Statistical Modeling for Sentiment Classification: A Review
Varsha Pal1 , Akshay Varkale2
Section:Review Paper, Product Type: Journal Paper
Volume-8 ,
Issue-10 , Page no. 100-105, Oct-2020
CrossRef-DOI: https://doi.org/10.26438/ijcse/v8i10.100105
Online published on Oct 31, 2020
Copyright © Varsha Pal, Akshay Varkale . This is an open access article distributed under the Creative Commons Attribution License, which permits unrestricted use, distribution, and reproduction in any medium, provided the original work is properly cited.
View this paper at Google Scholar | DPI Digital Library
How to Cite this Paper
- IEEE Citation
- MLA Citation
- APA Citation
- BibTex Citation
- RIS Citation
IEEE Style Citation: Varsha Pal, Akshay Varkale, “Statistical Modeling for Sentiment Classification: A Review,” International Journal of Computer Sciences and Engineering, Vol.8, Issue.10, pp.100-105, 2020.
MLA Style Citation: Varsha Pal, Akshay Varkale "Statistical Modeling for Sentiment Classification: A Review." International Journal of Computer Sciences and Engineering 8.10 (2020): 100-105.
APA Style Citation: Varsha Pal, Akshay Varkale, (2020). Statistical Modeling for Sentiment Classification: A Review. International Journal of Computer Sciences and Engineering, 8(10), 100-105.
BibTex Style Citation:
@article{Pal_2020,
author = {Varsha Pal, Akshay Varkale},
title = {Statistical Modeling for Sentiment Classification: A Review},
journal = {International Journal of Computer Sciences and Engineering},
issue_date = {10 2020},
volume = {8},
Issue = {10},
month = {10},
year = {2020},
issn = {2347-2693},
pages = {100-105},
url = {https://www.ijcseonline.org/full_paper_view.php?paper_id=5239},
doi = {https://doi.org/10.26438/ijcse/v8i10.100105}
publisher = {IJCSE, Indore, INDIA},
}
RIS Style Citation:
TY - JOUR
DO = {https://doi.org/10.26438/ijcse/v8i10.100105}
UR - https://www.ijcseonline.org/full_paper_view.php?paper_id=5239
TI - Statistical Modeling for Sentiment Classification: A Review
T2 - International Journal of Computer Sciences and Engineering
AU - Varsha Pal, Akshay Varkale
PY - 2020
DA - 2020/10/31
PB - IJCSE, Indore, INDIA
SP - 100-105
IS - 10
VL - 8
SN - 2347-2693
ER -
![]() |
![]() |
![]() |
171 | 191 downloads | 110 downloads |
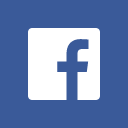
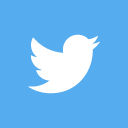
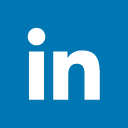
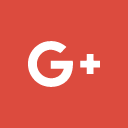
Abstract
Sentiment classification is the process of using NLP, statistics, or machine learning methods to extract, identify, or otherwise characterize the sentiment content of a text unit. Based on a sample of tweets, how are people responding to this ad campaign/product release/news item? There are several application of opinion mining such as on business intelligence, Politics/political science, Law/policy making, Sociology, Psychology etc. By use of digital platform administration can collect response from consumer and by means of applying opinion mining technique a useful information from user collected data. In this paper we have given a brief review over different work done in the field of sentiment classification and given tabular comparison among different opinion classification technique based on accuracy.
Key-Words / Index Term
TPR,FNR,ML,NL,SVM ANN
References
[1] B. Vamshi Krishna, Ajeet Kumar Pandey and A. P. Siva Kumar Feature Based Opinion Mining and Sentiment Analysis Using Fuzzy Logic Springer 2018.
[2] Y. Wang, J. Zhang Keyword Extraction from Online Product Reviews Based on Bi-Directional LSTM Recurrent Neural Network IEEE 2017.
[3] Sandra Garcia Esparza , Michael P. O’Mahony, Barry Smyth Mining the real-time web: A novel approach to product recommendation Elsevier 2011.
[4] Isa Maks , Piek Vossen A lexicon model for deep sentiment analysis and opinion mining applications Elsevier 2012.
[5] Rodrigo Moraes, João Francisco Valiati, Wilson P. Gavião Neto Document-level sentiment classification: An empirical comparison between SVM and ANN Elsevier 2013.
[6] Tarik S. Zakzouk Comparing text classifiers for sports news Elsevier 2012.
[7] Ngoc Phuong Chau, Viet Anh Phan, Minh Le Nguyen Deep Learning and Sub-Tee Mining for Document Level Sentiment Classification KSE 2016.
[8] Ivo Danihelka et. al. Associative Long Short-Term Memory arXiv 2016.