A Machine Learning Approach to Predict Crop yeild and Reduction of Cost by Finding Best Accuracy
Spoorthi P1 , Jayashankara M2
Section:Research Paper, Product Type: Journal Paper
Volume-8 ,
Issue-3 , Page no. 61-66, Mar-2020
CrossRef-DOI: https://doi.org/10.26438/ijcse/v8i3.6166
Online published on Mar 30, 2020
Copyright © Spoorthi P, Jayashankara M . This is an open access article distributed under the Creative Commons Attribution License, which permits unrestricted use, distribution, and reproduction in any medium, provided the original work is properly cited.
View this paper at Google Scholar | DPI Digital Library
How to Cite this Paper
- IEEE Citation
- MLA Citation
- APA Citation
- BibTex Citation
- RIS Citation
IEEE Citation
IEEE Style Citation: Spoorthi P, Jayashankara M, “A Machine Learning Approach to Predict Crop yeild and Reduction of Cost by Finding Best Accuracy,” International Journal of Computer Sciences and Engineering, Vol.8, Issue.3, pp.61-66, 2020.
MLA Citation
MLA Style Citation: Spoorthi P, Jayashankara M "A Machine Learning Approach to Predict Crop yeild and Reduction of Cost by Finding Best Accuracy." International Journal of Computer Sciences and Engineering 8.3 (2020): 61-66.
APA Citation
APA Style Citation: Spoorthi P, Jayashankara M, (2020). A Machine Learning Approach to Predict Crop yeild and Reduction of Cost by Finding Best Accuracy. International Journal of Computer Sciences and Engineering, 8(3), 61-66.
BibTex Citation
BibTex Style Citation:
@article{P_2020,
author = {Spoorthi P, Jayashankara M},
title = {A Machine Learning Approach to Predict Crop yeild and Reduction of Cost by Finding Best Accuracy},
journal = {International Journal of Computer Sciences and Engineering},
issue_date = {3 2020},
volume = {8},
Issue = {3},
month = {3},
year = {2020},
issn = {2347-2693},
pages = {61-66},
url = {https://www.ijcseonline.org/full_paper_view.php?paper_id=5051},
doi = {https://doi.org/10.26438/ijcse/v8i3.6166}
publisher = {IJCSE, Indore, INDIA},
}
RIS Citation
RIS Style Citation:
TY - JOUR
DO = {https://doi.org/10.26438/ijcse/v8i3.6166}
UR - https://www.ijcseonline.org/full_paper_view.php?paper_id=5051
TI - A Machine Learning Approach to Predict Crop yeild and Reduction of Cost by Finding Best Accuracy
T2 - International Journal of Computer Sciences and Engineering
AU - Spoorthi P, Jayashankara M
PY - 2020
DA - 2020/03/30
PB - IJCSE, Indore, INDIA
SP - 61-66
IS - 3
VL - 8
SN - 2347-2693
ER -
![]() |
![]() |
![]() |
280 | 341 downloads | 229 downloads |
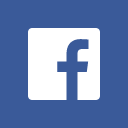
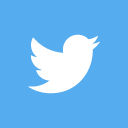
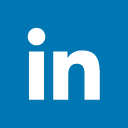
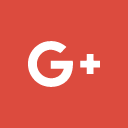
Abstract
Among
worldwide, agriculture has the major responsibility for improving the economic
contribution of the nation. However, still the most agricultural fields are
under developed due to the lack of deployment of ecosystem control
technologies. Due to these problems, the crop production is not improved which
affects the agriculture economy. Hence a development of agricultural productivity
is enhanced based on the plant yield prediction. To prevent this problem,
Agricultural sectors have to predict the crop from given dataset using machine
learning techniques. The analysis of dataset by supervised machine learning
technique(SMLT) to capture several information’s like, variable identification,
uni-variate analysis, bi-variate and multi-variate analysis, missing value
treatments etc. A comparative study
between machine learning algorithms had been carried out in order to determine
which algorithm is the most accurate in predicting the best crop. The
results show that the effectiveness of the proposed machine learning algorithm
technique can be compared with best accuracy with entropy calculation,
precision, Recall, F1 Score, Sensitivity, Specificity.
Key-Words / Index Term
Dataset,Machine learning-classification method
References
[1]Robotic Agriculture, ser. UK-RAS White Papers. UK-RAS Network,2018.
[2]Priyanka R.R., Mahesh M., Pallavi., Jayapala G., Pooja M.R.Crop protection by an alert based system using deep learning concept Research Paper|Isroset(IJSRCSE)Vol.6,Issue.6, pp47-49,Dec-2018
[3] P. Lottes, M. Hoeferlin, S. Sander, M. Muter, P. Schulze, and L. C. ¨Stachniss, “An effective classification system for separating sugar beetsand weeds for precision farming applications,” in ICRA, 2016, pp. 5157–5163.
[4] A. Milioto, P. Lottes, and C. Stachniss, “Real-time semantic segmentation of crop and weed for precision agriculture robots leveragingbackground knowledge in cnns,” in ICRA, 2018, pp. 2229–2235.
[5] P. Lottes, J. Behley, A. Milioto, and C. Stachniss,“Fully convolutionalnetworks with sequential information for robust crop and weeddetectionn precision farming,” IEEE Robotics and Automation Letters (RA-L),vol. 3, pp. 3097–3104, 2018.
[6] O. Ronneberger, P. Fischer, and T. Brox, “U-net:Convolutional networksfor biomedical image segmentation,” in International Conference onMedical image computing and computer-assisted intervention, 2015, pp.234–241.
[7] K.Simonyan and A.Zisserman,“Very deep convolutional networks forlarge-scale image recognition,” arXiv preprint arXiv:1409.1556, 2014.
[8] F.-M. De Rainville, A. Durand, F.-A. Fortin, K. Tanguy, X. Maldague,B. Panneton, and M.-J. Simard, “Bayesian classification and unsupervised learning for isolating weeds in row crops,” Pattern Analysis andApplications, vol. 17, no. 2, pp. 401–414, 2014.
[9] S. Haug, A. Michaels, P. Biber, and J. Ostermann, “Plant classificationsystem for crop/weed discrimination without segmentation,” in WACV.IEEE, 2014, pp. 1142–1149.
[10] P. Lottes, R. Khanna, J. Pfeifer, R. Siegwart, and C. Stachniss, “Uavbased crop and weed classification for smart farming,” in ICRA, 2017,pp. 3024–3031.
[11] S. H. Lee, C. S. Chan, P. Wilkin, and P. Remagnino, “Deep-plant: Plantidentification with convolutional neural networks,” in ICIP. IEEE, 2015