A Survey on Feature Selection in Microarray Data: Methods, Algorithms and Challenges
Khadija Abdullah Uthman1 , Fadl Mutaher Ba-Alwi2 , Suad Mohammed Othman3
Section:Survey Paper, Product Type: Journal Paper
Volume-8 ,
Issue-10 , Page no. 106-116, Oct-2020
CrossRef-DOI: https://doi.org/10.26438/ijcse/v8i10.106116
Online published on Oct 31, 2020
Copyright © Khadija Abdullah Uthman, Fadl Mutaher Ba-Alwi, Suad Mohammed Othman . This is an open access article distributed under the Creative Commons Attribution License, which permits unrestricted use, distribution, and reproduction in any medium, provided the original work is properly cited.
View this paper at Google Scholar | DPI Digital Library
How to Cite this Paper
- IEEE Citation
- MLA Citation
- APA Citation
- BibTex Citation
- RIS Citation
IEEE Style Citation: Khadija Abdullah Uthman, Fadl Mutaher Ba-Alwi, Suad Mohammed Othman, “A Survey on Feature Selection in Microarray Data: Methods, Algorithms and Challenges,” International Journal of Computer Sciences and Engineering, Vol.8, Issue.10, pp.106-116, 2020.
MLA Style Citation: Khadija Abdullah Uthman, Fadl Mutaher Ba-Alwi, Suad Mohammed Othman "A Survey on Feature Selection in Microarray Data: Methods, Algorithms and Challenges." International Journal of Computer Sciences and Engineering 8.10 (2020): 106-116.
APA Style Citation: Khadija Abdullah Uthman, Fadl Mutaher Ba-Alwi, Suad Mohammed Othman, (2020). A Survey on Feature Selection in Microarray Data: Methods, Algorithms and Challenges. International Journal of Computer Sciences and Engineering, 8(10), 106-116.
BibTex Style Citation:
@article{Uthman_2020,
author = {Khadija Abdullah Uthman, Fadl Mutaher Ba-Alwi, Suad Mohammed Othman},
title = {A Survey on Feature Selection in Microarray Data: Methods, Algorithms and Challenges},
journal = {International Journal of Computer Sciences and Engineering},
issue_date = {10 2020},
volume = {8},
Issue = {10},
month = {10},
year = {2020},
issn = {2347-2693},
pages = {106-116},
url = {https://www.ijcseonline.org/full_paper_view.php?paper_id=5240},
doi = {https://doi.org/10.26438/ijcse/v8i10.106116}
publisher = {IJCSE, Indore, INDIA},
}
RIS Style Citation:
TY - JOUR
DO = {https://doi.org/10.26438/ijcse/v8i10.106116}
UR - https://www.ijcseonline.org/full_paper_view.php?paper_id=5240
TI - A Survey on Feature Selection in Microarray Data: Methods, Algorithms and Challenges
T2 - International Journal of Computer Sciences and Engineering
AU - Khadija Abdullah Uthman, Fadl Mutaher Ba-Alwi, Suad Mohammed Othman
PY - 2020
DA - 2020/10/31
PB - IJCSE, Indore, INDIA
SP - 106-116
IS - 10
VL - 8
SN - 2347-2693
ER -
![]() |
![]() |
![]() |
306 | 517 downloads | 175 downloads |
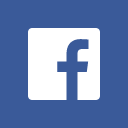
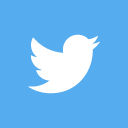
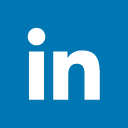
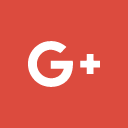
Abstract
In biomedical researches a massive amount of data are produced day after day, using machine learning algorithms to discover the knowledge is very important in early diagnosis, prevention and treatment, as well as drug development. Biomedical data like DNA microarray suffers from curse of dimensionality phenomenon, since there are a huge number of features (genes) with high ambiguity. Feature selection is still a hot topic which cares about reducing the high of dimensionality by applying different techniques. Different contributions are conducted with new models, frameworks, methodologies and algorithms aiming to dissolve the curse of dimensionality problem and produce more meaningful and reliable data. The objective of this study is to explain the concept of feature selection, its methods, the algorithms and techniques that have been recently used in microarray data and the most popular microarray datasets were used. Moreover, the challenges that can appear when selecting more informative and non-redundant features from high dimensional datasets.
Key-Words / Index Term
Feature Selection, Filter Method, Wrapper Method, Hybrid Method, DNA microarray, Metaheuristic
References
[1] R. K. Bania and A. Halder, "R-Ensembler: A greedy rough set based ensemble attribute selection algorithm with kNN imputation for classification of medical data," Computer Methods and Programs in Biomedicine, vol. 184, p. 105122, 2020.
