Review of Time Series based Anomaly Detection Techniques
Raghavendran R.1 , Chaitra B.H.2
Section:Research Paper, Product Type: Journal Paper
Volume-8 ,
Issue-5 , Page no. 94-95, May-2020
CrossRef-DOI: https://doi.org/10.26438/ijcse/v8i5.9495
Online published on May 30, 2020
Copyright © Raghavendran R., Chaitra B.H. . This is an open access article distributed under the Creative Commons Attribution License, which permits unrestricted use, distribution, and reproduction in any medium, provided the original work is properly cited.
View this paper at Google Scholar | DPI Digital Library
How to Cite this Paper
- IEEE Citation
- MLA Citation
- APA Citation
- BibTex Citation
- RIS Citation
IEEE Citation
IEEE Style Citation: Raghavendran R., Chaitra B.H., “Review of Time Series based Anomaly Detection Techniques,” International Journal of Computer Sciences and Engineering, Vol.8, Issue.5, pp.94-95, 2020.
MLA Citation
MLA Style Citation: Raghavendran R., Chaitra B.H. "Review of Time Series based Anomaly Detection Techniques." International Journal of Computer Sciences and Engineering 8.5 (2020): 94-95.
APA Citation
APA Style Citation: Raghavendran R., Chaitra B.H., (2020). Review of Time Series based Anomaly Detection Techniques. International Journal of Computer Sciences and Engineering, 8(5), 94-95.
BibTex Citation
BibTex Style Citation:
@article{R._2020,
author = {Raghavendran R., Chaitra B.H.},
title = {Review of Time Series based Anomaly Detection Techniques},
journal = {International Journal of Computer Sciences and Engineering},
issue_date = {5 2020},
volume = {8},
Issue = {5},
month = {5},
year = {2020},
issn = {2347-2693},
pages = {94-95},
url = {https://www.ijcseonline.org/full_paper_view.php?paper_id=5115},
doi = {https://doi.org/10.26438/ijcse/v8i5.9495}
publisher = {IJCSE, Indore, INDIA},
}
RIS Citation
RIS Style Citation:
TY - JOUR
DO = {https://doi.org/10.26438/ijcse/v8i5.9495}
UR - https://www.ijcseonline.org/full_paper_view.php?paper_id=5115
TI - Review of Time Series based Anomaly Detection Techniques
T2 - International Journal of Computer Sciences and Engineering
AU - Raghavendran R., Chaitra B.H.
PY - 2020
DA - 2020/05/30
PB - IJCSE, Indore, INDIA
SP - 94-95
IS - 5
VL - 8
SN - 2347-2693
ER -
![]() |
![]() |
![]() |
313 | 388 downloads | 244 downloads |
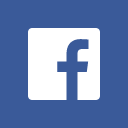
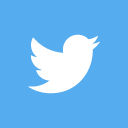
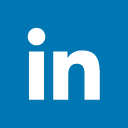
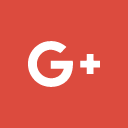
Abstract
Time series comprise a big portion of real-world data. Time series analysis is one of the most useful tools for researchers and developers. Anomaly detection is one of the key areas of focus in time series. This paper aims to provide a concise and cogent review of the existing research on the topic. In this paper, some widely accepted views and definitions of time series and anomalies are presented. Then, the various methods of anomaly detection in time series are presented, outlining their underlying mechanism, essential features, advantages and drawbacks. Finally, some common trends and observations are summarized, along with a look on the future directions of the research
Key-Words / Index Term
Time series; anomaly detection; multivariate time series; deep learning; statistics
References
[1] Gómez, J.A., Jaraiz, M.D., Vega, M.A. and Sánchez, J.M. (2008). Optimization of Time Series Using Parallel, Adaptive, and Neural Techniques. In Optimization Techniques for Solving Complex Problems (eds A.Y. Zomaya, E. Alba, C. Blum, P. Isasi, C. León and J.A. Gómez). doi:10.1002/9780470411353.ch8
[2] A. Hosseini and M. Sarrafzadeh, "Unsupervised Prediction of Negative Health Events Ahead of Time," 2019 IEEE EMBS International Conference on Biomedical & Health Informatics (BHI), Chicago, IL, USA, 2019, pp. 1-4.
[3] K. Golmohammadi and O. R. Zaiane, "Time series contextual anomaly detection for detecting market manipulation in stock market," 2015 IEEE International Conference on Data Science and Advanced Analytics (DSAA), Paris, 2015, pp. 1-10.
[4] Z. Ferdousi and A. Maeda, "Unsupervised Outlier Detection in Time Series Data," 22nd International Conference on Data Engineering Workshops (ICDEW`06), Atlanta, GA, USA, 2006, pp. x121-x121.
[5] L. Wei, E. Keogh and X. Xi, "SAXually Explicit Images: Finding Unusual Shapes," Sixth International Conference on Data Mining (ICDM`06), Hong Kong, 2006, pp. 711-720.
[6] H. Wu, "A survey of research on anomaly detection for time series," 2016 13th International Computer Conference on Wavelet Active Media Technology and Information Processing (ICCWAMTIP), Chengdu, 2016, pp. 426-431.
