A Comparative Study of Ensemble Classifiers for Paddy Blast Disease Prediction Model
Varsha M.1 , Poornima B.2
Section:Research Paper, Product Type: Journal Paper
Volume-8 ,
Issue-9 , Page no. 91-100, Sep-2020
CrossRef-DOI: https://doi.org/10.26438/ijcse/v8i9.91100
Online published on Sep 30, 2020
Copyright © Varsha M., Poornima B. . This is an open access article distributed under the Creative Commons Attribution License, which permits unrestricted use, distribution, and reproduction in any medium, provided the original work is properly cited.
View this paper at Google Scholar | DPI Digital Library
How to Cite this Paper
- IEEE Citation
- MLA Citation
- APA Citation
- BibTex Citation
- RIS Citation
IEEE Style Citation: Varsha M., Poornima B., “A Comparative Study of Ensemble Classifiers for Paddy Blast Disease Prediction Model,” International Journal of Computer Sciences and Engineering, Vol.8, Issue.9, pp.91-100, 2020.
MLA Style Citation: Varsha M., Poornima B. "A Comparative Study of Ensemble Classifiers for Paddy Blast Disease Prediction Model." International Journal of Computer Sciences and Engineering 8.9 (2020): 91-100.
APA Style Citation: Varsha M., Poornima B., (2020). A Comparative Study of Ensemble Classifiers for Paddy Blast Disease Prediction Model. International Journal of Computer Sciences and Engineering, 8(9), 91-100.
BibTex Style Citation:
@article{M._2020,
author = {Varsha M., Poornima B.},
title = {A Comparative Study of Ensemble Classifiers for Paddy Blast Disease Prediction Model},
journal = {International Journal of Computer Sciences and Engineering},
issue_date = {9 2020},
volume = {8},
Issue = {9},
month = {9},
year = {2020},
issn = {2347-2693},
pages = {91-100},
url = {https://www.ijcseonline.org/full_paper_view.php?paper_id=5218},
doi = {https://doi.org/10.26438/ijcse/v8i9.91100}
publisher = {IJCSE, Indore, INDIA},
}
RIS Style Citation:
TY - JOUR
DO = {https://doi.org/10.26438/ijcse/v8i9.91100}
UR - https://www.ijcseonline.org/full_paper_view.php?paper_id=5218
TI - A Comparative Study of Ensemble Classifiers for Paddy Blast Disease Prediction Model
T2 - International Journal of Computer Sciences and Engineering
AU - Varsha M., Poornima B.
PY - 2020
DA - 2020/09/30
PB - IJCSE, Indore, INDIA
SP - 91-100
IS - 9
VL - 8
SN - 2347-2693
ER -
![]() |
![]() |
![]() |
226 | 226 downloads | 142 downloads |
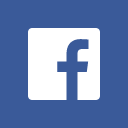
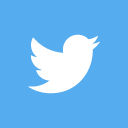
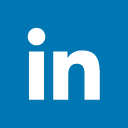
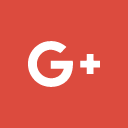
Abstract
Paddy blast has become most epidemic disease in many rice growing countries. Various statistical methods have been used for the prediction of paddy blast but previously used methods failed in predicting diseases with good accuracy. However, the need to develop new model that considers both weather factors and non-weather data called blast disease data that influences paddy disease to grow. Given this point we developed ensemble classifier-based paddy disease prediction model taking weather data from January 2013 to December 2019 from Agricultural and Horticulture Research Station Kathalgere, Davangere District. For the predictive model we collected 7 kinds of weather data and 7 kinds of disease related data that includes Minimum Temperature, Maximum Temperature, Temperature Difference, Relative Humidity, Stages of Paddy Cultivation, Varieties of seeds, Season of cropping and so on. It is observed and analyzed that Minimum Temperature, Humidity and Rainfall has huge correlation with occurrence of disease. In the collected data some of the variables are non-numeric to convert them to numeric data one hot encoding approach is followed and to improve efficiency of ensemble classifiers 4 different filter-based features selection methods are used such as Pearson’s correlation, Mutual information, ANNOVA F Value, Chi Square. Further three different ensemble classifiers are used as predictive models and classifiers are compared it is observed that Bagging ensemble technique has achieved accuracy of 98% compared to Adaboost of 97% and Voting classifier of 88%. Along with this other classification metrics are used evaluate Ensemble classifiers like precision, recall, F1 Score, ROC and precision recall score. Our proposed ensemble classifiers for paddy blast disease prediction has achieved high precision and high recall but when the solutions of model are closely looked bagging classifier is good compared to other ensemble classifiers that are proposed in predicting paddy blast disease.
Key-Words / Index Term
Paddy Blast Disease, Mutual Information, ANNOVA F Value, Voting Classifer, Bagging, Adaboost, Precision-recallScore,ROC
References
[1] Shafaullah, Muhammad Aslam Khan, Nasir Ahmed Khan And Yasir Mahmood, “Effect Of Epidemiological Factors On The Incidence Of Paddy Blast (Pyricularia Oryzae) Disease,” Pak J.Phytopayhol.,Vol 23(2):108-111, 2011.
[2] Kwang-Hyung Kima, Jaepil Cho, Yong Hwan Lee, Woo Seop Lee, “Predicting potential epidemics of rice leaf blast and sheath blight in South Korea under the RCP 4.5 and RCP 8.5 climate change scenarios using a rice disease epidemiology model, EPIRICE”, Elsevier Agricultural and Forest Meteorology 203(2015) 191 207.
