MSVM Based Technique Used To Detect Diabetic Retinopathy at Early Stage
Kaveri Devi1 , Arshdeep Kaur2
Section:Survey Paper, Product Type: Journal Paper
Volume-9 ,
Issue-3 , Page no. 34-40, Mar-2021
CrossRef-DOI: https://doi.org/10.26438/ijcse/v9i3.3440
Online published on Mar 31, 2021
Copyright © Kaveri Devi, Arshdeep Kaur . This is an open access article distributed under the Creative Commons Attribution License, which permits unrestricted use, distribution, and reproduction in any medium, provided the original work is properly cited.
View this paper at Google Scholar | DPI Digital Library
How to Cite this Paper
- IEEE Citation
- MLA Citation
- APA Citation
- BibTex Citation
- RIS Citation
IEEE Style Citation: Kaveri Devi, Arshdeep Kaur, “MSVM Based Technique Used To Detect Diabetic Retinopathy at Early Stage,” International Journal of Computer Sciences and Engineering, Vol.9, Issue.3, pp.34-40, 2021.
MLA Style Citation: Kaveri Devi, Arshdeep Kaur "MSVM Based Technique Used To Detect Diabetic Retinopathy at Early Stage." International Journal of Computer Sciences and Engineering 9.3 (2021): 34-40.
APA Style Citation: Kaveri Devi, Arshdeep Kaur, (2021). MSVM Based Technique Used To Detect Diabetic Retinopathy at Early Stage. International Journal of Computer Sciences and Engineering, 9(3), 34-40.
BibTex Style Citation:
@article{Devi_2021,
author = {Kaveri Devi, Arshdeep Kaur},
title = {MSVM Based Technique Used To Detect Diabetic Retinopathy at Early Stage},
journal = {International Journal of Computer Sciences and Engineering},
issue_date = {3 2021},
volume = {9},
Issue = {3},
month = {3},
year = {2021},
issn = {2347-2693},
pages = {34-40},
url = {https://www.ijcseonline.org/full_paper_view.php?paper_id=5314},
doi = {https://doi.org/10.26438/ijcse/v9i3.3440}
publisher = {IJCSE, Indore, INDIA},
}
RIS Style Citation:
TY - JOUR
DO = {https://doi.org/10.26438/ijcse/v9i3.3440}
UR - https://www.ijcseonline.org/full_paper_view.php?paper_id=5314
TI - MSVM Based Technique Used To Detect Diabetic Retinopathy at Early Stage
T2 - International Journal of Computer Sciences and Engineering
AU - Kaveri Devi, Arshdeep Kaur
PY - 2021
DA - 2021/03/31
PB - IJCSE, Indore, INDIA
SP - 34-40
IS - 3
VL - 9
SN - 2347-2693
ER -
![]() |
![]() |
![]() |
398 | 377 downloads | 157 downloads |
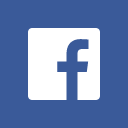
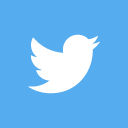
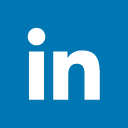
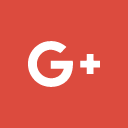
Abstract
Diabetic retinopathy causes the life of eye decay considerably. There are stages associated with the DR. Early detection of DR could lead to the adverse affect of DR to be minimised. Techniques have been devised to tackle and identify the problems of DR at early stage. This paper presents the comprehensive review of techniques such as machine learning and deep learning, used for the purpose of detection of DR and also performs the comparative analysis of parameters used for the same. The proposed algorithm uses MSVM algorithm that discovers more patterns to detect disease accurately. The results will help in predicting quicker and more accurate disease so that it lead timely treatment of the patients.
Key-Words / Index Term
Diabetic retinopathy, machine learning, deep learning, datasets
References
[1] P. Adarsh and D. Jeyakumari, “Multiclass svm-based automated diagnosis of diabetic retinopathy,” Int. Conf. Commun. Signal Process., pp. 206–210, 2013.
