Medical Image Segmentation: A Review of Recent Techniques, Advancements and a Comprehensive Comparison
Aarish Shafi Dar1 , Devanand Padha2
Section:Review Paper, Product Type: Journal Paper
Volume-7 ,
Issue-7 , Page no. 114-124, Jul-2019
CrossRef-DOI: https://doi.org/10.26438/ijcse/v7i7.114124
Online published on Jul 31, 2019
Copyright © Aarish Shafi Dar, Devanand Padha . This is an open access article distributed under the Creative Commons Attribution License, which permits unrestricted use, distribution, and reproduction in any medium, provided the original work is properly cited.
View this paper at Google Scholar | DPI Digital Library
How to Cite this Paper
- IEEE Citation
- MLA Citation
- APA Citation
- BibTex Citation
- RIS Citation
IEEE Citation
IEEE Style Citation: Aarish Shafi Dar, Devanand Padha, “Medical Image Segmentation: A Review of Recent Techniques, Advancements and a Comprehensive Comparison,” International Journal of Computer Sciences and Engineering, Vol.7, Issue.7, pp.114-124, 2019.
MLA Citation
MLA Style Citation: Aarish Shafi Dar, Devanand Padha "Medical Image Segmentation: A Review of Recent Techniques, Advancements and a Comprehensive Comparison." International Journal of Computer Sciences and Engineering 7.7 (2019): 114-124.
APA Citation
APA Style Citation: Aarish Shafi Dar, Devanand Padha, (2019). Medical Image Segmentation: A Review of Recent Techniques, Advancements and a Comprehensive Comparison. International Journal of Computer Sciences and Engineering, 7(7), 114-124.
BibTex Citation
BibTex Style Citation:
@article{Dar_2019,
author = { Aarish Shafi Dar, Devanand Padha},
title = {Medical Image Segmentation: A Review of Recent Techniques, Advancements and a Comprehensive Comparison},
journal = {International Journal of Computer Sciences and Engineering},
issue_date = {7 2019},
volume = {7},
Issue = {7},
month = {7},
year = {2019},
issn = {2347-2693},
pages = {114-124},
url = {https://www.ijcseonline.org/full_paper_view.php?paper_id=4732},
doi = {https://doi.org/10.26438/ijcse/v7i7.114124}
publisher = {IJCSE, Indore, INDIA},
}
RIS Citation
RIS Style Citation:
TY - JOUR
DO = {https://doi.org/10.26438/ijcse/v7i7.114124}
UR - https://www.ijcseonline.org/full_paper_view.php?paper_id=4732
TI - Medical Image Segmentation: A Review of Recent Techniques, Advancements and a Comprehensive Comparison
T2 - International Journal of Computer Sciences and Engineering
AU - Aarish Shafi Dar, Devanand Padha
PY - 2019
DA - 2019/07/31
PB - IJCSE, Indore, INDIA
SP - 114-124
IS - 7
VL - 7
SN - 2347-2693
ER -
![]() |
![]() |
![]() |
1355 | 682 downloads | 224 downloads |
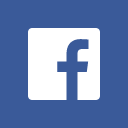
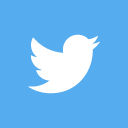
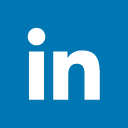
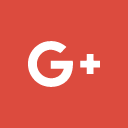
Abstract
Image segmentation is the most critical function in image analysis and processing. Results of segmentation fundamentally affect all subsequent image analysis processes such as representation and description of objects, measurement of features, and even higher-level tasks such as classification of objects. Image segmentation is therefore the most essential and crucial process for facilitating the delineation, characterization and visualization of regions of interest in any medical image. The radiologist`s manual segmentation of the medical image is not just a tedious and time-consuming technique, also not very accurate, especially with the increasing medical imaging modalities and the unmanageable quantity of medical images that need to be examined. It is therefore necessary to review current image segmentation methodologies using automated algorithms that are accurate and require as little user interaction as possible, especially for medical images. In the segmentation process, it is necessary to delineate and extract the anatomical structure or region of interest so that it can be viewed individually. In this paper, we are projecting the important place of image segmentation in decision-making information extraction and deliberating upon current techniques which are used in medical imaging and discussing about various advancements in this research field.
Key-Words / Index Term
Medical Imaging, Segmentation, Watershed Transform (WT), Expectation Maximization (EM), Level Set Method (LSM), Genetic Algorithms (GA), Artificial Neural Networks (ANN)
References
[1] Gonzalez RC, Woods RE. 1992. Digital Image Processing. Reading, MA: Addison-Wesley
[2] P. Soille, Morphological Image Analysis: Principles and Applications. Berlin, Germany: Springer-Verlag, 1999.
