Review on Performance Analysis of Dense Micro-block Difference and SURF Method for Texture Classification
Ankita Boni1 , Sagar Shinde2
Section:Review Paper, Product Type: Journal Paper
Volume-7 ,
Issue-6 , Page no. 571-574, Jun-2019
CrossRef-DOI: https://doi.org/10.26438/ijcse/v7i6.571574
Online published on Jun 30, 2019
Copyright © Ankita Boni, Sagar Shinde . This is an open access article distributed under the Creative Commons Attribution License, which permits unrestricted use, distribution, and reproduction in any medium, provided the original work is properly cited.
View this paper at Google Scholar | DPI Digital Library
How to Cite this Paper
- IEEE Citation
- MLA Citation
- APA Citation
- BibTex Citation
- RIS Citation
IEEE Style Citation: Ankita Boni, Sagar Shinde, “Review on Performance Analysis of Dense Micro-block Difference and SURF Method for Texture Classification,” International Journal of Computer Sciences and Engineering, Vol.7, Issue.6, pp.571-574, 2019.
MLA Style Citation: Ankita Boni, Sagar Shinde "Review on Performance Analysis of Dense Micro-block Difference and SURF Method for Texture Classification." International Journal of Computer Sciences and Engineering 7.6 (2019): 571-574.
APA Style Citation: Ankita Boni, Sagar Shinde, (2019). Review on Performance Analysis of Dense Micro-block Difference and SURF Method for Texture Classification. International Journal of Computer Sciences and Engineering, 7(6), 571-574.
BibTex Style Citation:
@article{Boni_2019,
author = {Ankita Boni, Sagar Shinde},
title = {Review on Performance Analysis of Dense Micro-block Difference and SURF Method for Texture Classification},
journal = {International Journal of Computer Sciences and Engineering},
issue_date = {6 2019},
volume = {7},
Issue = {6},
month = {6},
year = {2019},
issn = {2347-2693},
pages = {571-574},
url = {https://www.ijcseonline.org/full_paper_view.php?paper_id=4593},
doi = {https://doi.org/10.26438/ijcse/v7i6.571574}
publisher = {IJCSE, Indore, INDIA},
}
RIS Style Citation:
TY - JOUR
DO = {https://doi.org/10.26438/ijcse/v7i6.571574}
UR - https://www.ijcseonline.org/full_paper_view.php?paper_id=4593
TI - Review on Performance Analysis of Dense Micro-block Difference and SURF Method for Texture Classification
T2 - International Journal of Computer Sciences and Engineering
AU - Ankita Boni, Sagar Shinde
PY - 2019
DA - 2019/06/30
PB - IJCSE, Indore, INDIA
SP - 571-574
IS - 6
VL - 7
SN - 2347-2693
ER -
![]() |
![]() |
![]() |
271 | 257 downloads | 104 downloads |
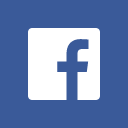
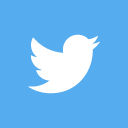
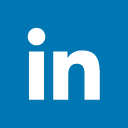
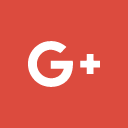
Abstract
The paper proposes a novel picture portrayal for surface characterization. The ongoing headways in the field of fix based highlights compressive detecting and highlight encoding are joined to plan a hearty picture descriptor. In our methodology, we initially propose the neighbourhood highlights, Dense Micro-square Difference (DMD), which catches the nearby structure from the picture patches at high scales. Rather than the pixel we process the little squares from pictures which catch the miniaturized scale structure from it. DMD can be figured productively utilizing vital pictures. The highlights are then encoded utilizing Fisher Vector strategy to get a picture descriptor which thinks about the higher request measurements. The proposed picture portrayal is joined with straight SVM classifier. The analysis is done on the standard surface datasets (KTH-TIPS-2a, Brodatz and Curet). On KTH-TIPS-2a dataset the proposed strategy beats the best revealed outcomes by 5.5% and has a practically identical exhibition to the best in class techniques on the different datasets.
Key-Words / Index Term
Texture classification, descriptors, compressive sensing, SURF
References
[1] Irene Epifanio & Guillermo Ayala, “A Random Set View of Texture Classification,” IEEE Transactions on Image Processing, vol. 11, no. 8, August 2002.
[2] X. Liu & Deliang Wang, “Texture classification using spectral histograms, IEEE Transaction on Image Processing, vol. 12, no.6, June, 2003.
[3] M. Varma & A. Zisserman, “A Statistical Approach to Texture Classification from Single Image,” International Journal of Computer Vision, vol. 62, pp. 61-81, 2005/04/25/2005.
[4] M. Crosier & L. D. Gfiffin, “Texture Classification with a Dictionary of Basic Image Features in Computer Vision & Pattern Recognition, IEEE conference, pages 1-7, June 2008.
[5] T. Ahonen, J. Matas, C. He and M. Pietikainen, “Multiresolution gray scale and Rotation invariant texture classification with local binary patterns”, IEEE Trans. Pattern Anal. Mach. Intell., vol. 24, no. 7, pp. 61-70.
[6] Y. Xu, H. Ji and C. Fermuller, “Viewpoint invariant texture description using fractal analysis”, Int. J. Comput. Vis., vol. 83, no. 1, pp. 85-100, 2009
[7] L. Liu, P. Fieguth, G. Kuang and H. Zha, “Sorted random projections for robust texture classification”, in Proc. IEEE. Int. Conf. Comput. Vis (ICCV), Nov. 2011, pp. 391-398.
[8] Dang Huu Nghi, Luong Chi Mai “Training Data Selection for Support Vector Machines Model” in International Conference on Information and Electronics Engineering IPCSIT vol.6 (2011) IACSIT Press, Singapore.
[9] Anastasia Dubrovina, Pavel Kisilev, Daniel Freedman, Sagi Schein, Ruth Bergman “Efficient and robust image descriptor for GUI object classification” Copyright 2011 Hewlett-Packard Development Company, L.P.
[10] Li Liu & Pawl W. Fiegnth, “Texture Classification from Random Features,” IEEE Transactions on Pattern Analysis & Machine Intelligence, vol. 34, no. 3, March, 2012.
[11] Anna Wojnar and Antonio M. G. Pinheiro, “Annotation of Medical Images Using the SURF descriptor”, 2012 IEEEMs.
[12] M. Mushrif and Y. Dubey, “Texture Classification using Cosine- modultaed Wavelet”, International Journal of Computer and Electrical Engineering, vol. 4, no. 3, June 2012.
[13] J. Sanchez, F. Perronnin, T. Mensink, and J. Verbeek, “Image Classification with the Fisher vector: Theory and Practice,” Int. J. Comput. Vis., vol. 105, no.3, pp. 222-245, 2013.
[14] D. Sanghai and Prof. S. Maniar, ‘Performance evolution of texture classification’, International Journal of Emerging Research in Management & Technology, ISSN: 2278-9359, vol. 2, Issue. 10, Oct. 2013
[15] Dr. Y. Venkateswarlu, Dr. U. Babu and Ch Kumar, “An Efficient Texture Clasification Technique Based on Semi Uniform LBP”, IOSR Journal of Computer Engineering (IOSR- JCE), vol. 16, Issue 5, pp. 36-42, 2014.
[16] A. Vupputuri and S. Meher, “Facial Expression Recognition using Local Binary Patterns and Kulluback Leibler Divergence,” IEEE ICCSP conference, 2015.
[17] Parul Prashar and H. Kundra, “Hybrid Approach for Image Classification using SVM Classifier and SURF Descriptor”, International Journal of Computer Science and Information Technology, Vol. 6(1), 2015, 249-251.