Study of Incentive Compatible Privacy Preserving Data Analysis
Yuvraj Singh1 , Pankaj Pratap Singh2 , Anirudh Kumar Tripathi3 , Amit Kishor4
Section:Research Paper, Product Type: Journal Paper
Volume-7 ,
Issue-6 , Page no. 737-741, Jun-2019
CrossRef-DOI: https://doi.org/10.26438/ijcse/v7i6.737741
Online published on Jun 30, 2019
Copyright © Yuvraj Singh, Pankaj Pratap Singh, Anirudh Kumar Tripathi, Amit Kishor . This is an open access article distributed under the Creative Commons Attribution License, which permits unrestricted use, distribution, and reproduction in any medium, provided the original work is properly cited.
View this paper at Google Scholar | DPI Digital Library
How to Cite this Paper
- IEEE Citation
- MLA Citation
- APA Citation
- BibTex Citation
- RIS Citation
IEEE Style Citation: Yuvraj Singh, Pankaj Pratap Singh, Anirudh Kumar Tripathi, Amit Kishor, “Study of Incentive Compatible Privacy Preserving Data Analysis,” International Journal of Computer Sciences and Engineering, Vol.7, Issue.6, pp.737-741, 2019.
MLA Style Citation: Yuvraj Singh, Pankaj Pratap Singh, Anirudh Kumar Tripathi, Amit Kishor "Study of Incentive Compatible Privacy Preserving Data Analysis." International Journal of Computer Sciences and Engineering 7.6 (2019): 737-741.
APA Style Citation: Yuvraj Singh, Pankaj Pratap Singh, Anirudh Kumar Tripathi, Amit Kishor, (2019). Study of Incentive Compatible Privacy Preserving Data Analysis. International Journal of Computer Sciences and Engineering, 7(6), 737-741.
BibTex Style Citation:
@article{Singh_2019,
author = {Yuvraj Singh, Pankaj Pratap Singh, Anirudh Kumar Tripathi, Amit Kishor},
title = {Study of Incentive Compatible Privacy Preserving Data Analysis},
journal = {International Journal of Computer Sciences and Engineering},
issue_date = {6 2019},
volume = {7},
Issue = {6},
month = {6},
year = {2019},
issn = {2347-2693},
pages = {737-741},
url = {https://www.ijcseonline.org/full_paper_view.php?paper_id=4622},
doi = {https://doi.org/10.26438/ijcse/v7i6.737741}
publisher = {IJCSE, Indore, INDIA},
}
RIS Style Citation:
TY - JOUR
DO = {https://doi.org/10.26438/ijcse/v7i6.737741}
UR - https://www.ijcseonline.org/full_paper_view.php?paper_id=4622
TI - Study of Incentive Compatible Privacy Preserving Data Analysis
T2 - International Journal of Computer Sciences and Engineering
AU - Yuvraj Singh, Pankaj Pratap Singh, Anirudh Kumar Tripathi, Amit Kishor
PY - 2019
DA - 2019/06/30
PB - IJCSE, Indore, INDIA
SP - 737-741
IS - 6
VL - 7
SN - 2347-2693
ER -
![]() |
![]() |
![]() |
212 | 238 downloads | 105 downloads |
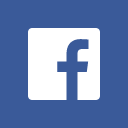
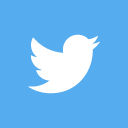
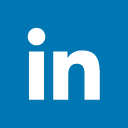
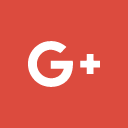
Abstract
In corporate and government department’s increasingly keeping large size electronic databases, which are accessed using internet or intranet. Important information implement from the data using Privacy data mining methods. While performing data mining steps, there is an inherent danger to the privacy of the data. The valuable data stored in the database should not be accessible to users. Most of the privacy preserving methods are based on reduction in the granularity of the implementing of the data. This ends to loss of information but it improves privacy. Therefore, in PPDM there is a conflict between loss of information and the privacy. Effective Methods are required which do not compromise the security mechanisms. Some of the methods proposed for privacy preserving data mining include randomization method, k-anonymity model, l-diversity and distributed privacy preservation. The k-anonymity model is based on a quasi-identifier, which is a collection of attributes in a database that is the identifier for the entire data. All the data in the database is assumed to be in a set of tables, and each tuple is information of an individual customer. K-anonymity Methods are based on the reduction of granularity in representation of data using pseudo identifiers. Major Methods used for granularity reduction are generalization and suppression. In generalization, the attribute values are converted into a range that reduces the granularity and reduces the risk of identifying individual values. In suppression, value of the attribute is removed completely. These methods introduce loss of detail which may affect the accuracy. This induces the search for anonymization algorithms that achieve the required level of anonymization while incurring a minimization of loss of information. Finding optimal anonymous datasets using generalization or suppression has been proved to be a NP hard problem. Therefore, some standard heuristic search Methods such as Genetic Algorithms (GAs), Particle Swam Optimization (PSO) and Ant Colony Optimization (ACO) can be used to find optimal datasets.
Key-Words / Index Term
Data mining, Secure Multiparty Computation, Genetic Algorithm, Particle Swam Optimization, Ant Colony Optimization
References
[1] Xinjing Ge and Jianming Zhu, (2011), Privacy Preserving Data Mining, New Fundamental Technologies in Data Mining.
[2] Agrawal R., Srikant R. Privacy-Preserving Data Mining. Proceedings of the ACM SIGMOD Conference, 2000.
[3] Malin, B., Benitez, K., & Masys, D. (2011). Never too old for anonymity: a statistical standard for demographic data sharing via the HIPAA Privacy Rule. Journal of the American Medical Informatics Association, 18(1), 3-10.
