Multi-Model Analysis of Mammograms
Vijaylaxmi K. Kochari1
Section:Research Paper, Product Type: Journal Paper
Volume-9 ,
Issue-1 , Page no. 30-35, Jan-2021
CrossRef-DOI: https://doi.org/10.26438/ijcse/v9i1.3035
Online published on Jan 31, 2021
Copyright © Vijaylaxmi K. Kochari . This is an open access article distributed under the Creative Commons Attribution License, which permits unrestricted use, distribution, and reproduction in any medium, provided the original work is properly cited.
View this paper at Google Scholar | DPI Digital Library
How to Cite this Paper
- IEEE Citation
- MLA Citation
- APA Citation
- BibTex Citation
- RIS Citation
IEEE Style Citation: Vijaylaxmi K. Kochari, “Multi-Model Analysis of Mammograms,” International Journal of Computer Sciences and Engineering, Vol.9, Issue.1, pp.30-35, 2021.
MLA Style Citation: Vijaylaxmi K. Kochari "Multi-Model Analysis of Mammograms." International Journal of Computer Sciences and Engineering 9.1 (2021): 30-35.
APA Style Citation: Vijaylaxmi K. Kochari, (2021). Multi-Model Analysis of Mammograms. International Journal of Computer Sciences and Engineering, 9(1), 30-35.
BibTex Style Citation:
@article{Kochari_2021,
author = {Vijaylaxmi K. Kochari},
title = {Multi-Model Analysis of Mammograms},
journal = {International Journal of Computer Sciences and Engineering},
issue_date = {1 2021},
volume = {9},
Issue = {1},
month = {1},
year = {2021},
issn = {2347-2693},
pages = {30-35},
url = {https://www.ijcseonline.org/full_paper_view.php?paper_id=5291},
doi = {https://doi.org/10.26438/ijcse/v9i1.3035}
publisher = {IJCSE, Indore, INDIA},
}
RIS Style Citation:
TY - JOUR
DO = {https://doi.org/10.26438/ijcse/v9i1.3035}
UR - https://www.ijcseonline.org/full_paper_view.php?paper_id=5291
TI - Multi-Model Analysis of Mammograms
T2 - International Journal of Computer Sciences and Engineering
AU - Vijaylaxmi K. Kochari
PY - 2021
DA - 2021/01/31
PB - IJCSE, Indore, INDIA
SP - 30-35
IS - 1
VL - 9
SN - 2347-2693
ER -
![]() |
![]() |
![]() |
497 | 454 downloads | 208 downloads |
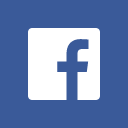
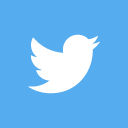
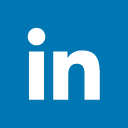
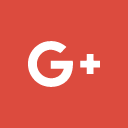
Abstract
In this paper mammogram classification is introduced. The system takes mammogram, pre-processes it by applying Adaptive Histogram Equalization. The enhanced image is segmented using K Means Clustering algorithm. Statistical features such as standard deviation and mean of a segmented mammogram are extracted. SVM takes these features as input. DCT is applied on the segmented mammogram, these extracted features are fed as input to FFBPNN. These classify the mammogram as normal or abnormal. The training time of both the classifiers are compared to know which classifier takes less training time. The accuracy of the classifiers are determined by analyzing the results.
Key-Words / Index Term
Mammograms, pre-process, SVM, FFBPNN
References
[1] S. Shanthi, V. Murali Bhaskaran, “Computer aided detection and classification of mammogram using self-adaptive resource allocation network classifier”, Proceedings of the International Conference on Pattern Recognition, Informatics and Medical Engineering , March 21-23, 2012
[2] Vijaylaxmi Kochari “Feed Forward Back Propagation Neural Network for Detection of Breast Cancer” International Journal of Computer Science Trends and Technology (IJCST) – Volume 6 Issue 6, Nov-Dec 2018, ISSN : 2347-8578.
[3] K Menaka, S Karpagavalli , “Mammogram Classification using Extreme Learning Machine and Genetic Programming,” International Conference on Computer Communication and Informatics (ICCCI -2014), Jan. 03 – 05, 2014
[4] Digambar A Kulkarni, Vijaylaxmi K Kochari “Detection of Breast Cancer Using K Means Algorithm” International Journal of Emerging Technology and Advanced Engineering Website: www.ijetae.com (ISSN 2250-2459, ISO 9001:2008 Certified Journal, Volume 6, Issue 4, April 2016.
[5] S .Bhadra “Analysis of Tumor Detection Methods for Mammogram Images” International Journal Of Computer Science and Engineering Vol.- 7, Issue- 5, May 2019 E-ISSN : 2347-2693
[6] M Arfan Jaffar, Nawazish Naveed, Sultan Zia, Bilal Ahmed and Tae-Sun Choi, “DCT Features Based Malignancy and Abnormality Type Detection Method For Mammograms” International Journal of Innovative Computing, Information and Control Volume 7, No.9, September 2011.
[7] Leonardo de Oliveira Martins, Geraldo Braz Junior, Aristofanes Correa Silva,Anselmo Cardoso de Paiva, and Marcelo Gattass_, “Detection of Masses in Digital Mammograms using K-means and Support Vector Machine”, Electronic Letters on Computer Vision and Image Analysis 8(2):39-50, 2009