[2] L. Venkataramana, S. G. Jacob, R. Ramadoss, D. Saisuma, D. Haritha, and K. Manoja, "Improving classification accuracy of cancer types using parallel hybrid feature selection on microarray gene expression data," Genes & genomics, vol. 41, pp. 1301-1313, 2019.
[3] B. Remeseiro and V. Bolon-Canedo, "A review of feature selection methods in medical applications," Computers in biology and medicine, p. 103375, 2019.
[4] K. Tadist, S. Najah, N. S. Nikolov, F. Mrabti, and A. Zahi, "Feature selection methods and genomic big data: a systematic review," Journal of Big Data, vol. 6, p. 79, 2019.
[5] K. Zheng, X. Wang, B. Wu, and T. Wu, "Feature subset selection combining maximal information entropy and maximal information coefficient," Applied Intelligence, pp. 1-15, 2019.
[6] N. Sánchez-Maroño, O. Fontenla-Romero, and B. Pérez-Sánchez, "Classification of Microarray Data," in Microarray Bioinformatics, ed: Springer, 2019, pp. 185-205.
[7] A. Alonso-Betanzos, V. Bolón-Canedo, L. Morán-Fernández, and B. Seijo-Pardo, "Feature Selection Applied to Microarray Data," in Microarray Bioinformatics, ed: Springer, 2019, pp. 123-152.
[8] S. Sayed, M. Nassef, A. Badr, and I. Farag, "A nested genetic algorithm for feature selection in high-dimensional cancer microarray datasets," Expert Systems with Applications, vol. 121, pp. 233-243, 2019.
[9] J. Li, K. Cheng, S. Wang, F. Morstatter, R. P. Trevino, J. Tang, et al., "Feature selection: A data perspective," ACM Computing Surveys (CSUR), vol. 50, p. 94, 2018.
[10] E. Hoseini and E. G. Mansoori, "Unsupervised feature selection in linked biological data," Pattern Analysis and Applications, vol. 22, pp. 999-1013, 2019.
[11] Y. Huo, L. Xin, C. Kang, M. Wang, Q. Ma, and B. Yu, "SGL-SVM: A novel method for tumor classification via support vector machine with sparse group Lasso," Journal of Theoretical Biology, vol. 486, p. 110098, 2020.
[12] A. K. Shukla, D. Tripathi, B. R. Reddy, and D. Chandramohan, "A study on metaheuristics approaches for gene selection in microarray data: algorithms, applications and open challenges," Evolutionary Intelligence, pp. 1-21, 2019.
[13] N. AlNuaimi, M. M. Masud, M. A. Serhani, and N. Zaki, "Streaming Feature Selection Algorithms for Big Data: A Survey," Applied Computing and Informatics, 2019.
[14] X. H. Han, D. A. Li, and L. Wang, "A Hybrid Cancer Classification Model Based Recursive Binary Gravitational Search Algorithm in Microarray Data," Procedia Computer Science, vol. 154, pp. 274-282, 2019.
[15] M. Ghosh, S. Begum, R. Sarkar, D. Chakraborty, and U. Maulik, "Recursive memetic algorithm for gene selection in microarray data," Expert Systems with Applications, vol. 116, pp. 172-185, 2019.
[16] X. Zheng, W. Zhu, C. Tang, and M. Wang, "Gene selection for microarray data classification via adaptive hypergraph embedded dictionary learning," Gene, vol. 706, pp. 188-200, 2019.
[17] Y. Zhang, Q. Zhang, Z. Chen, J. Shang, and H. Wei, "Feature assessment and ranking for classification with nonlinear sparse representation and approximate dependence analysis," Decision Support Systems, vol. 122, p. 113064, 2019.
[18] M. Sun, K. Liu, Q. Wu, Q. Hong, B. Wang, and H. Zhang, "A novel ECOC algorithm for multiclass microarray data classification based on data complexity analysis," Pattern Recognition, vol. 90, pp. 346-362, 2019.
[19] L. Sun, X.-Y. Zhang, Y.-H. Qian, J.-C. Xu, S.-G. Zhang, and Y. Tian, "Joint neighborhood entropy-based gene selection method with fisher score for tumor classification," Applied Intelligence, vol. 49, pp. 1245-1259, 2019.
[20] S. Kiliçarslan, K. Adem, and M. Çelik, "Diagnosis and Classification of Cancer Using Hybrid Model Based on ReliefF and Convolutional Neural Network," Medical Hypotheses, p. 109577, 2020.
