Performance Evaluation of Various Machine Learning Techniques for Human Activity Recognition using Smartphone
Anju S.S.1 , Kavitha K.V.2
Section:Research Paper, Product Type: Journal Paper
Volume-7 ,
Issue-8 , Page no. 316-319, Aug-2019
CrossRef-DOI: https://doi.org/10.26438/ijcse/v7i8.316319
Online published on Aug 31, 2019
Copyright © Anju S.S., Kavitha K.V. . This is an open access article distributed under the Creative Commons Attribution License, which permits unrestricted use, distribution, and reproduction in any medium, provided the original work is properly cited.
View this paper at Google Scholar | DPI Digital Library
How to Cite this Paper
- IEEE Citation
- MLA Citation
- APA Citation
- BibTex Citation
- RIS Citation
IEEE Style Citation: Anju S.S., Kavitha K.V., “Performance Evaluation of Various Machine Learning Techniques for Human Activity Recognition using Smartphone,” International Journal of Computer Sciences and Engineering, Vol.7, Issue.8, pp.316-319, 2019.
MLA Style Citation: Anju S.S., Kavitha K.V. "Performance Evaluation of Various Machine Learning Techniques for Human Activity Recognition using Smartphone." International Journal of Computer Sciences and Engineering 7.8 (2019): 316-319.
APA Style Citation: Anju S.S., Kavitha K.V., (2019). Performance Evaluation of Various Machine Learning Techniques for Human Activity Recognition using Smartphone. International Journal of Computer Sciences and Engineering, 7(8), 316-319.
BibTex Style Citation:
@article{S.S._2019,
author = {Anju S.S., Kavitha K.V.},
title = {Performance Evaluation of Various Machine Learning Techniques for Human Activity Recognition using Smartphone},
journal = {International Journal of Computer Sciences and Engineering},
issue_date = {8 2019},
volume = {7},
Issue = {8},
month = {8},
year = {2019},
issn = {2347-2693},
pages = {316-319},
url = {https://www.ijcseonline.org/full_paper_view.php?paper_id=4830},
doi = {https://doi.org/10.26438/ijcse/v7i8.316319}
publisher = {IJCSE, Indore, INDIA},
}
RIS Style Citation:
TY - JOUR
DO = {https://doi.org/10.26438/ijcse/v7i8.316319}
UR - https://www.ijcseonline.org/full_paper_view.php?paper_id=4830
TI - Performance Evaluation of Various Machine Learning Techniques for Human Activity Recognition using Smartphone
T2 - International Journal of Computer Sciences and Engineering
AU - Anju S.S., Kavitha K.V.
PY - 2019
DA - 2019/08/31
PB - IJCSE, Indore, INDIA
SP - 316-319
IS - 8
VL - 7
SN - 2347-2693
ER -
![]() |
![]() |
![]() |
731 | 340 downloads | 160 downloads |
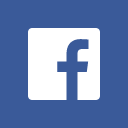
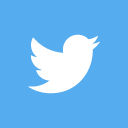
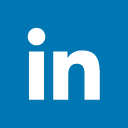
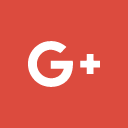
Abstract
The process of Human Activity recognition nowadays had found a wide variety of applications in healthcare and security surveillance. The commonly used smartphones are now available with inbuilt accelerometer and gyroscope sensors. The data collected using these sensors are used for recognizing the activity performed by the person who carries the smartphone. The sensor data collected from these sensors are fed to activity classifiers to train them. In this paper, the performance of various machine learning techniques are trained and evaluated for finding the better classification technique. In particular, examines the use of Decision tree, Naive bayes, K-nearest neighbour, Support Vector Machine and Random forest. The evaluation metrics used are accuracy, sensitivity, specificity and precision. During evaluation the results showed that the SVM showed better accuracy with the smartphone data.
Key-Words / Index Term
Activity Recognition, Smart phone, Accelerometer, Machine Learning, Support Vector Machines
References
[1] T.Dinh Le and C. Van Nguyen, "Human activity recognition by smartphone", 2015 2nd National Foundation for Science and Technology Development Conference on Information and Computer Science (NICS), Ho Chi Minh City, pp. 219-224, 2015.
[2] J. E. Bulbul, A. Cetin and I. A. Dogru, "Human Activity Recognition Using Smartphones", 2018 2nd International Symposium on Multidisciplinary Studies and Innovative Technologies (ISMSIT), Ankara, pp. 1-6, 2018.
[3] A. M. Khan, Y. K. Lee and S. Y. Lee, ”Human activity Recognition via An Accelerometer Enabled Smartphone Using kernel discriminent Analysis”, 2010 5th International Conference on Future Information Technology, Busan, pp. 1-6, 2010.
[4] Jindong Wang, Yiqiang Chen, Shuji Hao, Xiaohui Peng, Lisha Hu," Deep Learning for Sensor-based Activity Recognition: A Survey", Pattern Recognition Letters, pp. 1-9, 2018.
[5] M. C. Sorkun, A. E. Danisman and O. D. Incel, "Human activity recognition with mobile phone sensors: Impact of sensors and window size", 2018 26th Signal Processing and Communications Applications Conference (SIU), Izmir, pp. 1-4, 2018.
[6] K. Altun, B. Barshan, Tuncel," Comparative study on classifying human activities with miniature inertial and magnetic sensors", Pattern Recognition Letters, vol. 43, no.10, pp. 3605–3620, 2010.
[7] A. Wang, G. Chen, J. Yang, S. Zhao and C. Chang, "A Comparative Study on Human Activity Recognition Using Inertial Sensors in a Smartphone", IEEE Sensors Journal, vol. 16, no. 11, pp. 4566-4578, 2016.
[8] Meenu Shukla, Sanjiv Sharma, "Analysis of Efficient Classification Algorithm for Detection of Phishing Site", International Journal of Scientific Research in Computer Science and Engineering, Vol.5, Issue.3, pp.136-141, 2017.
[9] Davide Anguita, Alessandro Ghio, Luca Oneto, Xavier Parra and Jorge L. Reyes-Ortiz. “A Public Domain Dataset for Human Activity Recognition Using Smartphones”, 21th European Symposium on Artificial Neural Networks, Computational Intelligence and Machine Learning, ESANN 2013. Bruges, Belgium, pp. 24-26, 2013.
[10] Gagandeep Kaur, Harmanpreet Kaur, "Ensemble based J48 and random forest based C6H6 air pollution detection", International Journal of Scientific Research in Computer Science and Engineering, Vol.6, Issue.2, pp.41-50, 2018.