[3] L. Xie, R. Hong, B. Zhang, Q. Tian, "Image classification and retrieval are ONE", Proc. ACM Int. Conf. Multimedia Retrieval, pp. 3-10, 2015.
[4] Nameirakpam Dhanachandra, Yabem Jina Chanu and Kumathem Manglem Singh, “Image segmentation using k-means clustering algorithm and subtractive clustering algorithm,” Procedia Computer Science. 2015; 54; 764-771.
[5] N. K. Mishra and M. E. Celebi, “An overview of melanoma detection in dermoscopy images using image processing and machine learning,” arXiv preprint arXiv:1601.07843, 2016.
[6] X.Zhang et al., A multi-scale 3D Otsu thresholding algorithm for medical image segmentation, Digit. Signal Process. (2016), http://dx.doi.org/10.1016/j.dsp.2016.08.003
[7] Han Jie, Yang Chunhua, Zhou Xiaojun, Gui Weihua, A new multi-threshold image segmentation approach using state transition algorithm, Applied Mathematical Modelling (2017), doi: 10.1016/j.apm.2017.02.015
[8] Song, C. Yang, L. Fan, K. Wang, F. Yang, S. Liu, J. Tian, Lung lesion extraction using a toboggan based growing automatic segmentation approach, IEEETrans. Med. Imaging 99(2015)1, http://dx.doi.org/10.1109/TMI.2015.2474119.
[9] M.A. Mohammed, M.K.A. Ghani, R.I. Hamed,M.K. Abdullah, D.A. Ibrahim, Automatic Segmentation and Automatic Seed Point Selection of Nasopharyngeal Carcinoma from Microscopy Images Using Region Growing Based Approach, Journal of Computational Science (2017), http://dx.doi.org/10.1016/j.jocs.2017.03.009
[10] Y. Li, X. Bai, L. Jiao, Y. Xue, Partitioned-cooperative quantum-behaved particle swarm optimization based on multilevel thresholding applied to medical image segmentation, Appl. Soft Comput. J. 56 (2017) 345–356. doi:10.1016/j.asoc.2017.03.018.
[11] Rouhi, R., et al. Benign and malignant breast tumors classification based on region growing and CNN segmentation. Expert Systems with Applications (2014), http://dx.doi.org/10.1016/j.eswa.2014.09.020
[12] Tyan, Y-S.; Wu, M-C.; Chin, C-L.; Kuo, Y-L.; Lee, M-S.;Chang, H-Y.: Ischemic stroke detection system with a computer-aided diagnostic ability using an unsupervised feature perception enhancement method. Int. J. Biomed. Imaging2014, 12, Article ID947539 (2014). https://doi.org/10.1155/2014/947539
[13] M. Nikolic, E. Tuba, and M. Tuba, “Edge detection in medical ultra-sound images using adjusted Canny edge detection algorithm,” in24thTelecommunications Forum TELFOR. IEEE, 2016, pp. 691–694.
[14] W. S. Oliveira, J. V. Teixeira, T. I. Ren, G. D. Cavalcanti, J. Sijbers, Unsupervised retinal vessel segmentation using combined filters, PloS one 11 (2) (2016) e0149943
[15] X. Fan, X. Wang, S. Wang, A fuzzy edge-weighted centroidal Voronoi tessellation model for image segmentation, Comput. Math. Appl.(2015) http://dx.doi.org/10.1016/j.camwa.2015.11.003
[16] Aganj, M. G. Harisinghani, R. Weissleder, and B. Fischl, ‘‘Unsupervised medical image segmentation based on the local center of mass,’’Sci. Rep.,vol. 8, no. 1, Aug. 2018, Art. no. 13012.
[17] Lay Khoon Lee et, al.: A review of image segmentation methodologies in medical images. Springer international publishing Switzerland 2015
[18] Rouhi, R., Jafari, M., 2016. Classification of benign and malignant breast tumors based on hybrid level set segmentation. Expert Systems with Applications 46, 45–59.
[19] Jean-Baptiste Fasquel and Nicolas Delanoue. A graph-based image interpretation method using a priori qualitative inclusion and photometric relationships. IEEE Transactions on Pattern Analysis and Machine Intelligence, 2017.
[20] Ghosha, P., Mitchellb, M., Tanyid, J.A. and Hungd, A.Y., (2016) Incorporating Priors for Medical Image Segmentation Using a Genetic Algorithm, Neurocomputing, 195,181-194.
