Client Churn Prediction of Banking and fund industry utilizing Machine Learning Techniques
G. Ravi Kumar1 , K. Tirupathaiah2 , B. Krishna Reddy3
Section:Research Paper, Product Type: Journal Paper
Volume-7 ,
Issue-6 , Page no. 842-846, Jun-2019
CrossRef-DOI: https://doi.org/10.26438/ijcse/v7i6.842846
Online published on Jun 30, 2019
Copyright © G. Ravi Kumar, K. Tirupathaiah, B. Krishna Reddy . This is an open access article distributed under the Creative Commons Attribution License, which permits unrestricted use, distribution, and reproduction in any medium, provided the original work is properly cited.
View this paper at Google Scholar | DPI Digital Library
How to Cite this Paper
- IEEE Citation
- MLA Citation
- APA Citation
- BibTex Citation
- RIS Citation
IEEE Style Citation: G. Ravi Kumar, K. Tirupathaiah, B. Krishna Reddy, “Client Churn Prediction of Banking and fund industry utilizing Machine Learning Techniques,” International Journal of Computer Sciences and Engineering, Vol.7, Issue.6, pp.842-846, 2019.
MLA Style Citation: G. Ravi Kumar, K. Tirupathaiah, B. Krishna Reddy "Client Churn Prediction of Banking and fund industry utilizing Machine Learning Techniques." International Journal of Computer Sciences and Engineering 7.6 (2019): 842-846.
APA Style Citation: G. Ravi Kumar, K. Tirupathaiah, B. Krishna Reddy, (2019). Client Churn Prediction of Banking and fund industry utilizing Machine Learning Techniques. International Journal of Computer Sciences and Engineering, 7(6), 842-846.
BibTex Style Citation:
@article{Kumar_2019,
author = {G. Ravi Kumar, K. Tirupathaiah, B. Krishna Reddy},
title = {Client Churn Prediction of Banking and fund industry utilizing Machine Learning Techniques},
journal = {International Journal of Computer Sciences and Engineering},
issue_date = {6 2019},
volume = {7},
Issue = {6},
month = {6},
year = {2019},
issn = {2347-2693},
pages = {842-846},
url = {https://www.ijcseonline.org/full_paper_view.php?paper_id=4640},
doi = {https://doi.org/10.26438/ijcse/v7i6.842846}
publisher = {IJCSE, Indore, INDIA},
}
RIS Style Citation:
TY - JOUR
DO = {https://doi.org/10.26438/ijcse/v7i6.842846}
UR - https://www.ijcseonline.org/full_paper_view.php?paper_id=4640
TI - Client Churn Prediction of Banking and fund industry utilizing Machine Learning Techniques
T2 - International Journal of Computer Sciences and Engineering
AU - G. Ravi Kumar, K. Tirupathaiah, B. Krishna Reddy
PY - 2019
DA - 2019/06/30
PB - IJCSE, Indore, INDIA
SP - 842-846
IS - 6
VL - 7
SN - 2347-2693
ER -
![]() |
![]() |
![]() |
393 | 249 downloads | 121 downloads |
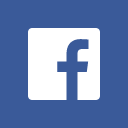
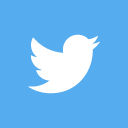
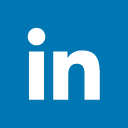
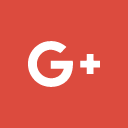
Abstract
With the exceptional challenge and expanding globalization in the money related markets, banking association must create client situated procedures so as to contend effectively in the focused financial condition. Client beat forecast goes for identifying clients with a high inclination to cut ties with an administration or an organization. An exact expectation enables an organization to take activities to the focusing on clients who are well on the way to beat, which can improve the productive utilization of the constrained assets and result in huge effect on business. The fundamental commitment of our work is to build up a client beat forecast model which helps banking and money related organizations to anticipate clients who are in all probability subject to stir. In this investigation we utilized the Decision Tree and Artificial Neural Networks to recognize the clients who are going to beat. In our test results demonstrates that Neural Network system model has showed signs of improvement exactness (86.52%) in contrasted with Decision Tree model (79.77%).
Key-Words / Index Term
Churn, Stir, Decision Tree and Neural Networks
References
[1] Kiansing Ng and Huan Liu, “Customer Retention via Data Mining”, Artificial Intelligence Review, 14(6), 569-590, 2000
[2] Kirui, Clement, Li Hong, Wilson Cheruiyot, and Hillary Kirui. "Predicting customer churn in mobile telephony industry using probabilistic classifiers in data mining." IJCSI International Journal of Computer Science Issues 10, no. 2 (2013): 1694-0784.
[3] N. Lu, H. Lin, J. Lu and G. Zhang, "A Customer Churn Prediction Model in Telecom Industry Using Boosting," in IEEE Transactions on Industrial Informatics, vol. 10, no. 2, pp. 1659-1665, May 2014. doi: 10.1109/TII.2012.2224355.
[4] Bagheri, Mahnaz, M. Valipour, and V. Amin. "The Bankruptcy Prediction in Tehran share holding using Neural Network and its Comparison with Logistic Regression." The Journal of mathematics and computer science 5, no. 3 (2012): 219-228.
[5] Ahna J.H, Hana S.P and Leeb Y.S, “Customer churn analysis: churn determinants and mediation effects of partial defection in the Korean mobile telecommunications service industry”. Telecommunication policy, 30(10), 552-568, 2006
[6] Bose I & Chen X, “Hybrid models using Unsupervised clustering for prediction of customer churn”, Journal of organizational computing & Electronic Commerce, 19(2), 133-151, 2009
[7] A.O. Oyeniyi & A.B. Adeyemo,” Customer Churn Analysis in Banking Sector Using Data Mining Techniques”, African Journal of Computing & ICT, PP 165-174, Vol 8. No. 3 – September, 2015
[8] Hend Sayed, Manal A. Abdel-Fattah, Sherif Kholief, “Predicting Potential Banking Customer Churn using Apache Spark ML and MLlib Packages: A Comparative Study”, International Journal of Advanced Computer Science and Applications, PP 674-677Vol. 9, No. 11, 2018
[9] Jiri Pribil and Michaela Polejova , “A Churn Analysis Using Data Mining Techniques: Case of Electricity Distribution Company”, Proceedings of the World Congress on Engineering and Computer Science 2017 Vol I WCECS 2017, October 25-27, 2017, San Francisco, USA, ISBN: 978-988-14047-5-6
[10] J.Han and M.Kamber, “Data Mining Concepts and Techniques”, the Morgan Kaufmann series in Data Management Systems, 2nd Edition, Morgan Kaufmann, 2006