[4] Singh, M. D., Krishna, P. R., & Saxena, A. (2010, January). A cryptography based privacy preserving solution to mine cloud data. In Proceedings of the Third Annual ACM Bangalore Conference (p. 14). ACM.
[5] Patrick Sharkey, Hongwei Tian, Weining Zhang, and Shouhuai Xu, 2008, Privacy-Preserving Data Mining through Knowledge Model Sharing, Springer-Verlag Berlin Heidelberg, pp. 97– 115, 2008
[6] Pawel Jurczyk, Li Xiong, 2008, Privacy-Preserving Data Publishing for Horizontally Partitioned Databases, CIKM’08, October 26–30USA., ACM 978-1-59593-991- 3/08/10.
[7] Campan, A., & Truta, T. (2009). Data and structural k-anonymity in social networks. Privacy, Security, and Trust in KDD, 33-54.
[8] Nergiz, M. E., Clifton, C., & Nerg iz, A. E. (2009). Multirelational k- anonymity. Knowledge and Data Engineering, IEEE Transactions on, 21(8), 110 4-1117.
[9] Stokes, K., & Torra, V. (2012, March). N-Confusion: a generalization of k-anonymity. In Proceedings of the 2012 Joint EDBT/ICDT Workshops (pp. 211-215). ACM.
[10] Cao, J., Karras, P., Kalnis, P., & Tan, K. L. (2011). SABRE: a Sensitive Attribute Bucketization and RE distribution framework for t-closeness. The VLDB Journal, 20(1), 59-81.
[11] Shi, P., Xiong, L., & Fung, B. (2010, October). Anonymizing data with quasi-sensitive attribute values. In Proceedings of the 19th ACM international conference on Information and knowledge management (pp. 1389-1392). ACM.
[12] A. Meyerson, R. Williams, On the complexity of optimal k-anonymity, in: Proc. Of the 23rd ACM SIGMOD-SIGCAT-SIGART Symposium, ACM, New York,NY, 2004, pp. 223–228.
[13] P.Samarati, Protecting respondents’ identities in micro data release, IEEE Transactions on Knowledge and Data Engineering 13 (6) (2001) 1010–1027.
[14] Van der Merwe, D., & Engelbrecht, A. P. (2003). Data clustering using particle swarm optimization. In IEEE congress on evolutionary computation (1) (pp. 215–220). New York: IEEE.
[15] Holden, N., & Freitas, A. (2008).A hybrid PSO/ACO algorithm for discovering classification rules in data mining. Journal of Artificial Evolution and Applications, 2008, 11 pages.
[16] Van den Bergh F. and Engelbrecht A.P., ‘A Cooperative Approach to Particle Swarm Optimization’, IEEE Transactions on Evolutionary Computation, 2004, pp. 225-239.
[17] Premalatha, K., & Natarajan, A.M. (2009). Hybrid PSO and GA for g lobal maximization. Int. J. Open Problems Compt. Math, 2(4), 597-608.
[18] Bayardo R. J., Agrawal R.: Data Privacy through Optimal k-Anonymization. Proceedings of the ICDE Conference, pp. 217–228, 2005.
[19] Sakuma, J., & Kobayashi, S. (2007, July). A genetic algorithm for privacy preserving combinatorial optimization. In Proceedings of the 9th annual conference on Genetic and evolutionary computation (pp. 1372-1379). ACM.
[20] Dehkordi, M. N., Badie, K., & Z adeh, A. K. (2009). A novel method for privacy preserving in association rule mining based on genetic algorithms. Journal of software, 4(6), 555-562.
[21] Matatov, N., Rokach, L., & Maim on, O. (2010). Privacy-preserving data mining: A feature set partitioning approach. Information Sciences, 180(14), 2696-2720.
[22] P. Samarati and L. Sweeney. Protecting privacy when disclosing information: k-anonymity and its enforcement through generalization and suppression. Technical report, CMU, SRI, 1998.
[23] Lefevre, K., Dewitt, D., And Ramakrishnan, R. 2005. Incognito: Efficient full domain k-anonymity. In SIGMOD.
[24] Zhong, S., Yang, Z., And Wright,R. N. 2005. Privacy-enhancing k-anonymization of customer data. In Proceedings of the International Conference on Principles ofHData Systems (PODS).
[25] L. David, Handbook of Genetic Algorithms. New York: Van Nostrand Reinhold. 1991.
[26] D.E. Goldberg, Genetic Algorithms: in Search, Optimization, and Machine Learning. New York: Addison-Wesley Publishing Co. Inc. 1989.
[27] Qing Cao, Tian He, and Tarek Abdelzaher, uCast: Unified Connectionless Multicast for Energy Efficient Content Distribution in Sensor Networks, IEEE Transactions On Parallel And Distributed Systems, Vol. 18, No. 2, February 2007.
[28] Latiff, N.M.A.; Tsimenidis, C.C.; Sharif, B.S., "Performance Comparison of Optimization Algorithms for Clustering in Wireless Sensor Networks," Mobile Adhoc and Sensor Systems, 2007. MASS 2007. IEEE International Conference on , vol., no., pp.1-4, 8-11 Oct. 2007.
[29] Matthew Settles,” An Introduction to Particle Swarm Optimization”, 2005.
[30] Eberhart, R. C., Shi, Y.: Particle swarm optimization: Developments, applications and resources, In Proceedings of IEEE International Conference on Evolutionary Computation, vol. 1 (2001), 81-86.
[31] El-Abd, M., & Kamel, M. (2005). A taxonomy of cooperative search algorithms.Hybrid Metaheuristics, 902-902.