[21] Mohammed MA, Ghani MKA, Hamed RI, Ibrahim DA, Abdullah MK (2017) Artificial neural networks for automatic segmentation and identification of nasopharyngeal carcinoma. J Comput Sci 21:263–274
[22] Lee, L. K., Liew, S. C., & Thong, W. J. (2015). A review of image segmentation methodologies in medical image. Advanced computer and communication engineering technology (pp. 1069–1080). Springer International Publishing
[23] Ma Z, Tavares JMRS, Jorge RMN, Mascaranhas T. 2010. A review of algorithms for medical image segmentation and their applications to the female pelvic cavity. Comput Method Biomech Biomed Eng. 13(2):235–246.
[24] M. Rajchl, J. S. Baxter, A. J. McLeod, J. Yuan, W. Qiu, T. M. Peters, and A. R. Khan, “Hierarchical max-flow segmentation framework for multiatlas segmentation with kohonen self-organizing map based gaussian mixture modeling,” Medical image analysis, vol. 27, pp. 45–56, 2016.
[25] Y. Feng, H. Zhao, X. Li, X. Zhang, and H. Li, “A multi-scale 3D Otsu thresholding algorithm for medical image segmentation,” Digital Signal Processing, vol. 60, pp. 186-199, 2017.
[26] Zhou, C., Tian, L., Zhao, H., & Zhao, K. (2015). A method of two-dimensional Otsu image threshold segmentation based on improved firefly algorithm. In Proceeding of IEEE
[27] International conference on cyber technology in automation, control, and intelligent systems, 2015 (pp. 1420–1424). N. Greggio et al., “Fast estimation of Gaussian mixture models for image segmentation,” Mach. Vis. App., Special Issue: Microscopy Image Analysis for Biomedical Applications, 23(4), 773–789 (2012).
[28] Kumar, D.; Pramanik, A.; Kar, S.S.; Maity, S.P. Retinal blood vessel segmentation using matched filterand laplacian of gaussian. In Proceedings of the 2016 International Conference on Signal Processing and Communications (SPCOM), Bangalore, India, 12–15 June 2016; pp. 1–5.
[29] Hore, S.; Chakraborty, S.; Chatterjee, S.; Dey, N.; Ashour, A.S.;Chung, L.V.; Le, D.-N.: An integrated interactive technique for image segmentation using stack based seeded region growing and thresholding. Int. J. Electr. Comput. Eng. (IJECE)6(6), 2773–2780(2016)
[30] Abhishek M. Taori, A. K. Chaudhari, S. S. Patankar. Segmentation of macula in retinal images using automated seeding region growing technique. Inventive Computation Technologies (ICICT), International Conference on, Aug 2016, vol.2, pp.1-5
[31] Javadpour, A. and Mohammadi, A., 2016. Improving Brain Magnetic Resonance Image (MRI) Segmentation via a Novel Algorithm based on Genetic and Regional Growth. Journal of biomedical physics and engineering. 6, 95.
[32] E. Hancer, D. Karaboga, A comprehensive survey of traditional, merge-split and evolutionary approachs proposed for determination of cluster number, Swarm Evol. Comput, 32(2017) 49-67.
[33] Kelkar, D., Gupta, S., “Improved Quadtree Method for Split Merge Image Segmentation”, International Conference on Emerging Trends in Engineering and Technology, 2008, Page(s): 44 – 47.
[34] Sakamoto R, Yakami M, Fujimoto K, et al. Temporal subtraction of serial CT images with large deformation diffeomorphic metric mapping in the identification of bone metastases. Radiology 2017; 285:161942.
[35] A. Anand, S. S. Tripathy and R. S. Kumar, An improved edge detectionusing morphological Laplacian of Gaussian operator, Signal Processing and Integrated Networks (SPIN), 2015 2nd International Conference on,pp. 532-536, Feb. 2015
[36] Haple GN, Daruwala RD, Gofane MS (2015) Comparisions of Robert, Prewitt, Sobel operator-based edge detection methods for real time uses on FPGA. Int Conf Technol Sustain Dev (ICTSD), Mumbai, 1–4.doi: 10.1109/ICTSD.2015.70959
[37] 2016 International Conference on, A. Kalra, R.L. Chhokar, A hybrid approach using Sobel and Canny operator for digital image edge detection, In Micro-Electronics and Telecommunication Engineering (ICMETE), (2016), pp. 305–310 IEEE
[38] M. Nikolic, E. Tuba, and M. Tuba, “Edge detection in medical ultra-sound images using adjusted Canny edge detection algorithm,” in24thTelecommunications Forum TELFOR. IEEE, 2016, pp. 691–694.
