A Review of Big Data in Network Intrusion Detection System: Challenges, Approaches, Datasets, and Tools
Reem Alshamy1 , Mossa Ghurab2
Section:Review Paper, Product Type: Journal Paper
Volume-8 ,
Issue-7 , Page no. 62-75, Jul-2020
CrossRef-DOI: https://doi.org/10.26438/ijcse/v8i7.6275
Online published on Jul 31, 2020
Copyright © Reem Alshamy, Mossa Ghurab . This is an open access article distributed under the Creative Commons Attribution License, which permits unrestricted use, distribution, and reproduction in any medium, provided the original work is properly cited.
View this paper at Google Scholar | DPI Digital Library
How to Cite this Paper
- IEEE Citation
- MLA Citation
- APA Citation
- BibTex Citation
- RIS Citation
IEEE Citation
IEEE Style Citation: Reem Alshamy, Mossa Ghurab, “A Review of Big Data in Network Intrusion Detection System: Challenges, Approaches, Datasets, and Tools,” International Journal of Computer Sciences and Engineering, Vol.8, Issue.7, pp.62-75, 2020.
MLA Citation
MLA Style Citation: Reem Alshamy, Mossa Ghurab "A Review of Big Data in Network Intrusion Detection System: Challenges, Approaches, Datasets, and Tools." International Journal of Computer Sciences and Engineering 8.7 (2020): 62-75.
APA Citation
APA Style Citation: Reem Alshamy, Mossa Ghurab, (2020). A Review of Big Data in Network Intrusion Detection System: Challenges, Approaches, Datasets, and Tools. International Journal of Computer Sciences and Engineering, 8(7), 62-75.
BibTex Citation
BibTex Style Citation:
@article{Alshamy_2020,
author = {Reem Alshamy, Mossa Ghurab},
title = {A Review of Big Data in Network Intrusion Detection System: Challenges, Approaches, Datasets, and Tools},
journal = {International Journal of Computer Sciences and Engineering},
issue_date = {7 2020},
volume = {8},
Issue = {7},
month = {7},
year = {2020},
issn = {2347-2693},
pages = {62-75},
url = {https://www.ijcseonline.org/full_paper_view.php?paper_id=5167},
doi = {https://doi.org/10.26438/ijcse/v8i7.6275}
publisher = {IJCSE, Indore, INDIA},
}
RIS Citation
RIS Style Citation:
TY - JOUR
DO = {https://doi.org/10.26438/ijcse/v8i7.6275}
UR - https://www.ijcseonline.org/full_paper_view.php?paper_id=5167
TI - A Review of Big Data in Network Intrusion Detection System: Challenges, Approaches, Datasets, and Tools
T2 - International Journal of Computer Sciences and Engineering
AU - Reem Alshamy, Mossa Ghurab
PY - 2020
DA - 2020/07/31
PB - IJCSE, Indore, INDIA
SP - 62-75
IS - 7
VL - 8
SN - 2347-2693
ER -
![]() |
![]() |
![]() |
508 | 609 downloads | 213 downloads |
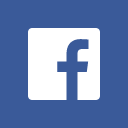
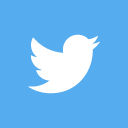
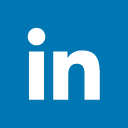
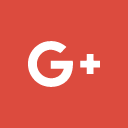
Abstract
Intrusion Detection System (IDS) is a promised research field in the cybersecurity due to the rapid development of the Internet. Many IDS employ classification algorithms for classifying network traffic, and these classification algorithms failed to achieve accurate attack detection due to the huge amount of data. However, by applying dimensional reduction, data can be efficiently reduced and achieve accurate attack detection. The main work in this paper is to provide a comprehensive review of the IDS types and methods used to detect attack, advantages and disadvantages of each type. Furthermore, the authors focus on the Network Intrusion Detection System (NIDS) type and introduce the ten characteristics of Big Data and the challenges of Big Data in NIDS. Furthermore, we analyze different approaches used in NIDS based on machine learning algorithms, for each approach we study the performance of classifiers (Binary or Multi classification) under eight datasets and dimensional reduction techniques. A comparison of some machine learning algorithms and the five tools used for analyzing Big Data are presented. Discussions came from our analysis of current research. Finally, we will finish this paper by representing conclusions and describe future work
Key-Words / Index Term
Big Data, Network Intrusion Detection System, Classification, Big Data Techniques
References
[1] D. Gaurav, J. K. P. S. Yadav, R. K. Kaliyar, and A. Goyal, "An Outline on Big Data and Big Data Analytics," pp. 74-79.
