Performance of Machine Learning Techniques in the Prevention of Financial Frauds
Saleha Farheen1 , Monika Raghuwanshi2
Section:Research Paper, Product Type: Journal Paper
Volume-9 ,
Issue-1 , Page no. 27-29, Jan-2021
CrossRef-DOI: https://doi.org/10.26438/ijcse/v9i1.2729
Online published on Jan 31, 2021
Copyright © Saleha Farheen, Monika Raghuwanshi . This is an open access article distributed under the Creative Commons Attribution License, which permits unrestricted use, distribution, and reproduction in any medium, provided the original work is properly cited.
View this paper at Google Scholar | DPI Digital Library
How to Cite this Paper
- IEEE Citation
- MLA Citation
- APA Citation
- BibTex Citation
- RIS Citation
IEEE Style Citation: Saleha Farheen, Monika Raghuwanshi, “Performance of Machine Learning Techniques in the Prevention of Financial Frauds,” International Journal of Computer Sciences and Engineering, Vol.9, Issue.1, pp.27-29, 2021.
MLA Style Citation: Saleha Farheen, Monika Raghuwanshi "Performance of Machine Learning Techniques in the Prevention of Financial Frauds." International Journal of Computer Sciences and Engineering 9.1 (2021): 27-29.
APA Style Citation: Saleha Farheen, Monika Raghuwanshi, (2021). Performance of Machine Learning Techniques in the Prevention of Financial Frauds. International Journal of Computer Sciences and Engineering, 9(1), 27-29.
BibTex Style Citation:
@article{Farheen_2021,
author = {Saleha Farheen, Monika Raghuwanshi},
title = {Performance of Machine Learning Techniques in the Prevention of Financial Frauds},
journal = {International Journal of Computer Sciences and Engineering},
issue_date = {1 2021},
volume = {9},
Issue = {1},
month = {1},
year = {2021},
issn = {2347-2693},
pages = {27-29},
url = {https://www.ijcseonline.org/full_paper_view.php?paper_id=5290},
doi = {https://doi.org/10.26438/ijcse/v9i1.2729}
publisher = {IJCSE, Indore, INDIA},
}
RIS Style Citation:
TY - JOUR
DO = {https://doi.org/10.26438/ijcse/v9i1.2729}
UR - https://www.ijcseonline.org/full_paper_view.php?paper_id=5290
TI - Performance of Machine Learning Techniques in the Prevention of Financial Frauds
T2 - International Journal of Computer Sciences and Engineering
AU - Saleha Farheen, Monika Raghuwanshi
PY - 2021
DA - 2021/01/31
PB - IJCSE, Indore, INDIA
SP - 27-29
IS - 1
VL - 9
SN - 2347-2693
ER -
![]() |
![]() |
![]() |
525 | 464 downloads | 213 downloads |
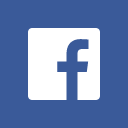
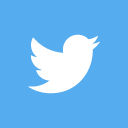
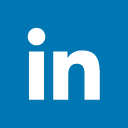
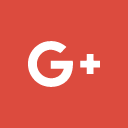
Abstract
Financial fraud presents more and more threat that has serious consequences in the financial sector. As a result, financial institutions are forced to continually improve their fraud detection systems. In recent years, several studies have used machine learning and data mining techniques to provide solutions to this problem. In this paper, we propose a state of art on various fraud techniques, as well as detection and prevention techniques proposed in the literature such as classification, clustering, And regression. The aim of this study is to identify the techniques and methods that give the best results that have been perfected so far. Financial fraud presents more and more threat that has serious consequences in the financial sector. As a result, financial institutions are forced to continually improve their fraud detection systems. In recent years, several studies have used machine learning and data mining techniques to provide solutions to this problem. In this paper, we propose a state of art on various fraud techniques, as well as detection and prevention techniques proposed in the literature such as classification, clustering, and regression. The aim of this study is to identify the techniques and methods that give the best results that have been perfected so far.
Key-Words / Index Term
Financial fraud, clustering, regression, machine learning
References
[1] Bolton, R. & Hand, D.. Statistical Fraud Detection: A Review (With Discussion). Statistical Science 17(3): 235–255.
[2] G.K. Palshikar, The Hidden Truth – Frauds and Their Control: A Critical Application for Business Intelligence, Intelligent Enterprise, vol. 5, no. 9, 28, pp. 46–51, May 2002.
[3] Mark. "Forensic Analytics: Methods and Techniques for Forensic Accounting Investigations". Hoboken, NJ: John Wiley & Sons Inc. ISBN 978-0-47089046-2.
[4] Pang-Ning Tan, Micheal Steinbach, Vipin Kumar, “Introduction to Data Mining”, Pearson Education, ISBN: 81-317-1472-1, 2006.
[5] Heyer, L.J., Kruglyak, S. and Yooseph, S., “Exploring Expression Data: Identification and Analysis of Coexpressed Genes”, Genome Research 9: pp:1106-1115, 1999.
[6] Ayse Yasemin SEYDIM “Intelligent Agents: A Data Mining Perspective” Southern Methodist University, Dallas, 1999
[7] T.Dean, J.Allen, Y.Aloimonos, “Artificial Intelligence: heory and Practice”, The Benjamin/Cummings Publishing Co. Inc., 1995.
[8] Eleni Mangina, “Intelligent Agent-Based Monitoring Platform for Applications in Engineerings”, International Journal of Computer Science & applications Vol.2, No.1, pp. 38-48, 2005.
[9] L. Yang, R. Karim, V. Ganapathy, and R. Smith, “Improving NFA-based signature matching using ordered binary decision diagrams,” in Proc.13th Int. Symp. Recent Adv. Intrusion Detect., pp. 58–78, Sep. 2010.
[10] A. Z. Broder, “Identifying and filtering near-duplicate documents,” in Proc. 11th Annu. Symp. Combinat. Pattern Matching, pp. 1–10, 2000.