438/ijcse/v7i5.17911804}
publisher = {IJCSE, Indore, INDIA},
}
RIS Style Citation:
TY - JOUR
DO = {https://doi.org/10.26438/ijcse/v7i5.17911804}
UR - https://www.ijcseonline.org/full_paper_view.php?paper_id=4489
TI - Factors Influencing Infection Source Identification in Complex Networks: An Empirical Study
T2 - International Journal of Computer Sciences and Engineering
AU - Syed Shafat Ali, Syed Afzal Murtaza Rizvi
PY - 2019
DA - 2019/05/31
PB - IJCSE, Indore, INDIA
SP - 1791-1804
IS - 5
VL - 7
SN - 2347-2693
ER -
![]() |
![]() |
![]() |
380 | 291 downloads | 165 downloads |
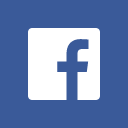
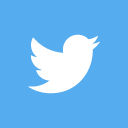
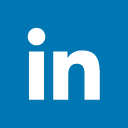
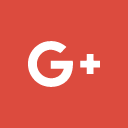
Abstract
One of the important characteristics of the modern-day world is its high connectivity. While it has brought people closer and made lives easier, it has also paved way for harmful content, such as diseases, rumors, computer viruses, etc., to flow easily and spread even quicker. Therefore, finding the source of such unwanted diffusion processes becomes critical to mitigate the damages and avoid future threats. Consequently, infection source identification in complex networks has become an important problem with wide range of effective and meaningful applications. Researchers, over the years, have produced elegant and efficient solutions for the same. The main aim of this paper is to study the factors affecting locating a source of infection. This study largely focuses on four such factors: topology, graph density, infection probability and infection size. For performance analysis, three well known state-of-art source identification techniques, i.e., Dynamic Age (DA), Reverse Infection (RI) and Minimum Description Length (MDL), are employed. Largescale and extensive experiments conducted on various datasets indicate that all the four factors play critical roles in infection source identification, irrespective of the source identification technique employed.
Key-Words / Index Term
Infection Source Identification, Information Diffusion, Social Networks, Complex Networks, SI Model
References
[1] G. S. Campos, A. C. Bandeira, S. I. Sardi, “Zika virus outbreak, Bahia, Brazil”, Emerging Infectious Diseases, Vol. 21, No. 10, pp. 1885, 2015.
[2] WHO Ebola Response Team, “Ebola virus disease in West Africa—The first 9 months of the epidemic and forward projections”, New England J. Med., Vol. 371, No. 16, pp. 1481–1495, 2014.
[3] H. Allcott, M. Gentzkow, “Social media and fake news in the 2016 election”, Journal of Economic Perspectives, Vol. 31, No. 2, pp. 211-36, 2017.
[4] B. Doerr, M. Fouz, T. Friedrich, “Why rumors spread so quickly in social networks”, Commun. ACM, Vol. 55, No. 6, pp. 70–75, Jun. 2012.
[5] C. Pash, “The lure of naked hollywood star photos sent the Internet into meltdown in New Zealand”, Business Insider Australia, Vol. 4, Sep. 2014.
[6] D. MacRae, “5 viruses to be on the alert for in 2014”, Computer Business Review, Tech. Rep., Feb. 2014.
[7] A. Singla, K. Jain, A. Gairola, "Delving into Security of Networks - Times Need", International Journal of Scientific Research in Network Security and Communication, Vol. 2, Issue. 3, pp. 1-8, 2014.
[8] U.K. Singh, C. Joshi, S.K. Singh, "Zero day Attacks Defense Technique for Protecting System against Unknown Vulnerabilities", International Journal of Scientific Research in Computer Science and Engineering, Vol. 5, Issue. 1, pp. 13-18, 2017.
[9] D. Shah, T. Zaman, “Detecting sources of computer viruses in networks: Theory and experiment”, In the Proc. ACM SIGMETRICS Int. Conf. Measur. Model. Comput. Syst. (SIGMETRICS), New York, NY, USA, pp. 203–214, Dec. 2010.