[7] V. l. Gorokhov and D. V. Kholodnyak, "Nonparametric statistics in multivariate time series for cognitive anomaly detection," 2016 XIX IEEE International Conference on Soft Computing and Measurements (SCM), St. Petersburg, 2016, pp. 435-436.
[8] Whitehead. B, Hoyt. W. A, “Function approximation approach to anomaly detection in propulsion system test data”, Journal of Propulsion and Power, vol.11, no.5, pp. 1074-1076, May, 2015.
[9] Hawkins D.M. (1980) Introduction. In: Identification of Outliers. Monographs on Applied Probability and Statistics. Springer, Dordrecht
[10] Ruts. I, Rousseeuw.P, “Computing depth contours of bivariate point clouds”, Computational statistics and data analysis, Vol.23, No.1, pp.153-168, Jan, 1996
[11] Diheng Qu et al., "Statistical anomaly detection for link-state routing protocols," Proceedings Sixth International Conference on Network Protocols (Cat. No.98TB100256), Austin, TX, USA, 1998, pp. 62-70.
[12] Knorr. E. M, Ng. R. T, “A unified notion of outliers: properties and computation”, Proceeding of the 3rd international conference on knowledge discovery and
data mining, Newport Beach, pp.219-222, Aug, 1997.
[13] Ramaswamy. S, Rastogi. R, Shim. K, “Efficient algorithms for mining outliers from large data sets”, Proceeding of the 2000 ACM SIGMOD international conference on management of data, New York, pp. 427-438, Jul, 2000.
[14] Dazhuo Zhou, “Clustering, similarity search and outlier detection in multivariate time series”, Tianjing University, Tianjing, 2008.
[15] Breunig, Markus M., et al. "LOF: identifying density-based local outliers." Proceedings of the 2000 ACM SIGMOD international conference on Management of data. 2000.
[16] Agyemang. M, Ezeife. C. I, “Large scale Mine. Algorithm for mining local outliers”, Proceeding of the 15th information resource management association international conference, New Orleans, pp.5-8, Apr, 2004.
[17] Papadimitriou. S, Kitagawa. H, Gibbons. P. B, “LOCI fast outlier detection using the local correlation ingral”, Technical report, pp.2-9, 2002.
[18] Hardin. J, Rocke. D. M, “Outlier detection in the multiple cluster Setting using the minimum covariance determinant estimator”, Computational Statistics and Data Analysis, Vol.44, No.4, pp.625-638, Apr, 2004.
[19] A. Jerome, T. Ishii and H. Chen, "Forecasting and Anomaly Detection on Application Metrics using LSTM," 2018 IEEE International Conference on Big Data (Big Data), Seattle, WA, USA, 2018, pp. 2221-2227.
[20] S. Hochreiter and J. Schmidhuber. “Long Short-Term Memory,” Neural Computation 9, 8 November 1997, 1735-1780.
[21] J. Shi, G. He and X. Liu, "Anomaly Detection for Key Performance Indicators Through Machine Learning," 2018 International Conference on Network Infrastructure and Digital Content (IC-NIDC), Guiyang, 2018, pp. 1-5.
[22] C. Feng, T. Li and D. Chana, "Multi-level Anomaly Detection in Industrial Control Systems via Package Signatures and LSTM Networks," 2017 47th Annual IEEE/IFIP International Conference on Dependable Systems and Networks (DSN), Denver, CO, 2017, pp. 261-272.
[23] T. Ergen and M. Kerpiççi, "A novel anomaly detection approach based on neural networks," 2018 26th Signal Processing and Communications Applications Conference (SIU), Izmir, 2018, pp. 1-4.
[24] J. Ma and S. Perkins, "Time-series novelty detection using one-class support vector machines," Proceedings of the International Joint Conference on Neural Networks, 2003., Portland, OR, 2003, pp. 1741-1745 vol.3.
[25] R. Madan and P. S. Mangipudi, "Predicting Computer Network Traffic: A Time Series Forecasting Approach Using DWT, ARIMA and RNN," 2018 Eleventh International Conference on Contemporary Computing (IC3), Noida, 2018, pp. 1-5.
[26] A. Nanduri and L. Sherry, "Anomaly detection in aircraft data using Recurrent Neural Networks (RNN)," 2016 Integrated Communications Navigation and Surveillance (ICNS), Herndon, VA, 2016, pp. 5C2-1-5C2-8.
[27] Asoke K. Nandi; Hosameldin Ahmed, "Decision Trees and Random Forests," in Condition Monitoring with Vibration Signals: Compressive Sampling and Learning Algorithms for Rotating Machines , IEEE, 2019, pp.199-224
[28] C. Pelletier, S. Valero, J. Inglada, G. Dedieu and N. Champion, "New iterative learning strategy to improve classification systems by using outlier detection techniques," 2017 IEEE International Geoscience and Remote Sensing Symposium (IGARSS), Fort Worth, TX, 2017, pp. 3676-3679.
