Classification of Audio Segments using Voice Activity Detection
S. Kaur1 , P. Mittal2
Section:Research Paper, Product Type: Journal Paper
Volume-8 ,
Issue-9 , Page no. 101-105, Sep-2020
CrossRef-DOI: https://doi.org/10.26438/ijcse/v8i9.101105
Online published on Sep 30, 2020
Copyright © S. Kaur, P. Mittal . This is an open access article distributed under the Creative Commons Attribution License, which permits unrestricted use, distribution, and reproduction in any medium, provided the original work is properly cited.
View this paper at Google Scholar | DPI Digital Library
How to Cite this Paper
- IEEE Citation
- MLA Citation
- APA Citation
- BibTex Citation
- RIS Citation
IEEE Style Citation: S. Kaur, P. Mittal, “Classification of Audio Segments using Voice Activity Detection,” International Journal of Computer Sciences and Engineering, Vol.8, Issue.9, pp.101-105, 2020.
MLA Style Citation: S. Kaur, P. Mittal "Classification of Audio Segments using Voice Activity Detection." International Journal of Computer Sciences and Engineering 8.9 (2020): 101-105.
APA Style Citation: S. Kaur, P. Mittal, (2020). Classification of Audio Segments using Voice Activity Detection. International Journal of Computer Sciences and Engineering, 8(9), 101-105.
BibTex Style Citation:
@article{Kaur_2020,
author = {S. Kaur, P. Mittal},
title = {Classification of Audio Segments using Voice Activity Detection},
journal = {International Journal of Computer Sciences and Engineering},
issue_date = {9 2020},
volume = {8},
Issue = {9},
month = {9},
year = {2020},
issn = {2347-2693},
pages = {101-105},
url = {https://www.ijcseonline.org/full_paper_view.php?paper_id=5219},
doi = {https://doi.org/10.26438/ijcse/v8i9.101105}
publisher = {IJCSE, Indore, INDIA},
}
RIS Style Citation:
TY - JOUR
DO = {https://doi.org/10.26438/ijcse/v8i9.101105}
UR - https://www.ijcseonline.org/full_paper_view.php?paper_id=5219
TI - Classification of Audio Segments using Voice Activity Detection
T2 - International Journal of Computer Sciences and Engineering
AU - S. Kaur, P. Mittal
PY - 2020
DA - 2020/09/30
PB - IJCSE, Indore, INDIA
SP - 101-105
IS - 9
VL - 8
SN - 2347-2693
ER -
![]() |
![]() |
![]() |
150 | 267 downloads | 115 downloads |
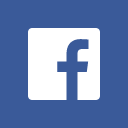
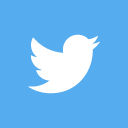
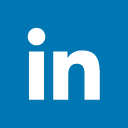
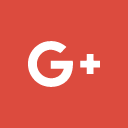
Abstract
Voice activity detection is classifying speech and non-speech frames. Effectively working and noise tolerant voice activity detection technique is responsible for better performance of many new speech technologies in the area of speech processing. In this paper, an unsupervised method for VAD is proposed to identify the segments of speech- presence and speech-absence in an audio. To make the presented algorithm effective and computationally fast, it is implemented by using long-term parameters that are extracted by using Petrosian algorithm used for fractal dimensions. This system plays a significant role in terms of achieving improved speech quality. Two types of datasets recorded in English and Arabic languages are used to analyses the output of the proposed algorithm. An Array of 85 audio signals of TIMIT Database, of different Signal to noise ratios is tested using the algorithm at once. The evaluated performance suggested that the proposed algorithm identifies segments in the audios with different SNR’s
Key-Words / Index Term
Fractal Dimensions
References
[1] J. Sohn, N. S. Kim, and W. Sung, “A statistical model-based voice activity detection,‘‘ IEEE Signal Process. Lett., vol. 6, no. 1, pp. 1–3, Jan. 1999.
[2] J. Ramirez, J. C. Segura, C. Benitez, L. Garcia, and A. Rubio, “Statistical voice activity detection using a multiple observation likelihood ratio test,‘‘ IEEE Signal Process. Lett., vol. 12, no. 10, pp. 689–692, Oct. 2005.
[3] J.-H. Chang, N. S. Kim, and S. K. Mitra, “Voice activity detection based on multiple statistical models,‘‘ IEEE Trans. Signal Process., vol. 54, no. 6, pp. 1965–1976, Jun. 2006.
[4] J. Wu and X.-L. Zhang, “Maximum margin clustering based statistical VAD with multiple observation compound feature, ‘‘ IEEE Signal Process. Lett., vol. 18, no. 5, pp. 283–286, May 2011.
[5] S. Mudaliar , T.Tahilramani, “Techniques of voice activity detection: A review? in “IJSRD - International Journal for Scientific Research & Development? Vol. 5, Issue 02, 2017
[6] R. Esteller, G. Vachtsevanos, J. Echauz, and B. Litt, “A comparison of waveform fractal dimension algorithms,‘‘ IEEE Trans. Circuits Syst. I, Fundam. Theory Appl., vol. 48, no. 2, pp. 177–183, Feb. 2001.
[7] Z.Ali, M.Talha, “ Innovative method for unsupervised voice activity detection and classification of audio segments, in IEEE Int. Conf., Special section on radio frequency identification and security technique , Vol no.6 April 2018.
[8] L. N. Tan, B. J. Borgstrom, and A. Alwan, “Voice activity detection using harmonic frequency components in likelihood ratio test,‘‘ in Proc. IEEE Int. Conf. Acoust., Speech Signal Process., Mar. 2010, pp. 4466–4469.
[9] M.M. Alsulaiman, G. Muhammd, M. A. Bencherif, A. Mahmood, and Z. Ali, “KSU rich Arabic speech database,‘‘ J. Inf., vol. 16, no. 6, pp. 4231–4253, 2013.
[10] R. J. Moran, R. B. Reilly, P. de Chazal, and P. D. Lacy, “Telephonybased voice pathology assessment using automated speech analysis,‘‘ IEEE Trans. Biomed. Eng., vol. 53, no. 3, pp. 468–477, Mar. 2006.
[11] T. R. Senevirathne, E. L. J. Bohez, and J. A. Van Winden, “Amplitude scale method: New and efficient approach to measure fractal dimension of speech waveforms,‘‘ Electron. Lett., vol. 28, no. 4, pp. 420–422, Feb. 1992.