[2] R. Maher and M. Dhopeshwarkar, “Automatic detection Non-proliferative Diabetic Retinopathy using image processing techniques,” J. Eng. Res. Appl., vol. 6, no. 1, pp. 122–127, 2016.
[3] R. Gargeya and T. Leng, “Automated Identi fi cation of Diabetic Retinopathy Using Deep Learning,” Ophthalmology, pp. 1–8, 2017.
[4] S. M. Student, C. Landran, and G. Kaur, “Review on: Detection of Diabetic Retinopathy using SVM and MDA,” Int. J. Comput. Appl., vol. 117, no. 19, pp. 975–8887, 2015.
[5] I. Ntroduction, “Diabetic Retinopathy Classification using SVM Classifier,” ACM Comput. Surv., vol. 6, no. 7, pp. 7–11, 2017.
[6] S. Shetty, K. B. Kari, and J. A. Rathod, “Detection of Diabetic Retinopathy Using Support Vector Machine ( SVM ),” IEEE 17th Int. Conf. Parallel Distrib. Syst., vol. 23, no. 6, pp. 207–211, 2016.
[7] M. J. Paranjpe and P. M. N. Kakatkar, “Automated Diabetic Retinopathy Severity Classification using Support Vector Machine,” Int. J. Res. Sci. Technol., no. 3, pp. 86–91, 2013.
[8] V. V. Kumari, N. Suriyaharayananm, and C. T. Saranya, “Feature Extraction for Early Detection of Diabetic Retinopathy,” in 2010 International Conference on Recent Trends in Information, Telecommunication and Computing, pp. 359–361, 2010.
[9] V. Ramya, “SVM Based Detection for Diabetic Retinopathy,” Iccad, vol. V, no. I, pp. 11–13, 2018.
[10] R. Gargeya and T. Leng, “Automated Identi fi cation of Diabetic Retinopathy Using Deep Learning,” Ophthalmology, pp. 1–8, 2017.
[11] M. S. Haleem, L. Han, J. Van Hemert, B. Li, and A. Fleming, “Retinal Area Detector from Scanning Laser Ophthalmoscope ( SLO ) Images for Diagnosing Retinal Diseases,” vol. 2194, no. MARCH, 2014.
[12] S. S. Rubini and A. Kunthavai, “Diabetic retinopathy detection based on eigenvalues of the hessian matrix,” Procedia Comput. Sci., vol. 47, no. C, pp. 311–318, 2014.
[13] S. M. Student, C. Landran, and G. Kaur, “Review on: Detection of Diabetic Retinopathy using SVM and MDA,” Int. J. Comput. Appl., vol. 117, no. 19, pp. 975–8887, 2015.
[14] V. Ramya, “SVM Based Detection for Diabetic Retinopathy,” IEEE, vol. V, no. I, pp. 11–13, 2018.
[15] A. Mukhlas and A. Ahmad, “Data Mining Technique?: Towards Supporting Local Co-operative Society in Customer Profiling , Market Analysis and Prototype Construction,” no. May, pp. 109–114, 2016.
[16] R. D. Q. D. Lqglu, D. Lv, D. Frqvlghuhg, I. R. U. Wkh, D. Dqdo, S. Lq, W. K. H. Olwhudwxuh, V. D. U. Pdq, and G. Lq, “A Review of Data Mining and Solar Power Prediction,” vol. 0, pp. 3–7, 2016.
[17] M. Bakon, I. Oliveira, D. Perissin, J. J. Sousa, and J. Papco, “A Data Mining Approach for Multivariate Outlier Detection in Postprocessing of Multitemporal InSAR Results,” vol. 1, pp. 1–8, 2017.
[18] E. E. Brown, “Improving Privacy Preserving Methods to Enhance Data Mining for Correlation Research,” pp. 3–6, 2017.
[19] K. Ahuja, “Data Elimination Based Technique for Mining Frequent Closed Item Set,” pp. 1–4.