[2] R. Devakunchari, "Analysis on big data over the years," International Journal of Scientific and Research Publications, vol. 4, pp. 1-7, 2014.
[3] A. Ju, Y. Guo, Z. Ye, T. Li, and J. Ma, "HeteMSD: A Big Data Analytics Framework for Targeted Cyber-Attacks Detection Using Heterogeneous Multisource Data," Security and Communication Networks, vol. 2019, 2019.
[4] L. Wang and R. Jones, "Big data analytics for network intrusion detection: A survey," International Journal of Networks and Communications, vol. 7, pp. 24-31, 2017.
[5] S. M. Othman, N. T. Alsohybe, F. M. Ba-Alwi, and A. T. Zahary, "Survey on Intrusion Detection System Types," International Journal of Cyber-Security and Digital Forensics, vol. 7, pp. 444-463, 2018.
[6] K. Kim, M. E. Aminanto, and H. C. Tanuwidjaja, Network Intrusion Detection Using Deep Learning: A Feature Learning Approach: Springer, 2018.
[7] S. Gulghane, V. Shingate, S. Bondgulwar, G. Awari, and P. Sagar, "A Survey on Intrusion Detection System Using Machine Learning Algorithms," pp. 670-675.
[8] Y. Hamid, M. Sugumaran, and L. Journaux, "Machine learning techniques for intrusion detection: a comparative analysis," pp. 1-6.
[9] P. Dehariya, "An Artificial Immune System and Neural Network to Improve the Detection Rate in Intrusion Detection System," International Journal of Scientific Research in Network Security and Communication, vol. 4, pp. 1-4, 2016.
[10] E. Guerra, J. de Lara, A. Malizia, and P. D?az, "Supporting user-oriented analysis for multi-view domain-specific visual languages," Information and Software Technology, vol. 51, pp. 769-784, 2009.
[11] P. Adluru, S. S. Datla, and X. Zhang, "Hadoop eco system for big data security and privacy," pp. 1-6.
[12] M. Kaur and A. M. Aslam, "Big Data Analytics on IOT: Challenges, Open Research Issues and Tools," International Journal of Scientific Research in Computer Science and Engineering, vol. 6, pp. 81-85, 2018.
[13] P. Zikopoulos, D. deRoos, K. Parasuraman, T. Deutsch, D. Corrigan, J. Giles, et al., "Harness the Power of Big Data?The IBM Big Data Platform. 2011," www-01. ibm. com/software/data/bigdata (letzter Zugriff am 31.03. 2018), 2011.
[14] R. Zuech, T. M. Khoshgoftaar, and R. Wald, "Intrusion detection and big heterogeneous data: a survey," Journal of Big Data, vol. 2, pp. 3-3, 2015.
[15] Z. Sun, "10 Bigs: Big data and its ten big characteristics," PNG UoT BAIS, vol. 3, pp. 1-10, 2018.
[16] N. Khan, M. Alsaqer, H. Shah, G. Badsha, A. A. Abbasi, and S. Salehian, "The 10 Vs, issues and challenges of big data," pp. 52-56, 2018.
[17] C. Zouhair, N. Abghour, K. Moussaid, A. El Omri, and M. Rida, "A Review of Intrusion Detection Systems in Cloud Computing," ed: IGI Global, , pp. 253-283, 2018.
[18] K. Siddique, Z. Akhtar, M. A. Khan, Y.-H. Jung, and Y. Kim, "Developing an Intrusion Detection Framework for High-Speed Big Data Networks: A Comprehensive Approach," KSII Transactions on Internet & Information Systems, vol. 12, 2018.
[19] F. A. B. H. Ali and Y. Y. Len, "Development of host based intrusion detection system for log files," pp. 281-285.
