Enhancement of Data Classification Accuracy using Bagging Technique in Random Forest
Vikas S.1 , Thimmaraju S.N.2
Section:Research Paper, Product Type: Journal Paper
Volume-7 ,
Issue-8 , Page no. 185-188, Aug-2019
CrossRef-DOI: https://doi.org/10.26438/ijcse/v7i8.185188
Online published on Aug 31, 2019
Copyright © Vikas S., Thimmaraju S.N. . This is an open access article distributed under the Creative Commons Attribution License, which permits unrestricted use, distribution, and reproduction in any medium, provided the original work is properly cited.
View this paper at Google Scholar | DPI Digital Library
How to Cite this Paper
- IEEE Citation
- MLA Citation
- APA Citation
- BibTex Citation
- RIS Citation
IEEE Style Citation: Vikas S., Thimmaraju S.N., “Enhancement of Data Classification Accuracy using Bagging Technique in Random Forest,” International Journal of Computer Sciences and Engineering, Vol.7, Issue.8, pp.185-188, 2019.
MLA Style Citation: Vikas S., Thimmaraju S.N. "Enhancement of Data Classification Accuracy using Bagging Technique in Random Forest." International Journal of Computer Sciences and Engineering 7.8 (2019): 185-188.
APA Style Citation: Vikas S., Thimmaraju S.N., (2019). Enhancement of Data Classification Accuracy using Bagging Technique in Random Forest. International Journal of Computer Sciences and Engineering, 7(8), 185-188.
BibTex Style Citation:
@article{S._2019,
author = {Vikas S., Thimmaraju S.N.},
title = {Enhancement of Data Classification Accuracy using Bagging Technique in Random Forest},
journal = {International Journal of Computer Sciences and Engineering},
issue_date = {8 2019},
volume = {7},
Issue = {8},
month = {8},
year = {2019},
issn = {2347-2693},
pages = {185-188},
url = {https://www.ijcseonline.org/full_paper_view.php?paper_id=4807},
doi = {https://doi.org/10.26438/ijcse/v7i8.185188}
publisher = {IJCSE, Indore, INDIA},
}
RIS Style Citation:
TY - JOUR
DO = {https://doi.org/10.26438/ijcse/v7i8.185188}
UR - https://www.ijcseonline.org/full_paper_view.php?paper_id=4807
TI - Enhancement of Data Classification Accuracy using Bagging Technique in Random Forest
T2 - International Journal of Computer Sciences and Engineering
AU - Vikas S., Thimmaraju S.N.
PY - 2019
DA - 2019/08/31
PB - IJCSE, Indore, INDIA
SP - 185-188
IS - 8
VL - 7
SN - 2347-2693
ER -
![]() |
![]() |
![]() |
338 | 407 downloads | 189 downloads |
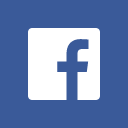
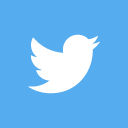
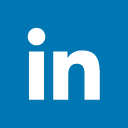
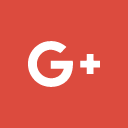
Abstract
Random forest are able to do classification on high performance through a classification ensemble with a decision trees that grow mistreatment at random elect subspaces of information. The performance of associate degree ensemble learner is very obsessed on the accuracy of every element learner and also the diversity among these parts. In random forest, organisation would cause incidence of unhealthy trees and should embrace related trees. This ends up in inappropriate and poor ensemble classification call. During this paper a shot has been created to enhance the performance of the model by applying material technique in a very random forest. Experimental results have shown that, the random forest are often more increased in terms of the classification accuracy.’
Key-Words / Index Term
Random forest, Classification Accuracy, Bagging
References
[1] Breiman, L. 2001. Random Forests. Machine Learning, Vol. 45 Issue 1, pp. 5-32.
[2] Breiman, L. 1996. Heuristics of instability and stabilization in model selection. The Annals of Statistics, Vol.24 Issue 6, pp. 2350–2383.’
[3] P. Boyle and B. Levin, "World Cancer Report,"World Health Organization, Geneva, Switzerland 2008.
[4]J. A. Cruz and D. S. Wishart, "Applications of machine learning in cancer prediction and prognosis," Cancer Informatics, vol. 2, pp. 59- 78, 2006’
[5]G. Richards, V. J. Rayward-Smith, P. Sönksen, S. Carey, and C. Weng, "Data mining for indicators of early mortality in a database of clinical records," Artificial intelligence in medicine, vol. 22, no. 3, pp. 215-231, 2001.
[6] L. Breiman, "Random forests," UC Berkeley TR567, 1999.
[7] (2016, 2016-12-10). Random Forest – RapidMiner Documentation.
[8] V Adegoke, Daqing Chen, Ebad Banissi, and Safia Barikzai. Prediction of breast cancer survivability using ensemble algorithms. 2017.
[9] Abdulsalam Alarabeyyat, Mohannad Alhanahnah, et al. Breast cancer detection using k-nearest neighbor machine learning algorithm. In Developments in eSystems Engineering (DeSE), 2016 9th International Conference on, pages 35–39. IEEE, 2016.
[10] Dania Abed Aljawad, Ebtesam Alqahtani, AL-Kuhaili Ghaidaa, Nada Qamhan, Noof Alghamdi, Saleh Alrashed, Jamal Alhiyafi, and Sunday O Olatunji. Breast cancer surgery survivability prediction using bayesian
network and support vector machines. In Informatics, Health & Technology (ICIHT), International Conference on, pages 1–6. IEEE, 2017.
[11] Bhavini J. Samajpati, Sheshang D. Degadwala,”A Survey on apple Fruit Diseases Detection and Classification” International Journal of Computer Applications (0975 –8887) Volume 130 – No.13, November 2015.
[12] Wang, Bo, et al. "Power System Transient Stability Assessment Based on Big Data and the Core Vector Machine." IEEE Transactions on Smart Grid (2016):1-1.