Prediction of Polysemous Words in Sentiment Analysis: A Review
Manisha Malik1 , Neetu Verma2
Section:Review Paper, Product Type: Journal Paper
Volume-8 ,
Issue-5 , Page no. 100-104, May-2020
CrossRef-DOI: https://doi.org/10.26438/ijcse/v8i5.100104
Online published on May 30, 2020
Copyright © Manisha Malik, Neetu Verma . This is an open access article distributed under the Creative Commons Attribution License, which permits unrestricted use, distribution, and reproduction in any medium, provided the original work is properly cited.
View this paper at Google Scholar | DPI Digital Library
How to Cite this Paper
- IEEE Citation
- MLA Citation
- APA Citation
- BibTex Citation
- RIS Citation
IEEE Citation
IEEE Style Citation: Manisha Malik, Neetu Verma, “Prediction of Polysemous Words in Sentiment Analysis: A Review,” International Journal of Computer Sciences and Engineering, Vol.8, Issue.5, pp.100-104, 2020.
MLA Citation
MLA Style Citation: Manisha Malik, Neetu Verma "Prediction of Polysemous Words in Sentiment Analysis: A Review." International Journal of Computer Sciences and Engineering 8.5 (2020): 100-104.
APA Citation
APA Style Citation: Manisha Malik, Neetu Verma, (2020). Prediction of Polysemous Words in Sentiment Analysis: A Review. International Journal of Computer Sciences and Engineering, 8(5), 100-104.
BibTex Citation
BibTex Style Citation:
@article{Malik_2020,
author = {Manisha Malik, Neetu Verma},
title = {Prediction of Polysemous Words in Sentiment Analysis: A Review},
journal = {International Journal of Computer Sciences and Engineering},
issue_date = {5 2020},
volume = {8},
Issue = {5},
month = {5},
year = {2020},
issn = {2347-2693},
pages = {100-104},
url = {https://www.ijcseonline.org/full_paper_view.php?paper_id=5116},
doi = {https://doi.org/10.26438/ijcse/v8i5.100104}
publisher = {IJCSE, Indore, INDIA},
}
RIS Citation
RIS Style Citation:
TY - JOUR
DO = {https://doi.org/10.26438/ijcse/v8i5.100104}
UR - https://www.ijcseonline.org/full_paper_view.php?paper_id=5116
TI - Prediction of Polysemous Words in Sentiment Analysis: A Review
T2 - International Journal of Computer Sciences and Engineering
AU - Manisha Malik, Neetu Verma
PY - 2020
DA - 2020/05/30
PB - IJCSE, Indore, INDIA
SP - 100-104
IS - 5
VL - 8
SN - 2347-2693
ER -
![]() |
![]() |
![]() |
294 | 424 downloads | 216 downloads |
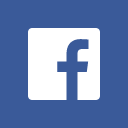
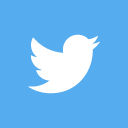
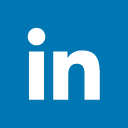
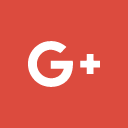
Abstract
In the last some years, new methods of communication channels have appeared and become indifferent. These communication channels are the social networking sites which have experienced an exponential growth. During the translation or communication, the problem of polysemy may cause difficulties. Therefore, there is a dire need for sentiment analysis process which can automatically extract and detect the sentiments of data extracted from micro blogging sites. It requires efficient techniques to collect a large amount of social media data and extract meaningful information from them. This paper presents a document level lexicon-based approach to detect the sentiment polarity. So, we focused on pre-processing of data. Instead of removing all polysemy, it includes some polysemous words in the complete procedure of sentiment analysis. We use specific number of polysemy words there but in future we will focus on different words and enhance the accuracy of our documentation.
Key-Words / Index Term
Sentiment Analysis, polysemy, polarity, wordnet
References
[1] Udaya Raj Dhungana1, Subarna Shakya, Kabita Baral and Bharat Sharma (2014),” Word Sense Dissambiguation using WSD specific WordNet of Polysemy Words,” in International Journal on Natural Language Computing (IJNLC).
[2] Rice, D. R., & Zorn, C. (2013). Corpus-based dictionaries for sentiment analysis of specialized vocabularies. Proceedings of NDATAD, 98-115.
[3] M. Sinha, M. K. Reddy, P. Bhattacharyya, P. Pandey, and L. Kashyap, “Hindi word sense disambiguation,” Master’s thesis, Indian Institute of Technology Bombay, Mumbai, India, 2004.