[20] A. K. Saxena, S. Sinha, and P. Shukla, "General study of intrusion detection system and survey of agent based intrusion detection system," pp. 421-471.
[21] M. Liu, Z. Xue, X. Xu, C. Zhong, and J. Chen, "Host-based intrusion detection system with system calls: Review and future trends," ACM Computing Surveys (CSUR), vol. 51, pp. 1-36, 2018.
[22] H.-J. Liao, C.-H. R. Lin, Y.-C. Lin, and K.-Y. Tung, "Intrusion detection system: A comprehensive review," Journal of Network and Computer Applications, vol. 36, pp. 16-24, 2013.
[23] L. Wang, "Big Data in intrusion detection systems and intrusion prevention systems," J Comput Netw, vol. 4, pp. 48-55, 2017.
[24] J. Frank, "Artificial intelligence and intrusion detection: Current and future directions," pp. 1-12.
[25] M. Belouch, S. El Hadaj, and M. Idhammad, "Performance evaluation of intrusion detection based on machine learning using Apache Spark," Procedia Computer Science, vol. 127, pp. 1-6, 2018.
[26] O. Faker and E. Dogdu, "Intrusion detection using big data and deep learning techniques," pp. 86-93.
[27] R. Chapaneri and S. Shah, "A comprehensive survey of machine learning-based network intrusion detection," ed: Springer, 2019, pp. 345-356.
[28] R. Patel, A. Thakkar, and A. Ganatra, "A survey and comparative analysis of data mining techniques for network intrusion detection systems," International Journal of Soft Computing and Engineering (IJSCE), vol. 2, pp. 260-265, 2012.
[29] S. Suthaharan, "A single-domain, representation-learning model for big data classification of network intrusion," pp. 296-310.
[30] P. Singh, S. Krishnamoorthy, A. Nayyar, A. K. Luhach, and A. Kaur, "Soft-computing-based false alarm reduction for hierarchical data of intrusion detection system," International Journal of Distributed Sensor Networks, vol. 15, 2019.
[31] K. K. Wankhade and K. C. Jondhale, "An ensemble clustering method for intrusion detection," International Journal of Intelligent Engineering Informatics, vol. 7, pp. 112-140, 2019.
[32] M. U. Farooq, H. Xiaoli, and S. A. Rauf, "Big Data Security Analysis in Network Intrusion Detection System," International Journal of Computer Applications, vol. 975, pp. 8887-8887, 2020.
[33] L. Lv, W. Wang, Z. Zhang, and X. Liu, "A novel intrusion detection system based on an optimal hybrid kernel extreme learning machine," Knowledge-Based Systems, pp. 105648-105648, 2020.
[34] N. Hariyale, M. S. Rathore, R. Prasad, and P. Saurabh, "A Hybrid Approach for Intrusion Detection System," ed: Springer, 2020, pp. 391-403.
[35] W. Fang, X. Tan, and D. Wilbur, "Application of intrusion detection technology in network safety based on machine learning," Safety Science, vol. 124, pp. 104604-104604, 2020.
[36] J. Ghasemi, J. Esmaily, and R. Moradinezhad, "Intrusion detection system using an optimized kernel extreme learning machine and efficient features," S?dhan?, vol. 45, pp. 1-9, 2020.
[37] A. Kumar, W. Glisson, and H. Cho, "Network Attack Detection using an Unsupervised Machine Learning Algorithm."
[38] D. Proti? and M. Stankovi?, "Detection of Anomalies in the Computer Network Behaviour," European Journal of Engineering and Formal Sciences, vol. 4, pp. 7-13, 2020.
[39] S. Krishnaveni, P. Vigneshwar, S. Kishore, B. Jothi, and S. Sivamohan, "Anomaly-Based Intrusion Detection System Using Support Vector Machine," ed: Springer, 2020, pp. 723-731.
[40] V. Kumar, A. K. Das, and D. Sinha, "Statistical analysis of the UNSW-NB15 dataset for intrusion detection," ed: Springer, 2020, pp. 279-294.