[21] G. Agapito, "Computer Tools to Analyze Microarray Data," in Microarray Bioinformatics, ed: Springer, 2019, pp. 267-282.
[22] J. Chaki and N. Dey, "Pattern analysis of genetics and genomics: a survey of the state-of-art," Multimedia Tools and Applications, pp. 1-32, 2019.
[23] A. Sharma and R. Rani, "C-HMOSHSSA: Gene selection for cancer classification using multi-objective meta-heuristic and machine learning methods," Computer methods and programs in biomedicine, vol. 178, pp. 219-235, 2019.
[24] M. A. Al-Betar, O. A. Alomari, and S. M. Abu-Romman, "A TRIZ-inspired bat algorithm for gene selection in cancer classification," Genomics, 2019.
[25] M. Mafarja, A. A. Heidari, H. Faris, S. Mirjalili, and I. Aljarah, "Dragonfly algorithm: theory, literature review, and application in feature selection," in Nature-Inspired Optimizers, ed: Springer, 2020, pp. 47-67.
[26] C. Kang, Y. Huo, L. Xin, B. Tian, and B. Yu, "Feature selection and tumor classification for microarray data using relaxed Lasso and generalized multi-class support vector machine," Journal of theoretical biology, vol. 463, pp. 77-91, 2019.
[27] J. Ge, X. Zhang, G. Liu, and Y. Sun, "A Novel Feature Selection Algorithm Based on Artificial Bee Colony Algorithm and Genetic Algorithm," in 2019 IEEE International Conference on Power, Intelligent Computing and Systems (ICPICS), 2019, pp. 131-135.
[28] C. Tang, L. Cao, X. Zheng, and M. Wang, "Gene selection for microarray data classification via subspace learning and manifold regularization," Medical & biological engineering & computing, vol. 56, pp. 1271-1284, 2018.
[29] S. Bakhshandeh, R. Azmi, and M. Teshnehlab, "Symmetric uncertainty class-feature association map for feature selection in microarray dataset," International Journal of Machine Learning and Cybernetics, vol. 11, pp. 15-32, 2020.
[30] S. A. Medjahed, T. A. Saadi, A. Benyettou, and M. Ouali, "Kernel-based learning and feature selection analysis for cancer diagnosis," Applied Soft Computing, vol. 51, pp. 39-48, 2017.
[31] A. K. Shukla and D. Tripathi, "Identification of potential biomarkers on microarray data using distributed gene selection approach," Mathematical biosciences, vol. 315, p. 108230, 2019.
[32] M. Alirezanejad, R. Enayatifar, H. Motameni, and H. Nematzadeh, "Heuristic filter feature selection methods for medical datasets," Genomics, 2019.
[33] M. J. Rani and D. Devaraj, "Two-Stage Hybrid Gene Selection Using Mutual Information and Genetic Algorithm for Cancer Data Classification," Journal of medical systems, vol. 43, p. 235, 2019.
[34] A. K. Shukla, P. Singh, and M. Vardhan, "A hybrid gene selection method for microarray recognition," Biocybernetics and Biomedical Engineering, vol. 38, pp. 975-991, 2018.
[35] J. Xie, M. Hao, W. Liu, and Y. Lin, "Fused variable screening for massive imbalanced data," Computational Statistics & Data Analysis, vol. 141, pp. 94-108, 2020.
[36] A. K. Shukla, P. Singh, and M. Vardhan, "A two-stage gene selection method for biomarker discovery from microarray data for cancer classification," Chemometrics and Intelligent Laboratory Systems, vol. 183, pp. 47-58, 2018.
[37] P. Lopez-Garcia, A. D. Masegosa, E. Osaba, E. Onieva, and A. Perallos, "Ensemble classification for imbalanced data based on feature space partitioning and hybrid metaheuristics," Applied Intelligence, vol. 49, pp. 2807-2822, 2019.
[38] Y. He, J. Zhou, Y. Lin, and T. Zhu, "A class imbalance-aware Relief algorithm for the classification of tumors using microarray gene expression data," Computational Biology and Chemistry, vol. 80, pp. 121-127, 2019.
[39] M. Ghosh, S. Adhikary, K. K. Ghosh, A. Sardar, S. Begum, and R. Sarkar, "Genetic algorithm based cancerous gene identification from microarray data using ensemble of filter methods," Medical & biological engineering & computing, vol. 57, pp. 159-176, 2019.
