Quality Assessment of Crops Through Disease Detection Using Machine Learning
Vandita Mathad1 , Greeshma R.R.2 , Harshitha J.V.3 , Deepika S.4 , Snigdha Sen5
Section:Research Paper, Product Type: Journal Paper
Volume-8 ,
Issue-2 , Page no. 99-102, Feb-2020
CrossRef-DOI: https://doi.org/10.26438/ijcse/v8i2.99102
Online published on Feb 28, 2020
Copyright © Vandita Mathad, Greeshma R.R., Harshitha J.V., Deepika S., Snigdha Sen . This is an open access article distributed under the Creative Commons Attribution License, which permits unrestricted use, distribution, and reproduction in any medium, provided the original work is properly cited.
View this paper at Google Scholar | DPI Digital Library
How to Cite this Paper
- IEEE Citation
- MLA Citation
- APA Citation
- BibTex Citation
- RIS Citation
IEEE Style Citation: Vandita Mathad, Greeshma R.R., Harshitha J.V., Deepika S., Snigdha Sen, “Quality Assessment of Crops Through Disease Detection Using Machine Learning,” International Journal of Computer Sciences and Engineering, Vol.8, Issue.2, pp.99-102, 2020.
MLA Style Citation: Vandita Mathad, Greeshma R.R., Harshitha J.V., Deepika S., Snigdha Sen "Quality Assessment of Crops Through Disease Detection Using Machine Learning." International Journal of Computer Sciences and Engineering 8.2 (2020): 99-102.
APA Style Citation: Vandita Mathad, Greeshma R.R., Harshitha J.V., Deepika S., Snigdha Sen, (2020). Quality Assessment of Crops Through Disease Detection Using Machine Learning. International Journal of Computer Sciences and Engineering, 8(2), 99-102.
BibTex Style Citation:
@article{Mathad_2020,
author = {Vandita Mathad, Greeshma R.R., Harshitha J.V., Deepika S., Snigdha Sen},
title = {Quality Assessment of Crops Through Disease Detection Using Machine Learning},
journal = {International Journal of Computer Sciences and Engineering},
issue_date = {2 2020},
volume = {8},
Issue = {2},
month = {2},
year = {2020},
issn = {2347-2693},
pages = {99-102},
url = {https://www.ijcseonline.org/full_paper_view.php?paper_id=5039},
doi = {https://doi.org/10.26438/ijcse/v8i2.99102}
publisher = {IJCSE, Indore, INDIA},
}
RIS Style Citation:
TY - JOUR
DO = {https://doi.org/10.26438/ijcse/v8i2.99102}
UR - https://www.ijcseonline.org/full_paper_view.php?paper_id=5039
TI - Quality Assessment of Crops Through Disease Detection Using Machine Learning
T2 - International Journal of Computer Sciences and Engineering
AU - Vandita Mathad, Greeshma R.R., Harshitha J.V., Deepika S., Snigdha Sen
PY - 2020
DA - 2020/02/28
PB - IJCSE, Indore, INDIA
SP - 99-102
IS - 2
VL - 8
SN - 2347-2693
ER -
![]() |
![]() |
![]() |
504 | 358 downloads | 177 downloads |
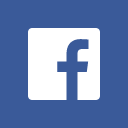
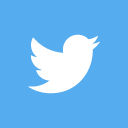
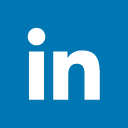
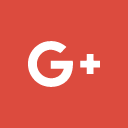
Abstract
Agriculture plays an
important role in our country, crops are considered to be vital as they are the
source of energy to mankind. Due to environmental conditions, crops are getting
affected with many diseases. Farmers are not able to detect these diseases at
an early stage. Disease in a crop leads to low productivity. Thus, assessment
of crop condition is vital. Quality assessment of crops deals with assessing
the quality and minimizing the loss of crops. It provides the fundamental
information for understanding the quality of the crops and its diseases. There
are various Machine Learning algorithms for detection and classification of
diseases. Use of machine learning algorithms like CNN not only yields better
results but it is also a cost efficient solution and it analyzes the data from
different aspects, and classifies it into one of the predefined set of classes.
