Prediction of Type 2 Diabetics besed on Clustering Algorithm
K. Gandhimathi1 , N. Umadevi2
Section:Research Paper, Product Type: Journal Paper
Volume-8 ,
Issue-11 , Page no. 72-78, Nov-2020
CrossRef-DOI: https://doi.org/10.26438/ijcse/v8i11.7278
Online published on Nov 30, 2020
Copyright © K. Gandhimathi, N. Umadevi . This is an open access article distributed under the Creative Commons Attribution License, which permits unrestricted use, distribution, and reproduction in any medium, provided the original work is properly cited.
View this paper at Google Scholar | DPI Digital Library
How to Cite this Paper
- IEEE Citation
- MLA Citation
- APA Citation
- BibTex Citation
- RIS Citation
IEEE Style Citation: K. Gandhimathi, N. Umadevi, “Prediction of Type 2 Diabetics besed on Clustering Algorithm,” International Journal of Computer Sciences and Engineering, Vol.8, Issue.11, pp.72-78, 2020.
MLA Style Citation: K. Gandhimathi, N. Umadevi "Prediction of Type 2 Diabetics besed on Clustering Algorithm." International Journal of Computer Sciences and Engineering 8.11 (2020): 72-78.
APA Style Citation: K. Gandhimathi, N. Umadevi, (2020). Prediction of Type 2 Diabetics besed on Clustering Algorithm. International Journal of Computer Sciences and Engineering, 8(11), 72-78.
BibTex Style Citation:
@article{Gandhimathi_2020,
author = {K. Gandhimathi, N. Umadevi},
title = {Prediction of Type 2 Diabetics besed on Clustering Algorithm},
journal = {International Journal of Computer Sciences and Engineering},
issue_date = {11 2020},
volume = {8},
Issue = {11},
month = {11},
year = {2020},
issn = {2347-2693},
pages = {72-78},
url = {https://www.ijcseonline.org/full_paper_view.php?paper_id=5266},
doi = {https://doi.org/10.26438/ijcse/v8i11.7278}
publisher = {IJCSE, Indore, INDIA},
}
RIS Style Citation:
TY - JOUR
DO = {https://doi.org/10.26438/ijcse/v8i11.7278}
UR - https://www.ijcseonline.org/full_paper_view.php?paper_id=5266
TI - Prediction of Type 2 Diabetics besed on Clustering Algorithm
T2 - International Journal of Computer Sciences and Engineering
AU - K. Gandhimathi, N. Umadevi
PY - 2020
DA - 2020/11/30
PB - IJCSE, Indore, INDIA
SP - 72-78
IS - 11
VL - 8
SN - 2347-2693
ER -
![]() |
![]() |
![]() |
219 | 308 downloads | 138 downloads |
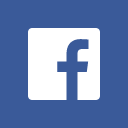
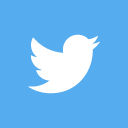
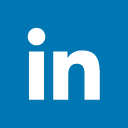
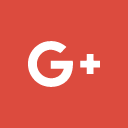
Abstract
Diabetes is one of the disease increases in humanity across the world-wide. Data mining is a process of extracting the information from a large dataset and transforms it into understandable structure. Medical data mining has been a great capability for finding hidden patterns from the large data sets of the medical dataset. The Data mining techniques used for the prediction of diseases like heart diseases, cancer, kidney stones, EEG etc. Prediction of Diabetes is an emerging and fastest growing technology in the medical analysis data. This research paper concentrates on the clustering method for grouping diabetic data based on cluster head attributes. In this paper the popular clustering algorithms K-Means, Fuzzy C-Means (FCM) and Gaussian Kernel based Fuzzy C-Means (GKFCM) clustering algorithms are selected and analyzed based on their fundamentals by using diabetes dataset. The algorithms performance is tested based on its various analyses. The results are compared with three algorithm algorithms. Finally we obtained that the GKFCM has best than K-means and FCM algorithm.
Key-Words / Index Term
K-means, Fuzzy C-Means (FCM), Gaussian Kernel based Fuzzy C-Means (GKFCM), Clustering, and Diabetes dataset.