[39] Y. Yang, S. Tong, S. Y. Huang, P. Lin, “Log-Gabor Energy Based Multimodal Medical Image Fusion in NSCT Domain,” Comput. Math. Method. M., vol. 2014, Article ID 835481, Aug. 2014.
[40] Kwon GR, Basukala D, Lee SW, Lee KH, Kang M. Brain image segmentation using a combination of expectation-maximization algorithm and watershed transform[J]. International Journal of Imaging Systems and Technology. 2016, 26(3): 225-232.
[41] Husain RA, Zayed AS, Ahmed WM, Elhaji HS. Image segmentation with improved watershed algorithm using radial bases function neural networks. In: 2015 16th International conference on sciences and techniques of automatic control and computer engineering (STA); 2015. p. 357–62. doi: 10.1109/STA.2015.7505175.
[42] E.Abdel-Maksoud,M.Elmogy,R.AlAwadi,Brain tumor segmentation based on a hybrid clustering technique, Egypt. Inform. J. 16 (1) (2015) 71–81, doi:10.1016/j.eij.2015.01.003.
[43] Funmilola A, Oke OA, Adedeji TO, Alade OM, Adewusi EA.Fuzzy K-C-means clustering algorithm for medical image seg-mentation. J Informat Eng Appl 2012;2(6):21–32.
[44] N. Dhanachandra, K. Manglem, Y.Jina Chanu, “Image Segmentation using K-means Clustering Algorithm and Subtractive Clustering Algorithm”, IMCIP-2015, Procedia Computer Science 54 ( 2015 ), pp.764 – 771.
[45] Sharma M, Purohit G and Mukherjee S. Information Retrieves from Brain MRI Images for Tumor Detection Using Hybrid Technique K-means and Artificial Neural Network (KMANN). Networking Communication and Data Knowledge Engineering. Springer, 2018, pp. 145-157.
[46] Prakash, R. M., & Kumari, R. S. S. (2017). Spatial fuzzy C means and expectation maximization algorithms with bias correction for segmentation of MR brain images. Journal of Medical Systems,41(1), 15.
[47] K.-W. Huang, Z.-Y. Zhao, Q. Gong, J. Zha, L. Chen, R. Yang, Nasopharyngeal carcinoma segmentation via HMRF-EMwith maximum entropy, in:2015 37th Annual International Conference of the IEEE Engineering in Medicine and Biology Society (EMBC), IEEE, 2015, pp. 2968–2972.
[48] Xie, S.P., Zhuang, W.Q., and Li, B.S., Blind deconvolution combined with level set method for correcting cup-ping artifacts in cone beam CT, Proc. SPIE 10133, Medical Imaging 2017: Image Processing, Florida, UnitedStates, 2017, pp. 1–4.
[49] Ian Goodfellow, et al. Deep learning. Book in preparation for MIT Press (www.deeplearningbook.org), 2016.
[50] V. Singh, A. Misra, Detection of plant leaf diseases using image segmentation and soft com-puting techniques, Information Processing in Agriculture 4 (1) (2017) 41–49.
[51] E. Iglesias and M. R. Sabuncu, ‘‘Multi-atlas segmentation of biomedical limages: A survey,’’Med. Image Anal., vol. 24, no. 1, pp. 205–219, 2015.
[52] Cabezas M, Oliver A, Llad ́o X, Freixenet J and Bach Cuadra M 2011 A review of atlas-based segmentation formagnetic resonance brain images Comput. Methods Programs Biomed.104 e158–77
[53] R. Phellan, A. Falc ̃ao, and J. Udupa, “Improving atlas-based medical image segmen-tation with a relaxed object search,” in Computational Modeling of Objects Presented in Images. Fundamentals, Methods, and Applications, 2014, vol. 8641, pp. 152–163.
[54] A. Ghaheri, S. Shoar,M. Naderan,andS. S. Hoseini," The Applications of Genetic Algorithms in Medicine", Oman Medical Journal, Vol. 30, Pages 406–416, 2015.
[55] Holzinger, K., Palade, V., Rabadan, R., Holzinger, A.: Darwin or Lamarck? Future Challenges in Evolutionary Algorithms for Knowledge Discovery and Data Mining. In: Holzinger, A., Jurisica, I. (eds.) Knowledge Discovery and Data Mining. LNCS, vol. 8401, pp. 35–56. Springer, Heidelberg (2014)
[56] Ghosha, P., Mitchellb, M., Tanyid, J.A. and Hungd, A.Y., (2016) Incorporating Priors for Medical Image Segmentation Using a Genetic Algorithm, Neurocomputing, 195,181-19