[41] K. V. Krishna, K. Swathi, and B. B. Rao, "A Novel Framework for NIDS through Fast kNN Classifier on CICIDS2017 Dataset," 2020.
[42] G. Karatas, O. Demir, and O. K. Sahingoz, "Increasing the Performance of Machine Learning-Based IDSs on an Imbalanced and Up-to-Date Dataset," IEEE Access, vol. 8, pp. 32150-32162, 2020.
[43] I. Obeidat, N. Hamadneh, M. Alkasassbeh, M. Almseidin, and M. AlZubi, "Intensive pre-processing of kdd cup 99 for network intrusion classification using machine learning techniques," 2019.
[44] D. A. Kumar and S. R. Venugopalan, "A design of a parallel network anomaly detection algorithm based on classification," International Journal of Information Technology, pp. 1-14, 2019.
[45] K. Ye, "Key feature recognition algorithm of network intrusion signal based on neural network and support vector machine," Symmetry, vol. 11, pp. 380-380, 2019.
[46] N. Kaja, A. Shaout, and D. Ma, "An intelligent intrusion detection system," Applied Intelligence, vol. 49, pp. 3235-3247, 2019.
[47] B. S. Bhati and C. S. Rai, "Analysis of Support Vector Machine-based Intrusion Detection Techniques," Arabian Journal for Science and Engineering, pp. 1-13, 2019.
[48] K. A. Taher, B. M. Y. Jisan, and M. M. Rahman, "Network intrusion detection using supervised machine learning technique with feature selection," pp. 643-646.
[49] M. R. G. Raman, N. Somu, S. Jagarapu, T. Manghnani, T. Selvam, K. Krithivasan, et al., "An efficient intrusion detection technique based on support vector machine and improved binary gravitational search algorithm," Artificial Intelligence Review, pp. 1-32, 2019.
[50] M. Nawir, A. Amir, N. Yaakob, A. R. Badlishah, A. M. Safar, M. N. M. Warip, et al., "Distributed Online Averaged One Dependence Estimator (DOAODE) Algorithm for Multi-class Classification of Network Anomaly Detection System," pp. 12015-12015.
[51] M. Alrowaily, F. Alenezi, and Z. Lu, "Effectiveness of machine learning based intrusion detection systems," pp. 277-288.
[52] V. Kanimozhi and T. P. Jacob, "Artificial Intelligence based Network Intrusion Detection with hyper-parameter optimization tuning on the realistic cyber dataset CSE-CIC-IDS2018 using cloud computing," pp. 33-36.
[53] S. M. Othman, F. M. Ba-Alwi, N. T. Alsohybe, and A. Y. Al-Hashida, "Intrusion detection model using machine learning algorithm on Big Data environment," Journal of Big Data, vol. 5, pp. 34-34, 2018.
[54] K. Peng, V. Leung, L. Zheng, S. Wang, C. Huang, and T. Lin, "Intrusion detection system based on decision tree over big data in fog environment," Wireless Communications and Mobile Computing, vol. 2018, 2018.
[55] E. M. Kurt and Y. Becerikli, "Network Intrusion Detection on Apache Spark with Machine Learning Algorithms," pp. 130-141.
[56] F. Karata? and S. A. Korkmaz, "Big Data: controlling fraud by using machine learning libraries on Spark," International Journal of Applied Mathematics Electronics and Computers, vol. 6, pp. 1-5, 2018.
[57] K. Peng, V. C. M. Leung, and Q. Huang, "Clustering approach based on mini batch kmeans for intrusion detection system over big data," IEEE Access, vol. 6, pp. 11897-11906, 2018.
[58] S. Aljawarneh, M. Aldwairi, and M. B. Yassein, "Anomaly-based intrusion detection system through feature selection analysis and building hybrid efficient model," Journal of Computational Science, vol. 25, pp. 152-160, 2018.
[59] S. K. Biswas, "Intrusion detection using machine learning: A comparison study," International Journal of Pure and Applied Mathematics, vol. 118, pp. 101-114, 2018.
[60] B. N. Kumar, M. S. V. S. B. Raju, and B. V. Vardhan, "Enhancing the performance of an intrusion detection system through multi-linear dimensionality reduction and Multi-class SVM," International Journal of Intelligent Engineering and Systems, vol. 11, pp. 181-192, 2018.
