Video Face Recognization Using Autoencoder and Softmax Classifications
Sonika Koganti1 , Talluri Sunil Kumar2
Section:Research Paper, Product Type: Journal Paper
Volume-7 ,
Issue-6 , Page no. 491-496, Jun-2019
CrossRef-DOI: https://doi.org/10.26438/ijcse/v7i6.491496
Online published on Jun 30, 2019
Copyright © Sonika Koganti, Talluri Sunil Kumar . This is an open access article distributed under the Creative Commons Attribution License, which permits unrestricted use, distribution, and reproduction in any medium, provided the original work is properly cited.
View this paper at Google Scholar | DPI Digital Library
How to Cite this Paper
- IEEE Citation
- MLA Citation
- APA Citation
- BibTex Citation
- RIS Citation
IEEE Style Citation: Sonika Koganti, Talluri Sunil Kumar, “Video Face Recognization Using Autoencoder and Softmax Classifications,” International Journal of Computer Sciences and Engineering, Vol.7, Issue.6, pp.491-496, 2019.
MLA Style Citation: Sonika Koganti, Talluri Sunil Kumar "Video Face Recognization Using Autoencoder and Softmax Classifications." International Journal of Computer Sciences and Engineering 7.6 (2019): 491-496.
APA Style Citation: Sonika Koganti, Talluri Sunil Kumar, (2019). Video Face Recognization Using Autoencoder and Softmax Classifications. International Journal of Computer Sciences and Engineering, 7(6), 491-496.
BibTex Style Citation:
@article{Koganti_2019,
author = {Sonika Koganti, Talluri Sunil Kumar},
title = {Video Face Recognization Using Autoencoder and Softmax Classifications},
journal = {International Journal of Computer Sciences and Engineering},
issue_date = {6 2019},
volume = {7},
Issue = {6},
month = {6},
year = {2019},
issn = {2347-2693},
pages = {491-496},
url = {https://www.ijcseonline.org/full_paper_view.php?paper_id=4578},
doi = {https://doi.org/10.26438/ijcse/v7i6.491496}
publisher = {IJCSE, Indore, INDIA},
}
RIS Style Citation:
TY - JOUR
DO = {https://doi.org/10.26438/ijcse/v7i6.491496}
UR - https://www.ijcseonline.org/full_paper_view.php?paper_id=4578
TI - Video Face Recognization Using Autoencoder and Softmax Classifications
T2 - International Journal of Computer Sciences and Engineering
AU - Sonika Koganti, Talluri Sunil Kumar
PY - 2019
DA - 2019/06/30
PB - IJCSE, Indore, INDIA
SP - 491-496
IS - 6
VL - 7
SN - 2347-2693
ER -
![]() |
![]() |
![]() |
313 | 244 downloads | 115 downloads |
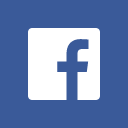
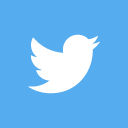
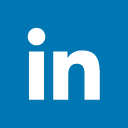
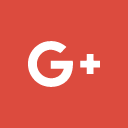
Abstract
Abundance and obtainability of audiovisual capturing devices, like mobile phones and loop camera, have prompted analysis in videocassette face appearance perseption, that is extraordinarily relevant in impostion solicitations .While this methodologies are declared high precisions at equivalent error rates, enactment at lesser lying acceptance rates wants significant development. So, we tend to introduced a completely unique face verification rule, 1st the feature-rich frames are designated from a video sequence .Frame choice done by illustration learning-based feature extraction, is finished by using: 1) deep learning, combining of stacked demising distributed auto-encoder 2) deep Boltzmann classifier (DBC) 3) apprising the loss purpose of DBC by as well as distributed and short rank regularization. Finally, the results verified on 2 wide conferred databases, YouTube and little videos and Shoot Challenge.
Key-Words / Index Term
Face Verification, Neural Networks, DBC, YouTube, Tiny videos
References
[1] Facial recognition technology safeguards Beijing Olympics, accessed on Mar. 10, 2017 [Online]. Available: http://english.cas.cn/resources/archive/china_archive/cn2008/200909/t20090923_42959.shtml.
[2] L. Wolf, T. Hassner, and I. Maoz, “Face recognition in unconstrained videos with matched background similarity,” in Proc. IEEE Conf. Comput. Vis. Pattern Recognit., Jun. 2011, pp. 529–534.
[3] L. Wolf and N. Levy, “The SVM-minus similarity score for video face recognition,” in Proc. IEEE Conf. Comput. Vis. Pattern Recognit., Jun. 2013, pp. 3523–3530.
[4] H. Li, G. Hua, Z. Lin, J. Brandt, and J. Yang, “Probabilistic elastic matching for pose variant face verification,” in Proc. IEEE Conf. Comput. Vis. Pattern Recognit., Jun. 2013, pp. 3499–3506.
[5] Z. Cui, W. Li, D. Xu, S. Shan, and X. Chen, “Fusing robust face region descriptors via multiple metric learning for face recognition in the wild,” in Proc. IEEE Conf. Comput. Vis. Pattern Recognit., Jun. 2013, pp. 3554–3561.
[6] H. Méndez-Vázquez, Y. Martínez-Díaz, and Z. Chai, “Volume structured ordinal features with background similarity measure for video face recognition,” in Proc. Int. Conf. Biometrics (ICB), Jun. 2013, pp. 1–6.
[7]H. S. Bhatt, R. Singh, and M. Vatsa, “On recognizing faces in videos using clustering-based re-ranking and fusion,” IEEE Trans. Inf. Forensics Security, vol. 9, no. 7, pp. 1056–1068, Jul. 2014.
[8] J. Y. Junlin Hu, J. Lu, and Y.-P. Tan, “Large margin multi-metric learning for face and kinship verification in the wild,” in Proc. Asian Conf. Comput. Vis., 2014, pp. 252–267.