References
[1]. Han J, Kamber M. Data Mining: Concepts and Techniques [J]. Data Mining Concepts Models Methods & Algorithms Second Edition, 2012.
[2]. Sumathi S, Sivanandam S N. Introduction to Data Mining and its Applications [J]. Studies in Computational Intelligence, 2006.
[3]. Hasim N, Haris N A. A study of open-source data mining tools for forecasting[C]// International Conference on Ubiquitous Information Management and Communication. ACM, 2015.
[4]. Norul Hidayah Ibrahim1 , Aida Mustapha2 , Rozilah Rosli3 , Nurdhiya Hazwani Helmee4 “A Hybrid Model of Hierarchical Clustering and Decision Tree for Rule-based Classification of Diabetic Patients” International Journal of Engineering and Technology (IJET) 2013.
[5]. Yihong Donga, Yueting Zhuanga, Ken Chenc, Xiaoying Taib, “A hierarchical clustering algorithm based on fuzzy graph connectedness”, Fuzzy Sets and Systems, Vol. 157 (13), pp. 1760– 1774.
[6]. P. Padmaja, V. Srikanth, N. Siddiqui, D. Praveen, B. Ambica, V.B.V.E. Venkata Rao, and V.J.P.Raju Rudraraju, “Characteristic evaluation of diabetes data using clustering techniques”International Journal of Computer Science and Network Security, Vol. 8 (11), pp. 244, 251, 2008.
[7]. Ashish Ghosh, Anindya Halder, Megha Kothari, and Susmita Ghosh, “Aggregation pheromone density based data clustering”, Information Sciences, Vol. 178 (13), pp.2816 –2831, 2008.
[8]. Adil M. Bagirow, “Modified global k- Means algorithm for minimum sum-of-squares clustering problems”, Pattern Recognition, Vol. 41 (10), pp. 3192–3199, 2008.
[9]. R.Nithya, P.Manikandan, and D.Ramyachitra, ”Analysis of clustering technique for the diabetes dataset using the training set parameter”, International Journal of Advanced Research in Computer and Communication Engineering, Vol. 4 (9), pp. 166–169, 2015.
[10]. Zeynel Cebeci and Figen Yildiz, “Comparison of K-Means and Fuzzy C-Means Algorithms on Different Cluster Structures”, Journal of Agricultural Informatics, Vol. 6 (3), pp. 13–23, 2015.
[11]. Usha G Biradar and Deepa S Mugali, “Clustering Algorithms on Diabetes Data:Comparative Case Study”, International Journal of Advanced Research in Computer Science,Vol.8(5), pp.550–552, 2017.
[12]. Jianpeng Qi, Yanwei Yu, Lihong Wang, Jinglei Liu and Yingjie Wang “An effective and efficient hierarchical k-Means clustering algorithm”, International Journal of Distributed Sensor Networks, Vol. 13 (8), pp.1–17, 2017.
[13]. K. Saravananathan and T. Velmurugan: Cluster based performance analysis for Diabetic data, International Journal of Pure and Applied Mathematics, 2018.
[14]. Karim M. Orabi1 , Yasser M. Kamal, and Thanaa M. Rabah. Early Predictive System for Diabetes Mellitus Disease. ICDM 2016, LNAI 9728, pp. 420– 427, 2016.
[15]. B.M. Patil, Hybrid prediction model for Type-2 diabetic patients. Expert Systems with Applications 37, 8102–8108, 2010.
[16]. Qinpei Zhao, Mantao Xu, and Pasi Fränti “Sum-of-Squares Based Cluster Validity Index and Significance Analysis* “
[17]. Bahman Bahmani, Benjamin Moseley, Andrea Vattani, “Scalable K Means++“ ellipses, IEEE Trans. Neural Networks 3 (5) 643–662, 1992.
[18]. Asha Gowda Karegowda , M.A. Jayaram, A.S. Manjunath, Cascading K-means Clustering and K-Nearest Neighbor Classifier for Categorization of Diabetic Patients.
[19]. WeinaWang, Yunjie Zhang, “On k means cluster validity indices”.