[40] S. Ramírez?Gallego, I. Lastra, D. Martínez?Rego, V. Bolón?Canedo, J. M. Benítez, F. Herrera, et al., "Fast?mRMR: Fast Minimum Redundancy Maximum Relevance Algorithm for High?Dimensional Big Data," International Journal of Intelligent Systems, vol. 32, pp. 134-152, 2017.
[41] S. H. Bouazza, K. Auhmani, A. Zeroual, and N. Hamdi, "Selecting significant marker genes from microarray data by filter approach for cancer diagnosis," Procedia Computer Science, vol. 127, pp. 300-309, 2018.
[42] W. Andaru, I. Syarif, and A. R. Barakbah, "Feature selection software development using artificial bee colony on dna microarray data," in 2017 International Electronics Symposium on Knowledge Creation and Intelligent Computing (IES-KCIC), 2017, pp. 6-11.
[43] M. Allam and M. Nandhini, "Optimal feature selection using binary teaching learning based optimization algorithm," Journal of King Saud University-Computer and Information Sciences, 2018.
[44] S. K. Baliarsingh, S. Vipsita, and B. Dash, "A new optimal gene selection approach for cancer classification using enhanced Jaya-based forest optimization algorithm," Neural Computing and Applications, pp. 1-18, 2019.
[45] M. A. Basir, M. S. Hussin, and Y. Yusof, "Ideal Combination Feature Selection Model for Classification Problem based on Bio-Inspired Approach," in Computational Science and Technology, ed: Springer, 2020, pp. 585-593.
[46] L. Zhang, W. Zhou, B. Wang, Z. Zhang, and F. Li, "Applying 1-norm SVM with squared loss to gene selection for cancer classification," Applied Intelligence, vol. 48, pp. 1878-1890, 2018.
[47] X. Huang, L. Zhang, B. Wang, F. Li, and Z. Zhang, "Feature clustering based support vector machine recursive feature elimination for gene selection," Applied Intelligence, vol. 48, pp. 594-607, 2018.
[48] M. S. M. Prince, A. Hasan, and F. M. Shah, "An Efficient Ensemble Method for Cancer Detection," in 2019 1st International Conference on Advances in Science, Engineering and Robotics Technology (ICASERT), 2019, pp. 1-6.
[49] T. Gangavarapu and N. Patil, "A novel filter–wrapper hybrid greedy ensemble approach optimized using the genetic algorithm to reduce the dimensionality of high-dimensional biomedical datasets," Applied Soft Computing, vol. 81, p. 105538, 2019.
[50] T. Ragunthar and S. Selvakumar, "A wrapper based feature selection in bone marrow plasma cell gene expression data," Cluster Computing, vol. 22, pp. 13785-13796, 2019.
[51] P. Singh, A. Shukla, and M. Vardhan, "Hybrid approach for gene selection and classification using filter and genetic algorithm," in 2017 International Conference on Inventive Computing and Informatics (ICICI), 2017, pp. 832-837.
[52] K. Passi, A. Nour, and C. K. Jain, "Markov blanket: Efficient strategy for feature subset selection method for high dimensional microarray cancer datasets," in 2017 IEEE International Conference on Bioinformatics and Biomedicine (BIBM), 2017, pp. 1864-1871.
[53] D. Utami and Z. Rustam, "Gene selection in cancer classification using hybrid method based on Particle Swarm Optimization (PSO), Artificial Bee Colony (ABC) feature selection and support vector machine," in AIP Conference Proceedings, 2019, p. 020047.
[54] T. Umamaheswari and P. Sumathi, "Enhanced firefly algorithm (EFA) based gene selection and adaptive neuro neutrosophic inference system (ANNIS) prediction model for detection of circulating tumor cells (CTCs) in breast cancer analysis," Cluster Computing, vol. 22, pp. 14035-14047, 2019.
[55] S. Singla, P. Ghosh, and U. Kumari, "Breast Cancer Detection using Genetic Algorithm with Correlation based Feature Selection: Experiment on Different Datasets," International Journal of Computer Sciences and Engineering, vol. 7, pp. 406-410, 2019.
[56] Y. Prasad, K. Biswas, and M. Hanmandlu, "A recursive PSO scheme for gene selection in microarray data," Applied Soft Computing, vol. 71, pp. 213-225, 2018.
[57] V. J. M. Praveena, "Particle Swarm Optimization based Feature Selection with Evolutionary Outlay-Aware Deep Belief Network Classifier (PSO-EOA-DBNC) for High Dimensional Datasets," International Journal of Computer Sciences and Engineering, vol. 7, pp. 61-69, 2019.