[3] Yoshihiro Taguchi, Mohsen Mohamed Elsharkawy, Naglaa Hassan, Mitsuro Hyakumachi, “ A novel method for controlling rice blast disease using fan-forced wind on paddy fields”, Elsevier, Crop Protection 63(2014) 68-75.
[4] Kwang-Hyung Kim & Jaepil Cho, “Predicting potential epidemics of rice diseases in Korea using multi-model ensembles for assessment of climate change impacts with uncertainty information”, Springer Climatic Change (2016) 134:327–339 DOI 10.1007/s10584-015-1503-2.
[5] G. Miah, M. Y. Rafii, M. R. Ismail, M. Sahebi, F. S. G. Hashemi,O. Yusuff and M. G. Usman, “Blast Disease Intimidation Towards Rice Cultivation: A Review Of Pathogen And Strategies To Control”, The Journal of Animal & Plant Sciences, 27(4): 2017, Page: 1058-1066 ISSN: 1018-7081.
[6] Rupankar Bhagawati, Kaushik Bhagawati†, D. Jini, R. A. Alone, R. Singh, A. Chandra, B. Makdoh, Amit Sen and Kshitiz K. Shukla, “Review on Climate Change and its Impact on Agriculture of Arunachal Pradesh in the Northeastern Himalayan Region of India”, Nature Environment and Pollution Technology An International Quarterly Scientific Journal p-ISSN: 0972-6268 e-ISSN: 2395-3454 Vol. 16 No. 2 pp. 535-539 2017.
[7] Rajendra Prasad, Anupam Sharma and Sweta Sehgal, “ Influence of weather parameters on occurrence of rice blast in mid hills of Himachal Pradesh”, Himachal Journal of Agricultural Research 41(2): 132-136, 2015.
[8] Rakesh Kaundal, Amar S Kapoor and Gajendra PS Raghava, “Machine learning techniques in disease forecasting: a case study on rice blast prediction”, BMC Bioinformatics Journal 7:485 doi:10.1186/1471-2105-7-485, 2006.
[9] Y.H. Gu, S.J. Yoo, C.J. Park, Y.H. Kim, S.K. Park, J.S. Kim, J.H. Lim, “BLITE-SVR: New forecasting model for late blight on potato using support-vector regression”, Elsevier, Computers and Electronics in Agriculture 130(2016) 169-176.
[10] Jia-You Hsieh, Wei Huang, Hsin-Tieh Yang, Chia-Chieh Lin, Yo-Chung Fan, Huan Chen, “Building the Rice Blast Disease Prediction Model based on Machine Learning and Neural Networks”, EasyChair Preprint.
[11] Alvin R. Malicdem, Proceso L. Fernandez, “Rice Blast Disease Forecasting for Northern Philippines”, Article in WSEAS Transactions on Information Science and Applications • January 2015.
[12] Yangseon Kim, Jae-Hwan Roh and Ha Young Kim, “ Early Forecasting of Rice Blast Disease Using Long Short-Term Memory Recurrent Neural Networks”, Journal on Sustainability, 10, 34; doi:10.3390/su10010034 2018.
[13] Dimitrios Katsantonis1 , Kalliopi Kadoglidou, Christos Dramalis And Pau Puigdollers, “Rice blast forecasting models and their practical value: a review”, Journal on Phytopathology Mediterranean, 56, 2, 187?216 DOI: 10.14601/Phytopathol_Mediterr-18706 2017.
[14] Chethana B.S., Deepak, C.A., Rajanna, M.P., Ramachandra, C. and Shivakumar, N., “Current Scenario Of Rice Diseases In Karnataka”, International journal of science and Nature., VOL.7 (2) 405-412, 2016.
[15] P. B. Jawade, Dattatray Chaugule, Devashri Patil, and Hemendra Shinde, “ Disease Prediction of Mango Crop Using Machine Learning and IoT”, International Joint Conference on e-Business and Telecommunication( ICETE 2019) , LAIS 3, pp. 254–260, 2020.
[16] Karim Foughali, Karim Fathallah, Ali Frihida, “Using Cloud IOT for disease prevention in precision agriculture”, International Conference on Sustainable Energy Information Technology, SEIT 2018, Porto, Portugal May 2018.
[17] Sehan Kim, Meonghun Lee, Changsun Shin, “IoT-Based Strawberry Disease Prediction System for Smart Farming” , Sensors , 18, 4051; doi:10.3390/s18114051 2018.
[18] S. Ramesh and Bharghava Rajaram, “ Iot Based Crop Disease Identification System Using Optimization Techniques”, ARPN Journal of Engineering and Applied Sciences, ISSN 1819-6608 Vol. 13, No. 4, February 2018.
[19] Jennifer G. Dy, Carla E. Brodley, “Feature Selection for Unsupervised Learning”, Journal of Machine Learning Research 845–889, 2004.
[20] Shadi Aljawarneh, Muneer Bani Yassein, Monther Aldwair, “ Anomaly-based intrusion detection system through feature selection analysis and building hybrid efficient model”, Journal of Computational Science 152-160, 28, 2018.
[21] S.I. Mostafa , Y.M. Abd El-Latif , N.M. Reda, “ Fast And Accurate System For Leaf Recognition”, International Journal of Computer Sciences and Engineering Vol.8, Issue.8, August 2020.
[22] R. Thirumahal , G. Sudha Sadasivam, “Data Integration Techniques For Healthcare – A Comprehensive Survey”, International Journal of Computer Sciences and Engineering, Vol.8, Issue.8, August 2020.