Machine Learning Techniques for Cancer Prediction: A Survey
Archana Pathak1 , Nidhi Ruthia2 , Chetan Agrawal3
Section:Survey Paper, Product Type: Journal Paper
Volume-8 ,
Issue-10 , Page no. 174-179, Oct-2020
CrossRef-DOI: https://doi.org/10.26438/ijcse/v8i10.174179
Online published on Oct 31, 2020
Copyright © Archana Pathak, Nidhi Ruthia, Chetan Agrawal . This is an open access article distributed under the Creative Commons Attribution License, which permits unrestricted use, distribution, and reproduction in any medium, provided the original work is properly cited.
View this paper at Google Scholar | DPI Digital Library
How to Cite this Paper
- IEEE Citation
- MLA Citation
- APA Citation
- BibTex Citation
- RIS Citation
IEEE Style Citation: Archana Pathak, Nidhi Ruthia, Chetan Agrawal, “Machine Learning Techniques for Cancer Prediction: A Survey,” International Journal of Computer Sciences and Engineering, Vol.8, Issue.10, pp.174-179, 2020.
MLA Style Citation: Archana Pathak, Nidhi Ruthia, Chetan Agrawal "Machine Learning Techniques for Cancer Prediction: A Survey." International Journal of Computer Sciences and Engineering 8.10 (2020): 174-179.
APA Style Citation: Archana Pathak, Nidhi Ruthia, Chetan Agrawal, (2020). Machine Learning Techniques for Cancer Prediction: A Survey. International Journal of Computer Sciences and Engineering, 8(10), 174-179.
BibTex Style Citation:
@article{Pathak_2020,
author = {Archana Pathak, Nidhi Ruthia, Chetan Agrawal},
title = {Machine Learning Techniques for Cancer Prediction: A Survey},
journal = {International Journal of Computer Sciences and Engineering},
issue_date = {10 2020},
volume = {8},
Issue = {10},
month = {10},
year = {2020},
issn = {2347-2693},
pages = {174-179},
url = {https://www.ijcseonline.org/full_paper_view.php?paper_id=5250},
doi = {https://doi.org/10.26438/ijcse/v8i10.174179}
publisher = {IJCSE, Indore, INDIA},
}
RIS Style Citation:
TY - JOUR
DO = {https://doi.org/10.26438/ijcse/v8i10.174179}
UR - https://www.ijcseonline.org/full_paper_view.php?paper_id=5250
TI - Machine Learning Techniques for Cancer Prediction: A Survey
T2 - International Journal of Computer Sciences and Engineering
AU - Archana Pathak, Nidhi Ruthia, Chetan Agrawal
PY - 2020
DA - 2020/10/31
PB - IJCSE, Indore, INDIA
SP - 174-179
IS - 10
VL - 8
SN - 2347-2693
ER -
![]() |
![]() |
![]() |
180 | 258 downloads | 153 downloads |
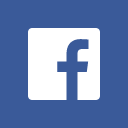
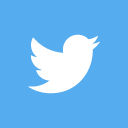
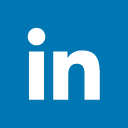
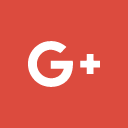
Abstract
In the present age of innovation medicinal field researcher are very much interested in disease classification for the analysis of disease. It is a point of concern on the grounds that real treatment of this disease isn`t found till date. Patients having this ailment must be spared if and just in the event that it is found in beginning period (arrange I and stage II). In the event that it is identified in last stage (arrange III and stage IV) at that point possibility of endurance will be exceptionally less. Machine learning and information mining system will assist medicinal with handling to handle with this issue. Cancer growth has different manifestations, for example, tumor, abnormal bleeding, more weight reduction and so forth. It isn`t vital that a wide range of tumors are harmful. Tumors are fundamentally of two kinds one is benign and the other one is malignant. To give suitable treatment to the patients, side effects must be contemplated appropriately and a programmed expectation framework is required which will characterize the tumor into benevolent or harmful. In the present web world, majority of information is created via web-based networking media or medicinal services sites. From this immense measure of information, side effects can be gotten by utilizing information mining method, which will be further helpful for disease location or classification. This paper makes study of such most recent research study that utilizes on the web and disconnected information for malignant growth arrangement.
Key-Words / Index Term
Tumors, Machine Learning, binary classification, malicious, gene expression
References
[1] R. A. Radcliffe, “Gene expression,” in Neurobehavioral Genetics: Methods and Applications, Second Edition, 2006.
[2] D. Oliva, M. Abd Elaziz, and S. Hinojosa, “Image Processing,” in Studies in Computational Intelligence, 2019.