In machine learning, Convolutional Neural Networks are complex feed forward
neural networks. CNNs are used for image classification and recognition because
of its high accuracy. CNN follows a hierarchical model which works on building
a network and finally gives out a fully-connected layer where all the
neurons are connected to each other and the output is processed. CNN
outperforms most of the ML algorithms when it comes to image classification provided
there are large number of images present in the dataset. The morphological
features and properties like color, intensity and dimensions of the plant
leaves are taken in to consideration for classification. Thus, detection of
disease in early stage will be beneficial for farmer so that necessary actions
can be taken.
Key-Words / Index Term
Machine Learning, Segmentation, Clustering, CNN
References
[1]
R. Poonguzhali, A.Vijayabhanu, “Crop Condition Assessment using Machine Learning”,International
Journal of Recent Technology and Engineering (IJRTE), Vol.7, Issue-6S2, April 2019.
[2]
SrdjanSladojevic, Marko Arsenovic, AndrasAnderla,
DubravkoCulibrk, and Darko Stefanovic, “Deep
Neural Networks Based Recognition of Plant Diseases by Leaf Image
Classification ”, Hindawi Publishing Corporation Computational Intelligence
and Neuroscience, Volume 2016, 29 May 2016.
[3]
Omkar Kulkarni, “Crop
Disease Detection using Deep Learning”, 2018 Fourth International Conference on Computing Communication
Control and Automation (ICCUBEA)
[4]
Nikhil Shah, SarikaJain,“Detection of Disease in Cotton Leaf using Artificial Neural Network”,
IEEE 2019
[5]
Shima Ramesh, Mr. Ramachandra Hebbar, Mr. P V Vinod, “Plant Disease Detection Using Machine
Learning”, 2018 International
Conference on Design Innovations for 3Cs Compute Communicate Control.
[6]
Radhika Deshmukh,
ManjushaDeshmukh, “Detection of Paddy
Leaf Diseases”, in: International Conference on Advances in Science and
Technology, 2015.
[7]
P. R.
Reddy, S. N. Divya, and R. Vijayalakshmi, “Plant
disease detection techniquetool—a theoretical approach,” International Journal of Innovative
Technology and Research,pp.
91–93, 2015.
[8]
P. R. Rothe and R. V. Kshirsagar, “Cotton Leaf Disease Identification using Pattern Recognition
Techniques”, International Conference onPervasive Computing (ICPC),2015.
[9]
Pasalu IC, Mishra B, Krishnaiah NV, Katti G, “Integrated pest management in rice in India:
Status and prospects”. In: Birthal PS, Sharma OP (eds) Proceedings 11.
Integrated pest management in Indian agriculture. National Centre for
Agricultural Economics and Policy Research (NCAP)/National Centre for
Integrated Pest Management (NCIPM), New Delhi, pp. 25–49,2004
[10] Singh, V., &
Misra, A. K , “Detection of plant leaf
diseases using image segmentation and soft computing techniques”.
Information processing in Agriculture, 4(1), 41-49, 2017
[11] Prajapati, H. B.,
Shah, J. P., & Dabhi, V. K , “Detection and classification of rice plant
diseases”. Intelligent Decision Technologies, 11(3), 357-373, 2017
[12] Mohan K. J,
Balasubramanian M , & Palanivel S, “Detection
and recognition of diseases from paddy plant leaf images”. International
Journal of Computer Applications, 144(12),
2016.
[13] Ferentinos, K. P, “Deep learning models for plant disease
detection and diagnosis”. Computers and Electronics in Agriculture, 145, 311-318, 2018.
[14] Zhang S, Huang W,
Zhang C,“Three-channel
convolutionalneural networks for vegetable leaf disease recognition”. Cognit
Syst Res 2019;53:31–41.
[15] Barbedo JGA, “Plant disease identification from individual
lesions and spots using deep learning”. Biosyst Eng 2019;180:96–107
[16] Liu B, Zhang Y, He D, Li Y, “Identification of apple leaf diseasesbased
on deep convolutional networks”. Symmetry 2018;10:11