[10] L. J. Allen, “Some discrete-time si, sir, and sis epidemic models”, Mathematical Biosciences, Vol. 124, Issue. 1, pp. 83–105, 1994.
[11] R. M. Anderson, R. M. May, B. Anderson, “Infectious diseases of humans: dynamics and control”, Vol. 28, Wiley Online Library, 1992.
[12] N. Karamchandani, M. Franceschetti, “Rumor source detection under probabilistic sampling”, In the Proc. IEEE Int. Symp. Inf. Theory (ISIT), Istanbul, Turkey, pp. 2184–2188, 2013.
[13] W. Luo, W. P. Tay, M. Leng, “Identifying infection sources and regions in large networks”, IEEE Trans. Signal Process., Vol. 61, No. 11, pp. 2850–2865, Jun. 2013.
[14] D. T. Nguyen, N. P. Nguyen, M. T. Thai, “Sources of misinformation in online social networks: Who to suspect?”, In the Proc. of 2012 IEEE Milit. Commun. Conf. (MILCOM), Orlando, FL, USA, pp. 1–6, 2012.
[15] W. Luo, W. P. Tay, M. Leng, “How to identify an infection source with limited observations”, IEEE J. Sel. Topics Signal Process., Vol. 8, No. 4, pp. 586–597, Aug. 2014.
[16] W. Luo, W. P. Tay, “Identifying infection sources in large tree networks”, In the Proc. of 9th Annu. 2012 IEEE Commun. Soc. Conf. Sensor Mesh Ad Hoc Commun. Netw. (SECON), Seoul, South Korea, pp. 281–289, 2012.
[17] K. Zhu, L. Ying, “Information source detection in the SIR model: A sample-path-based approach”, IEEE/ACM Transactions on Networking (TON), Vol. 24, Issue 1, pp. 408-421, 2016.
[18] V. Fioriti, M. Chinnici, J. Palomo, “Predicting the sources of an outbreak with a spectral technique”, Appl. Math. Sci., Vol. 8, No. 135, pp. 6775–6782, 2014.
[19] W. Luo, W. P. Tay, “Identifying multiple infection sources in a network”, In the Proc. of 2012 Conf. Rec. 46th Asilomar Conf. Signals Syst. Comput. (ASILOMAR), Pacific Grove, CA, USA, pp. 1483–1489, 2012.
[20] B. A. Prakash, J. Vreeken, C. Faloutsos, “Spotting culprits in epidemics: How many and which ones?”, In the Proc. of 2012 IEEE 12th Int. Conf. Data Min. (ICDM), Brussels, Belgium, pp. 11–20, 2012.
[21] B. A. Prakash, J. Vreeken, C. Faloutsos, “Efficiently spotting the starting points of an epidemic in a large graph”, Knowl. Inf. Syst., Vol. 38, No. 1, pp. 35–59, 2014.
[22] J. Leskovec, J. J. Mcauley, “Learning to discover social circles in ego networks, In Advances in Neural Information Processing Systems, Curran Associates, Inc., pp. 539–547, 2012.
[23] D. J. Watts, S. H. Strogatz, “Collective dynamics of ‘small-world’ networks”, Nature, Vol. 393, No. 6684, pp. 440–442, 1998.
[24] P. Erdos, A. Rényi, “On random graphs I”, Publ. Math. Debrecen, 6, pp. 290-297, 1959.
[25] P. Erdos, A. Rényi, “On the evolution of random graphs”, Publ. Math. Inst. Hung. Acad. Sci, Vol. 5, No. 1, pp. 17-60, 1960.
[26] J. G. Restrepo, E. Ott, and B. R. Hunt, “Characterizing the dynamical importance of network nodes and links,” Phys. Rev. Lett., Vol. 97, No. 9, Art. No. 094102, Sep. 2006.