[4] U. R. Dhungana and S. Shakya, “Word sense disambiguation in nepali language,” in The Fourth International Conference on Digital Information and Communication Technology and Its Application (DICTAP2014), Bangkok, Thailand, 2014, pp. 46–50.
[5] M. E. Lesk, “Automatic sense disambiguation using machine readable dictionaries: How to tell a pine cone from an ice cream cone,” in SIGDOC Conference, Toronto, Ontario, Canada, 1986.
[6] Kaur, J. (2016). A Review Paper on Twitter Sentiment Analysis Techniques. In International Journal for Research in Applied Science & Engineering Technology (IJRASET) (Vol. 4, No. 2016).
[7] Yih-Jeng LIN, Ming-Shing YU and Chin-Yu LIN (2010), A Combined Approach to the Polysemy Problems in a Chinese to Taiwanese TTS System, National Chung-Hsing University, Taichung. IEEE.
[9] Chauhan, C., & Sehgal, S. (2017, May). Sentiment analysis on product reviews. In Computing, Communication and Automation (ICCCA), 2017 International Conference on (pp. 26-31). IEEE.
[10] Astya, P. (2017, May). Sentiment analysis: Approaches and open issues. In Computing, Communication and Automation (ICCCA), 2017 International Conference on (pp. 154-158). IEEE.
[11] Kolkur, S., Dantal, G., & Mahe, R. (2015). Study of different levels for sentiment analysis. International Journal of Current Engineering and Technology, 5(2), 768Ű770.
[12] Ram, K.S.K., Araballi, S., BR, S., Shobha, G. (2014, December). Sentiment Analysis of Twitter Data. In International Journal of Advanced Research in Computer Engineering & Technology (IJARCET) (Vol. 3 Issue 12, December 2014)
[13] Xiaohui Yu,Yang Liu, Jimmy Xiangji Huang, and Aijun An,,” Mining Online Reviews for Predicting Sales Performance: A Case Study in the Movie Domain”, IEEE Trans.On knowledge and data engineering, vol. 24, no. 4, April 2012.
[14] Bhuta, S., Doshi, A., Doshi, U., & Narvekar, M. (2014, February). A review of techniques for sentiment analysis Of Twitter data. In Issues and challenges in intelligent computing techniques (ICICT), 2014 international conference on (pp. 583-591). IEEE.
[15] Mandal, S., & Gupta, S. (2016, December). A Lexicon-based text classification model to analyse and predict sentiments from online reviews. In Computer, Electrical & Communication Engineering (ICCECE), 2016 International Conference on (pp. 1-7). IEEE.
[16] Andrey Kapitanov, Ilona Kapitanova, Vladimir Troyanovskiy, Vladimir Ilyushechkin, Ekaterina Dorogova (2019), Clustering of Word Contexts as a Method of Eliminating Polysemy of Words, National Research University of Electronic Technology, Zelenograd, Moscow, Russia, IEEE.
[17] Mehto, A., & Indras, K. (2016, March). Data mining through sentiment analysis: Lexicon based sentiment analysis model using aspect catalogue. In Colossal Data Analysis and Networking (CDAN), Symposium on (pp. 1-7). IEEE.
[18] Pang, B., & Lee, L. (2008). Opinion mining and sentiment analysis. Foundations and Trends® in Information Retrieval, 2(1–2), 1-135.
[19] Shahare, F. F. (2017, June). Sentiment analysis for the news data based on the social media. In Intelligent Computing and Control Systems (ICICCS), 2017 International Conference on (pp. 1365-1370). IEEE.
[20] Ramteke, J., Shah, S., Godhia, D., & Shaikh, A. (2016, August). Election result prediction using Twitter sentiment analysis. In Inventive Computation Technologies (ICICT), International Conference on (Vol. 1, pp. 1-5). IEEE.
[21] Jain, A. P., & Dandannavar, P. (2016, July). Application of machine learning techniques to sentiment analysis. In Applied and Theoretical Computing and Communication Technology (iCATccT), 2016 2nd International Conference on (pp. 628-632). IEEE.
[22] Bhoir, P., & Kolte, S. (2015, December). Sentiment analysis of movie reviews using lexicon approach. In Computational Intelligence and Computing Research (ICCIC), 2015 IEEE International Conference on (pp. 1-6). IEEE.