[61] P. Dahiya and D. K. Srivastava, "Network intrusion detection in big dataset using Spark," Procedia Computer Science, vol. 132, pp. 253-262, 2018.
[62] T. Aldwairi, D. Perera, and M. A. Novotny, "An evaluation of the performance of Restricted Boltzmann Machines as a model for anomaly network intrusion detection," Computer Networks, vol. 144, pp. 111-119, 2018.
[63] A. Verma and V. Ranga, "Statistical analysis of CIDDS-001 dataset for network intrusion detection systems using distance-based machine learning," Procedia Computer Science, vol. 125, pp. 709-716, 2018.
[64] H. Zhang, S. Dai, Y. Li, and W. Zhang, "Real-time Distributed-Random-Forest-Based Network Intrusion Detection System Using Apache Spark," pp. 1-7.
[65] J. Maharani and Z. Rustam, "The Application of Multi-Class Support Vector Machines on Intrusion Detection System with the Feature Selection using Information Gain."
[66] H. Wang, Y. Xiao, and Y. Long, "Research of intrusion detection algorithm based on parallel SVM on spark," pp. 153-156.
[67] M. A. Manzoor and Y. Morgan, "Network intrusion detection system using apache storm," Probe, vol. 4107, pp. 4166-4166, 2017.
[68] M. C. Belavagi and B. Muniyal, "Multi Class Machine Learning Algorithms for Intrusion Detection-A Performance Study," pp. 170-178.
[69] I. S. Thaseen and C. A. Kumar, "Intrusion detection model using fusion of chi-square feature selection and multi class SVM," Journal of King Saud University-Computer and Information Sciences, vol. 29, pp. 462-472, 2017.
[70] M. Tavallaee, E. Bagheri, W. Lu, and A. A. Ghorbani, "A detailed analysis of the KDD CUP 99 data set IEEE Symp," Comput. Intell. Secur. Def. Appl. CISDA 2009, no. Cisda, pp. 1-6, 2009.
[71] M. K. Siddiqui and S. Naahid, "Analysis of KDD CUP 99 dataset using clustering based data mining," International Journal of Database Theory and Application, vol. 6, pp. 23-34, 2013.
[72] M. Ring, S. Wunderlich, D. Scheuring, D. Landes, and A. Hotho, "A survey of network-based intrusion detection data sets," Computers & Security, 2019.
[73] P. Kar, S. Banerjee, K. C. Mondal, G. Mahapatra, and S. Chattopadhyay, "A Hybrid Intrusion Detection System for Hierarchical Filtration of Anomalies," ed: Springer, 2019, pp. 417-426.
[74] C. Azad, A. K. Mehta, and V. K. Jha, "Evolutionary Decision Tree-Based Intrusion Detection System," pp. 271-282.
[75] T. Ahmad and M. N. Aziz, "Data Preprocessing and Feature Selection for Machine Learning Intrusion Detection Systems," ICIC Express Letter, vol. 13, pp. 93-101, 2019.
[76] J.-h. Woo, J.-Y. Song, and Y.-J. Choi, "Performance Enhancement of Deep Neural Network Using Feature Selection and Preprocessing for Intrusion Detection," pp. 415-417.
[77] S. Khalid, T. Khalil, and S. Nasreen, "A survey of feature selection and feature extraction techniques in machine learning," pp. 372-378.
[78] Z. M. Hira and D. F. Gillies, "A review of feature selection and feature extraction methods applied on microarray data," Advances in bioinformatics, vol. 2015, 2015.
[79] A. Subasi, Practical Guide for Biomedical Signals Analysis Using Machine Learning Techniques: Academic Press, 2019.
[80] H. Motoda and H. Liu, "Feature selection, extraction and construction," Communication of IICM (Institute of Information and Computing Machinery, Taiwan) Vol, vol. 5, pp. 2-2, 2002.
[81] A. A. Aburomman and M. B. I. Reaz, "Ensemble of binary SVM classifiers based on PCA and LDA feature extraction for intrusion detection," pp. 636-640.