[27] J. Jiang, S. Wen, S. Yu, Y. Xiang, W. Zhou, “K-center: An approach on the multi-source identification of information diffusion”, IEEE Transactions on Information Forensics and Security, Vol. 10, Issue. 12, pp. 2616-2626, 2015.
[28] K. Yang, A. H. Shekhar, D. Oliver, and S. Shekhar, “Capacity constrained network-Voronoi diagram: A summary of results”, In Advances in Spatial and Temporal Databases, Berlin, Germany: Springer-Verlag, pp. 56–73, 2013.
[29] D. Brockmann and D. Helbing, “The hidden geometry of complex, network-driven contagion phenomena”, Science, Vol. 342, No. 6164, pp. 1337–1342, 2013.
[30] Z. Wang, C. Wang, J. Pei, X. Ye, “Multiple Source Detection without Knowing the Underlying Propagation Model”, In the Proceedings of the Thirty-First 2017 AAAI Conference on Artificial Intelligence (AAAI-17), San Francisco, California, USA, pp. 217-223, 2017.
[31] K. Zhu, Z. Chen, L. Ying, “Catch `Em All: Locating Multiple Diffusion Sources in Networks with Partial Observations”, In the Proceedings of the Thirty-First 2017 AAAI Conference on Artificial Intelligence (AAAI-17), San Francisco, California, USA, pp. 1676-1683, 2017.
[32] P. C. Pinto, P. Thiran, and M. Vetterli, “Locating the source of diffusion in large-scale networks”, Phys. Rev. Lett., Vol. 109, No. 6, Art. No. 068702, Aug. 2012.
[33] A. Louni, K. P. Subbalakshmi, “Who Spread That Rumor: Finding the Source of Information in Large Online Social Networks With Probabilistically Varying Internode Relationship Strengths”, IEEE Transactions on Computational Social Systems, Vol. 5, Issue. 2, pp. 335-343, 2018.
[34] A. Agaskar and Y. M. Lu, “A fast Monte Carlo algorithm for source localization on graphs”, In the Proc. of 2013 SPIE Opt. Eng. Appl., San Diego, CA, USA, Art. No. 88581N, 2013.
[35] F. Altarelli, A. Braunstein, L. Dall’Asta, A. Lage-Castellanos, and R. Zecchina, “Bayesian inference of epidemics on networks via belief propagation”, Phys. Rev. Lett., Vol. 112, No. 11, Art. No. 118701, 2014.
[36] Y. Xie, V. Sekar, D. A. Maltz, M. K. Reiter, and H. Zhang, “Worm origin identification using random moonwalks”, in Proc. IEEE Symp. Security Privacy, Oakland, CA, USA, pp. 242–256, 2005.
[37] A. Y. Lokhov, M. Mézard, H. Ohta, and L. Zdeborová, “Inferring the origin of an epidemic with a dynamic message-passing algorithm”, Phys. Rev. E, Vol. 90, No. 1, Art. No. 012801, 2013.
[38] L. C. Freeman, “Centrality in social networks conceptual clarification”, Soc. Netw., Vol. 1, No. 3, pp. 215–239, 1978.
[39] M. E. J. Newman, “Epidemics on networks, in Networks: An Introduction”, Oxford, U.K.: Oxford Univ. Press, Ch. 17, pp. 700–750, 2010.
[40] B. Chang, E. Chen, F. Zhu, Q. Liu, T. Xu, Z. Wang, “Maximum a Posteriori Estimation for Information Source Detection”, IEEE Trans. on Systems, Man, and Cybernetics: Systems (Early Access), pp. 1-15, May, 2018.
[41] Z. Chen, K. Zhu, and L. Ying, “Detecting multiple information sources in networks under the SIR model,” in Proc. 48th Annu. Conf. Inf. Sci.Syst. (CISS), Mar. 2014, pp. 1–4.