A Comparative Study of Various Deep Learning Techniques Based on Automatic Image Captioning
Anurag 1 , Naresh Kumar2
Section:Review Paper, Product Type: Journal Paper
Volume-8 ,
Issue-4 , Page no. 156-160, Apr-2020
CrossRef-DOI: https://doi.org/10.26438/ijcse/v8i4.156160
Online published on Apr 30, 2020
Copyright © Anurag, Naresh Kumar . This is an open access article distributed under the Creative Commons Attribution License, which permits unrestricted use, distribution, and reproduction in any medium, provided the original work is properly cited.
View this paper at Google Scholar | DPI Digital Library
How to Cite this Paper
- IEEE Citation
- MLA Citation
- APA Citation
- BibTex Citation
- RIS Citation
IEEE Style Citation: Anurag, Naresh Kumar, “A Comparative Study of Various Deep Learning Techniques Based on Automatic Image Captioning,” International Journal of Computer Sciences and Engineering, Vol.8, Issue.4, pp.156-160, 2020.
MLA Style Citation: Anurag, Naresh Kumar "A Comparative Study of Various Deep Learning Techniques Based on Automatic Image Captioning." International Journal of Computer Sciences and Engineering 8.4 (2020): 156-160.
APA Style Citation: Anurag, Naresh Kumar, (2020). A Comparative Study of Various Deep Learning Techniques Based on Automatic Image Captioning. International Journal of Computer Sciences and Engineering, 8(4), 156-160.
BibTex Style Citation:
@article{Kumar_2020,
author = {Anurag, Naresh Kumar},
title = {A Comparative Study of Various Deep Learning Techniques Based on Automatic Image Captioning},
journal = {International Journal of Computer Sciences and Engineering},
issue_date = {4 2020},
volume = {8},
Issue = {4},
month = {4},
year = {2020},
issn = {2347-2693},
pages = {156-160},
url = {https://www.ijcseonline.org/full_paper_view.php?paper_id=5096},
doi = {https://doi.org/10.26438/ijcse/v8i4.156160}
publisher = {IJCSE, Indore, INDIA},
}
RIS Style Citation:
TY - JOUR
DO = {https://doi.org/10.26438/ijcse/v8i4.156160}
UR - https://www.ijcseonline.org/full_paper_view.php?paper_id=5096
TI - A Comparative Study of Various Deep Learning Techniques Based on Automatic Image Captioning
T2 - International Journal of Computer Sciences and Engineering
AU - Anurag, Naresh Kumar
PY - 2020
DA - 2020/04/30
PB - IJCSE, Indore, INDIA
SP - 156-160
IS - 4
VL - 8
SN - 2347-2693
ER -
![]() |
![]() |
![]() |
170 | 256 downloads | 137 downloads |
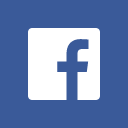
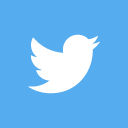
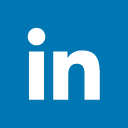
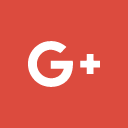
Abstract
Generating a description of an image is called image captioning. Image captioning requires recognizing the important objects, their attributes, and their relationships in an image. This process has many potential applications in real life. A noteworthy one would be to save the captions of an image so that it can be retrieved easily at a later stage just on the basis of this description. In this survey article, we aim to present a comprehensive review of existing deep-learning-based image captioning techniques. We discuss the foundation of the techniques to analyze their performances, strengths, and limitations. We also discuss the datasets and the evaluation metrics popularly used in deep-learning-based automatic image captioning.
Key-Words / Index Term
Image Captioning, Deep Learning, Encoder, Decoder
References
[1]. MD. ZAKIR HOSSAIN, FERDOUS SOHEL, MOHD FAIRUZ SHIRATUDDIN, and HAMID LAGA, “A Comprehensive Survey of Deep Learning for Image Captioning”, ACM Computing Surveys, Vol. 51, No. 6, Article 118, February 2019.
[2]. Zhihong Zeng, Xiaowen Li, “Application of human computing in image captioning under deep learning”, Springer Nature 2019, May 2019.