[3] N. Guenther and M. Schonlau, “Support vector machines,” Stata J., 2016.
[4] C. Bulac and A. Bulac, “Decision Trees,” in Advanced Solutions in Power Systems: HVDC, FACTS, and AI Techniques, 2016.
[5] T. G. Clarkson, “Introduction to neural networks,” Neural Netw. World, 1996.
[6] K. R. Koch, Introduction to bayesian statistics. 2007.
[7] P. J. García-Laencina, J. L. Sancho-Gómez, A. R. Figueiras-Vidal, and M. Verleysen, “K nearest neighbours with mutual information for simultaneous classification and missing data imputation,” Neurocomputing, 2009.
[8] O. Fortunato et al., “Assessment of circulating micrornas in plasma of lung cancer patients,” Molecules, 2014.
[9] S. Michiels, S. Koscielny, and C. Hill, “Prediction of cancer outcome with microarrays: A multiple random validation strategy,” Lancet, 2005.
[10] Y. C. Chen, W. C. Ke, and H. W. Chiu, “Risk classification of cancer survival using ANN with gene expression data from multiple laboratories,” Comput. Biol. Med., 2014.
[11] K. Park, A. Ali, D. Kim, Y. An, M. Kim, and H. Shin, “Robust predictive model for evaluating breast cancer survivability,” Eng. Appl. Artif. Intell., 2013.
[12] X. Xu, Y. Zhang, L. Zou, M. Wang, and A. Li, “A gene signature for breast cancer prognosis using support vector machine,” in 2012 5th International Conference on Biomedical Engineering and Informatics, BMEI 2012, 2012.
[13] O. Gevaert, F. De Smet, D. Timmerman, Y. Moreau, and B. De Moor, “Predicting the prognosis of breast cancer by integrating clinical and microarray data with Bayesian networks,” in Bioinformatics, 2006.
[14] P. Rosado, P. Lequerica-Fernandez, L. Villallain, I. Pena, F. Sanchez-Lasheras, and J. C. De Vicente, “Survival model in oral squamous cell carcinoma based on clinicopathological parameters, molecular markers and support vector machines,” Expert Syst. Appl., 2013.
[15] D. Delen, G. Walker, and A. Kadam, “Predicting breast cancer survivability: A comparison of three data mining methods,” Artif. Intell. Med., 2005.
[16] J. Kim and H. Shin, “Breast cancer survivability prediction using labeled, unlabeled, and pseudo-labeled patient data,” J. Am. Med. Informatics Assoc., 2013.
[17] “Pattern Recognition and Machine Learning,” J. Electron. Imaging, 2007.
[18] A. Agah, A. Niknejad, and D. Petrovic, “Introduction to Computational Intelligence Techniques and Areas of Their Applications in Medicine,” in Medical Applications of Artificial Intelligence, 2013.
[19] “Introduction to data mining,” Intell. Syst. Ref. Libr., 2011.
[20] R. Nair and A. Bhagat, “A Life Cycle on Processing Large Dataset - LCPL,” Int. J. Comput. Appl., 2018.
[21] Y. Drier and E. Domany, “Do two machine-learning based prognostic signatures for breast cancer capture the same biological processes?,” PLoS One, 2011.
[22] M. Greenacre and R. Primicerio, “Measures of distance between samples: Euclidean,” in Multivariate Analysis of Ecological Data, 2013.
[23] J. A. Nelder and R. W. M. Wedderburn, “Generalized Linear Models,” J. R. Stat. Soc. Ser. A J. R. Stat. Soc. Ser. A (General J. R. Stat. Soc. A, 1972.
[24] Martin, “Two-way ANOVA and ANCOVA,” None, 1000.
[25] R. Berk and J. M. MacDonald, “Overdispersion and poisson regression,” J. Quant. Criminol., 2008.
[26] K. Kourou, T. P. Exarchos, K. P. Exarchos, M. V Karamouzis, and D. I. Fotiadis, “Machine learning applications in cancer prognosis and prediction,” CSBJ, vol. 13, pp. 8–17, 2015.
[27] E. Alpayd?n, Introduction to machine learning, vol. 1107. 2014.
[28] D. P. R. G and R. T. Sriramaneni, “Literature Survey on Various Software Cost,” vol. 4, no. Iv, pp. 868–874, 2016.
[29] D. Mladeni?, J. Brank, M. Grobelnik, and I. Natasa Milic-Frayling, “Feature Selection using Linear Classifier Weights: Interaction with Classification Models,” in Proceedings of the 27th annual international ACM SIGIR conference on Research and development in information retrieval, 2004.