Data Analysis: Finding the Most Effective Factors Causing Cancer Deaths
V. Naveen Babu1 , T. Murali2 , Sk. Meer Hussian3 , S. Nava Chaitanya4 , Madda.Varalakshmi 5
Section:Technical Paper, Product Type: Journal Paper
Volume-8 ,
Issue-4 , Page no. 90-96, Apr-2020
CrossRef-DOI: https://doi.org/10.26438/ijcse/v8i4.9096
Online published on Apr 30, 2020
Copyright © V. Naveen Babu, T. Murali, Sk. Meer Hussian, S. Nava Chaitanya , Madda.Varalakshmi . This is an open access article distributed under the Creative Commons Attribution License, which permits unrestricted use, distribution, and reproduction in any medium, provided the original work is properly cited.
View this paper at Google Scholar | DPI Digital Library
How to Cite this Paper
- IEEE Citation
- MLA Citation
- APA Citation
- BibTex Citation
- RIS Citation
IEEE Style Citation: V. Naveen Babu, T. Murali, Sk. Meer Hussian, S. Nava Chaitanya , Madda.Varalakshmi, “Data Analysis: Finding the Most Effective Factors Causing Cancer Deaths,” International Journal of Computer Sciences and Engineering, Vol.8, Issue.4, pp.90-96, 2020.
MLA Style Citation: V. Naveen Babu, T. Murali, Sk. Meer Hussian, S. Nava Chaitanya , Madda.Varalakshmi "Data Analysis: Finding the Most Effective Factors Causing Cancer Deaths." International Journal of Computer Sciences and Engineering 8.4 (2020): 90-96.
APA Style Citation: V. Naveen Babu, T. Murali, Sk. Meer Hussian, S. Nava Chaitanya , Madda.Varalakshmi, (2020). Data Analysis: Finding the Most Effective Factors Causing Cancer Deaths. International Journal of Computer Sciences and Engineering, 8(4), 90-96.
BibTex Style Citation:
@article{Babu_2020,
author = {V. Naveen Babu, T. Murali, Sk. Meer Hussian, S. Nava Chaitanya , Madda.Varalakshmi},
title = {Data Analysis: Finding the Most Effective Factors Causing Cancer Deaths},
journal = {International Journal of Computer Sciences and Engineering},
issue_date = {4 2020},
volume = {8},
Issue = {4},
month = {4},
year = {2020},
issn = {2347-2693},
pages = {90-96},
url = {https://www.ijcseonline.org/full_paper_view.php?paper_id=5081},
doi = {https://doi.org/10.26438/ijcse/v8i4.9096}
publisher = {IJCSE, Indore, INDIA},
}
RIS Style Citation:
TY - JOUR
DO = {https://doi.org/10.26438/ijcse/v8i4.9096}
UR - https://www.ijcseonline.org/full_paper_view.php?paper_id=5081
TI - Data Analysis: Finding the Most Effective Factors Causing Cancer Deaths
T2 - International Journal of Computer Sciences and Engineering
AU - V. Naveen Babu, T. Murali, Sk. Meer Hussian, S. Nava Chaitanya , Madda.Varalakshmi
PY - 2020
DA - 2020/04/30
PB - IJCSE, Indore, INDIA
SP - 90-96
IS - 4
VL - 8
SN - 2347-2693
ER -
![]() |
![]() |
![]() |
227 | 311 downloads | 180 downloads |
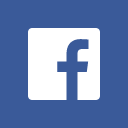
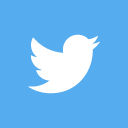
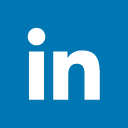
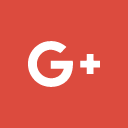
Abstract
The spreading of abnormal cells in the human body with much potential is a basic cause of disease cancer. The growth of abnormal cells may be affected by age group, being disease-oriented, or type of location in which people live and many factors. Because of the circumstances, there is no possibility of avoiding the growth of abnormal cells, but by taking corrective measures the growth can be slowed down to some extent. In addition to that, it will envisage the cancer causes which in turn can be used to create awareness among the people. In this fact, it is important to determine if someone has a high cancer risk by using biological test results which have been recorded. By working on these sample data, we can focus on finding the most influential factors that affect cancer. In this research, by applying a suitable Machine Learning algorithm on the data which have been collected using surveys, we are able to find the most important factors and mainly classification type of Machine Learning algorithms to be used for performance analysis.
Key-Words / Index Term
Data Analysis, Classification, Machine Learning, Cancer, XGBoost Algorithm
References
[1] J. Subramanian, R. Govindan, “Lung Cancer in Never Smokers”, Journal of Clinical Oncology, Vol.25(5), pp.561–570, 2007.
[2] A. Sreedevi, R. Javed, A. Dinesh, “Epidemiology of cervical cancer with special focus on India”, International Journal of Women’s Health, Vol.7, pp.405-414, 2015.
[3] K. Fernandes, D. Chicco, J.S. Cardoso, J Fernandes, “Supervised deep learning embeddings for the prediction of a cervical cancer diagnosis”, PeerJ Computer Science, Vol.4(8), p.e154, 2018.
[4] E. Roura, X. Castellsague, M. Pawlita, N. Travier, T. Waterboer, N. Margall et al., “Smoking as a major risk factor for cervical cancer and pre‐cancer: Results from the EPIC cohort”, International Journal of Cancer, Vol.135(2), pp. 453–466, 2014.
[5] V. Menon, D. Parikh, “Machine learning applied to Cervical Cancer Data”, International Journal of Scientific & Engineering Research, Vol. 9, Issue.7, pp.46-50, July-2018.
[6] S. Jayaprakash, E. Balamurugan, “A Comprehensive Survey on Data Preprocessing Methods in Web Usage Mining”, International Journal of Computer Science and Information Technologies, Vol.6 (3), pp. 3170-3174, 2015.
[7] T Chen, C Guestrin, “ XGBoost: A Scalable Tree Boosting System”, Proceedings of the 22nd ACM
SIGKDD International Conference on Knowledge Discovery and Data Mining - KDD ’16, August 13-17, 2016, San Francisco, CA, USAc©2016 ACM, ISBN 978-1-4503-4232-2/16/08.
[8] S. Zhao, Y Guo, Q. Sheng, Y. Shyr, “Advanced Heat Map and Clustering Analysis Using Heatmap3”, BioMed Research International, Vol. 2014, pp.1-6, 2014.
[9] M. Fernandes, “Data Mining: A Comparative Study of its Various Techniques and its Process”, International Journal of Scientific Research in Computer Science and Engineering, Vol.5, Issue.1, pp.19-23, February(2017).
[10] S Mahajan, "Convergence of IT and Data Mining with other technologies ", International Journal of Scientific Research in Computer Science and Engineering, Vol.01, Issue.4, pp.31-37, 2013.