[3]. Xianhua Zeng, Li Wen, Banggui Liu, Xiaojun Qi, “Deep Learning for Ultrasound Image Caption Generation based on Object Detection”, Neurocomputing (2019), doi: https://doi.org/10.1016/j.neucom.2018.11.114, Nov 2018.
[4]. Christian Otto, Matthias Springstein, Avishek Anand, Ralph Ewerth, “Understanding, Categorizing and Predicting Semantic Image-Text Relations”, ICMR ’19, Ottawa, ON, Canada , June 10–13, 2019.
[5]. Xinyu Xiao, Lingfeng Wang, Kun Ding, Shiming Xiang, and Chunhong Pan, “Deep Hierarchical Encoder-Decoder Network for Image Captioning”, DOI 10.1109/TMM.2019.2915033, IEEE Transactions on Multimedia, 2019.
[6]. Yuting Su, Yuqian Li, Ning Xu, An-An Liu, “Hierarchical Deep Neural Network for Image Captioning”, Springer Science+Business Media, LLC, Springer Nature , 2019.
[7]. CHENG WANG, HAOJIN YANG, and CHRISTOPH MEINEL,” 40 Image Captioning with Deep Bidirectional LSTMs and Multi-Task Learning”, ACM Trans, Multimedia Comput. Commun., Appl. 14, 2s, Article 40, April 2018.
[8]. Xiaoxiao Liu, Qingyang Xu, Ning Wang, “A survey on deep neural network-based image captioning”, https://doi.org/10.1007/s00371-018-1566-y, Springer Nature, 2018.
[9]. Vasiliki Kougia, John Pavlopoulos, Ion Androutsopoulos, “A Survey on Biomedical Image Captioning”, arxiv:1905.13302v1, May 2019.
[10]. Peter Anderson, Xiaodong He, Chris Buehler, Damien Teney, Mark Johnson, Stephen Gould, Lei Zhang, “Bottom-Up and Top-Down Attention for Image Captioning and Visual Question Answering”, IEEE Explore, 2019.
[11]. Justin Johnson, Agrim Gupta, Li Fei-Fei, “Image Generation from Scene Graphs”, IEEE Explore, 2019.
[12]. Yang Feng, Lin Ma, Wei Liu, Jiebo Luo, “Unsupervised Image Captioning”, IEEE Explore, 2019.
[13]. Songtao Ding , Shiru Qu , Yuling Xi , Arun Kumar Sangaiah , Shaohua Wan, “ Image caption generation with high-level image features”, Pattern Recognition Letters 123 (2019) 89–95, Mar 2019.
[14]. Lin Ma, Wenhao Jiang, Zequn Jie, Yu-Gang Jiang, and Wei Liu, “Matching Image and Sentence with Multi-faceted Representations”, DOI 10.1109/TCSVT.2019.2916167, IEEE Transactions on Circuits and Systems for Video Technology, 2019.
[15]. Lun Huang, Wenmin Wang, Gang Wang, “IMAGE CAPTIONING WITH TWO CASCADED AGENTS”, ICASSP 2019, IEEE, 978-1-5386-4658-8/18, 2019.
[16]. Alexander G Schwing Jyoti Aneja, Aditya Deshpande. 2018. Convolutional image captioning. In Proceedings of the IEEE Conference on Computer Vision and Pattern Recognition. 5561–5570.
[17]. Peter Anderson, Basura Fernando, Mark Johnson, and Stephen Gould. 2016. Spice: Semantic propositional image caption evaluation. In European Conference on Computer Vision. Springer, 382–398.
[18]. Xinlei Chen and C Lawrence Zitnick. 2015. Mind’s eye: A recurrent visual representation for image caption generation. In Proceedings of the IEEE conference on computer vision and pattern recognition. 2422–2431.
[19]. Kyunghyun Cho, Bart Van Merriënboer, Dzmitry Bahdanau, and Yoshua Bengio. 2014. On the properties of neural machine translation: Encoder-decoder approaches. In Association for Computational Linguistics. 103–111.
[20]. Junyoung Chung, Caglar Gulcehre, KyungHyun Cho, and Yoshua Bengio. 2014. Empirical evaluation of gated recurrent neural networks on sequence modeling. arXiv preprint arXiv:1412.3555.