[29] T. Radivilova, L. Kirichenko, D. Ageiev and V. Bulakh, "Classification Methods of Machine Learning to Detect DDoS Attacks," 2019 10th IEEE International Conference on Intelligent Data Acquisition and Advanced Computing Systems: Technology and Applications (IDAACS), Metz, France, 2019, pp. 207-210
[30] S. D. D. Anton, S. Sinha and H. Dieter Schotten, "Anomaly-based Intrusion Detection in Industrial Data with SVM and Random Forests," 2019 International Conference on Software, Telecommunications and Computer Networks (SoftCOM), Split, Croatia, 2019, pp. 1-6.
[31] P. Yang and B. Huang, "KNN Based Outlier Detection Algorithm in Large Dataset," 2008 International Workshop on Education Technology and Training & 2008 International Workshop on Geoscience and Remote Sensing, Shanghai, 2008, pp. 611-613.
[32] T. T. Dang, H. Y. T. Ngan and W. Liu, "Distance-based k-nearest neighbors outlier detection method in large-scale traffic data," 2015 IEEE International Conference on Digital Signal Processing (DSP), Singapore, 2015, pp. 507-510.
[33] Asniar and K. Surendro, "Using data science for detecting outliers with k Nearest Neighbors graph," 2014 International Conference on ICT For Smart Society (ICISS), Bandung, 2014, pp. 300-304.
[34] L. Cai, N. F. Thornhill, S. Kuenzel and B. C. Pal, "Real-Time Detection of Power System Disturbances Based on k -Nearest Neighbor Analysis," in IEEE Access, vol. 5, pp. 5631-5639, 2017.
[35] D. Liu, J. Pang, B. Xu, Z. Liu, J. Zhou and G. Zhang, "Satellite Telemetry Data Anomaly Detection with Hybrid Similarity Measures," 2017 International Conference on Sensing, Diagnostics, Prognostics, and Control (SDPC), Shanghai, 2017, pp. 591-596.
[36] PC Mahalanobis, “On the generalised distance in statistics”, Proceedings of the National Institute of Sciences of India, 2, 49-55, 1936
[37] R. De Maesschalck, D. Jouan-Rimbaud, D.L. Massart, “The Mahalanobis distance”, Chemometrics and Intelligent Laboratory Systems, Volume 50, Issue 1, 2000, Pages 1-18, ISSN 0169-7439
[38] A. Abid, A. Kachouri, A. Ben Fradj Guiloufi, A. Mahfoudhi, N. Nasri and M. Abid, "Centralized KNN anomaly detector for WSN," 2015 IEEE 12th International Multi-Conference on Systems, Signals & Devices (SSD15), Mahdia, 2015, pp. 1-4.
[39] J. Kao and J. Jiang, "Anomaly Detection for Univariate Time Series with Statistics and Deep Learning," 2019 IEEE Eurasia Conference on IOT, Communication and Engineering (ECICE), Yunlin, Taiwan, 2019, pp. 404-407.
[40] T. Kieu, B. Yang and C. S. Jensen, "Outlier Detection for Multidimensional Time Series Using Deep Neural Networks," 2018 19th IEEE International Conference on Mobile Data Management (MDM), Aalborg, 2018, pp. 125-134.
[41] M.A. Kramer, “Nonlinear principal component analysis using autoassociative neural networks”, AIChE Journal, 1991, 37: 233-243. doi:10.1002/aic.690370209
[42] M. Munir, S. A. Siddiqui, A. Dengel and S. Ahmed, "DeepAnT: A Deep Learning Approach for Unsupervised Anomaly Detection in Time Series," in IEEE Access, vol. 7, pp. 1991-2005, 2019.
[43] Chandola. V, “Anomaly detection for symbolic sequences and time series data”, The University of Minnesota, Minnesota, 2009.
[44] Naoya. T, Takehisa. Y, "Anomaly detection from multivariate time series with sparse representation", Proceeding of IEEE International Conference on Systems, Man and Cybernetics, San Diego, pp.2651-2656, Oct, 2014.
[45] Namrata Ghuse, Pranali Pawar, Amol Potgantwar, "An Improved Approch For Fraud Detection In Health Insurance Using Data Mining Techniques," International Journal of Scientific Research in Network Security and Communication, Vol.5, Issue.3, pp.27-33, 2017
[46] R. Satraboyina, GK. Chakravarthi, "Discovery of Ranking Fraud Detection System for Mobile Apps", International Journal of Scientific Research in Computer Science and Engineering, Vol.4, Issue.4, pp.7-10, 2016
[47] Priyanka R.R., Mahesh M., Pallavi S.S., Jayapala G., Pooja M.R., "Crop Protection by an alert Based System using Deep Learning Concept", International Journal of Scientific Research in Computer Science and Engineering, Vol.6, Issue.6, pp.47-49, 2018
[48] N. SelvaKumar, M. Rohini, C. Narmada, M. Yogeshprabhu, "Network Traffic Control Using AI," International Journal of Scientific Research in Network Security and Communication, Vol.8, Issue.2, pp.13-21, 2020