[82] G. Karatas, O. Demir, and O. K. Sahingoz, "Deep learning in intrusion detection systems," pp. 113-116.
[83] J. Miao and L. Niu, "A survey on feature selection," Procedia Computer Science, vol. 91, pp. 919-926, 2016.
[84] M. Ziaye, S. Khalid, and Y. Mehmood, "Survey of Feature Selection/Extraction Methods used in Biomedical Imaging," International Journal of Computer Science and Information Security (IJCSIS), vol. 16, 2018.
[85] L. Ladha and T. Deepa, "Feature selection methods and algorithms," International journal on computer science and engineering, vol. 3, pp. 1787-1797, 2011.
[86] P. Kumbhar and M. Mali, "A survey on feature selection techniques and classification algorithms for efficient text classification," International Journal of Science and Research, vol. 5, pp. 9-9, 2016.
[87] B. Sahu, S. Dehuri, and A. Jagadev, "A Study on the Relevance of Feature Selection Methods in Microarray Data," The Open Bioinformatics Journal, vol. 11, 2018.
[88] I. Guyon, J. Weston, S. Barnhill, and V. Vapnik, "Gene selection for cancer classification using support vector machines," Machine learning, vol. 46, pp. 389-422, 2002.
[89] M. Abdulrazaq and A. Salih, "Combination of multi classification algorithms for intrusion detection system," Int. J. Sci. Eng. Res., vol. 6, pp. 1364-1371, 2015.
[90] D. S. Kim and J. S. Park, "Network-based intrusion detection with support vector machines," pp. 747-756.
[91] M. Praveena and V. Jaiganesh, "A literature review on supervised machine learning algorithms and boosting process," International Journal of Computer Applications, vol. 169, pp. 32-35, 2017.
[92] M. Aly, "Survey on multiclass classification methods," Neural Netw, vol. 19, pp. 1-9, 2005.
[93] M. Topczewska, "Multiclass classification strategy based on dipoles," Zeszyty Naukowe Politechniki Bia?ostockiej. Informatyka, pp. 79-90, 2011.
[94] S. A. Mulay, P. R. Devale, and G. V. Garje, "Intrusion detection system using support vector machine and decision tree," International Journal of Computer Applications, vol. 3, pp. 40-43, 2010.
[95] I. A. Solomon, A. Jatain, and S. B. Bajaj, "Neural Network Based Intrusion Detection: State of the Art," Available at SSRN 3356505, 2019.
[96] S. Ewen, S. Schelter, K. Tzoumas, D. Warneke, and V. Markl, "Iterative parallel data processing with stratosphere: an inside look," pp. 1053-1056.
[97] S. Landset, T. M. Khoshgoftaar, A. N. Richter, and T. Hasanin, "A survey of open source tools for machine learning with big data in the Hadoop ecosystem," Journal of Big Data, vol. 2, pp. 24-24, 2015.
[98] J. Dean and S. Ghemawat, "MapReduce: Simplified data processing on large clusters," 2004.
[99] V. K. Vavilapalli, A. C. Murthy, C. Douglas, S. Agarwal, M. Konar, R. Evans, et al., "Apache hadoop yarn: Yet another resource negotiator," pp. 1-16.
[100] J. Dean and S. Ghemawat, "MapReduce: simplified data processing on large clusters," Communications of the ACM, vol. 51, pp. 107-113, 2008.
[101] A. K. Gupta and S. Gupta, "Security issues in big data with cloud computing," Int J Sci Res Comput Sci Eng, vol. 5, pp. 27-32, 2017.
[102] M. Zaharia, M. Chowdhury, M. J. Franklin, S. Shenker, and I. Stoica, "Spark: Cluster computing with working sets," HotCloud, vol. 10, pp. 95-95, 2010.
[103] M. Zaharia, M. Chowdhury, T. Das, A. Dave, J. Ma, M. McCauley, et al., "Fast and interactive analytics over Hadoop data with Spark," Usenix Login, vol. 37, pp. 45-51, 2012.
[104] N. Marz, "History of Apache Storm and lessons learned," Thoughts from the Red Planet, vol. 10, 2014.