A New Uncertainty Measure and Application to Image Processing
Sheeja T.K.1 , Sunny Kuriakose A.2
Section:Research Paper, Product Type: Journal Paper
Volume-9 ,
Issue-4 , Page no. 20-24, Apr-2021
CrossRef-DOI: https://doi.org/10.26438/ijcse/v9i4.2024
Online published on Apr 30, 2021
Copyright © Sheeja T.K., Sunny Kuriakose A. . This is an open access article distributed under the Creative Commons Attribution License, which permits unrestricted use, distribution, and reproduction in any medium, provided the original work is properly cited.
View this paper at Google Scholar | DPI Digital Library
How to Cite this Paper
- IEEE Citation
- MLA Citation
- APA Citation
- BibTex Citation
- RIS Citation
IEEE Style Citation: Sheeja T.K., Sunny Kuriakose A., “A New Uncertainty Measure and Application to Image Processing,” International Journal of Computer Sciences and Engineering, Vol.9, Issue.4, pp.20-24, 2021.
MLA Style Citation: Sheeja T.K., Sunny Kuriakose A. "A New Uncertainty Measure and Application to Image Processing." International Journal of Computer Sciences and Engineering 9.4 (2021): 20-24.
APA Style Citation: Sheeja T.K., Sunny Kuriakose A., (2021). A New Uncertainty Measure and Application to Image Processing. International Journal of Computer Sciences and Engineering, 9(4), 20-24.
BibTex Style Citation:
@article{T.K._2021,
author = {Sheeja T.K., Sunny Kuriakose A.},
title = {A New Uncertainty Measure and Application to Image Processing},
journal = {International Journal of Computer Sciences and Engineering},
issue_date = {4 2021},
volume = {9},
Issue = {4},
month = {4},
year = {2021},
issn = {2347-2693},
pages = {20-24},
url = {https://www.ijcseonline.org/full_paper_view.php?paper_id=5320},
doi = {https://doi.org/10.26438/ijcse/v9i4.2024}
publisher = {IJCSE, Indore, INDIA},
}
RIS Style Citation:
TY - JOUR
DO = {https://doi.org/10.26438/ijcse/v9i4.2024}
UR - https://www.ijcseonline.org/full_paper_view.php?paper_id=5320
TI - A New Uncertainty Measure and Application to Image Processing
T2 - International Journal of Computer Sciences and Engineering
AU - Sheeja T.K., Sunny Kuriakose A.
PY - 2021
DA - 2021/04/30
PB - IJCSE, Indore, INDIA
SP - 20-24
IS - 4
VL - 9
SN - 2347-2693
ER -
![]() |
![]() |
![]() |
422 | 362 downloads | 159 downloads |
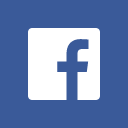
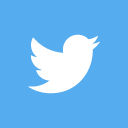
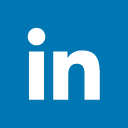
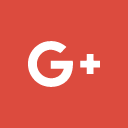
Abstract
Uncertainty measures form essential constituents of information theory as they provide a sufficient mechanism for determining the quantity of useful information contained in a system. In the present work, the concept of divergence between fuzzy sets are made use of in defining new measures of uncertainty in the framework of fuzzy rough sets. Further, these measures are utilized in developing an algorithm for binary image segmentation of a grey level image. Moreover, the proposed algorithm is implemented using different test images with the help of an OCTAVE program.
Key-Words / Index Term
Divergence, Image segmentation, Fuzzy Set, Rough set, Uncertainty measure
References
[1] C. E. Shannon, “A Mathematical Theory of Communication", The Bell System Technical Journal Vol.27, pp. 379 – 423, 1948.
[2] Z. Pawlak, "Rough Sets", International Journal of Computer and Information Sciences, Vol.11, Issue.5, pp.341 - 356, 1982.
[3] L. A. Zadeh, "Fuzzy Sets", Information and Control, Vol.8, Issue.3, pp.338-353, 1965.
[4] A. Nakamura, “Fuzzy Rough Sets”, Note on Multiple-Valued Logic in Japan, Vol.3, pp.1-8, 1988.
[5] A. Radzikowska and E. E. Kerre, "A comparative Study of Fuzzy Rough Sets", Fuzzy Sets and Systems, Vol.126, pp.137-155, 2002.
[6] T. Beaubouef, F. E.. Petry and G. Arora, “Information Theoretic Measures of Uncertainty for Rough Sets and Rough Relational Databases”, Information Sciences, Vol.109, pp.185 – 195, 1998.
[7] J. Y. Liang, K. S. Chin, C. Y. Dang and R. C. M. Yam, "A New Method for Measuring Uncertainty and Fuzziness in Rough Set Theory", International Journal of General Systems Vol.31(4) pp.331- 342, 2002.
[8] J. Y. Liang, J. H. Wang and Y. H. Qian, “A New Measure of Uncertainty Based on Knowledge Granulation for Rough Sets”, Information Sciences Vol.179, pp. 458 – 470, 2009.
[9] J.S. Mi, X. M. Li, H. Y. Zhao and T. Feng, "Information Theoretic Measures of Uncertainty in Generalized Fuzzy Rough Sets", in A. An et al. (eds) RSFDGrC 2007, LNAI 4482, Springer pp. 63 – 70, 2007.
[10] N. Dhanachandra and Y. J. Chanu, "A survey on image segmentation methods using clustering techniques", European Journal of Engineering Research and Science, Vol.2(1), pp 15-20, 2017.
[11] E. E. Kerre and M. Nachtegael(Eds), “Fuzzy Techniques in Image Processing”, Sringer-Verlag, Heidelberg, 2000.
[12] A. E. Hassanien, A. Abraham, J. F. Peters, G. Schaefer, and C. Henry, "Rough sets and near sets in medical imaging: a review", IEEE Transactions on Information Technology in Biomedicine, Vol.13(6), pp.955-968, 2009.
[13] S. S. Begum, D. R. Lakshmi, “A Review of Current Methods in Medical Image Segmentation”, International Journal of Computer Sciences and Engineering, Vol.7(12), pp.67–73, 2019.
[14] N. Senthilkumaran and S. Vaithegi, "Image Segmentation by Using Thresholding Techniques for Medical Images", Computer Science and Engineering: An International Journal, Vol.6(1), pp.1-13, 2016.
[15] J. C. Bezdek, “Pattern recognition with fuzzy objective function algorithms” Plenum Press, New York, 2013.
[16] N. Otsu, "A Threshold Selection Algorithm from Gray Level Histograms", IEEE Transactions on Systems, Man and Cybernetics, Vol.9, pp.62 – 66, 1979.
[17] J. Klir and B. Yuan, “Fuzzy Sets and Fuzzy Logic”, Prentice Hall, New Jersey, 1995.
[18] S. Montes, I. Couso, P. Gil and C. Bertoluzza, "Divergence Measure Between Fuzzy Sets", International Journal of Approximate Reasoning, Vol.30, pp.91-105, 2002.
[19] Z. Pawlak, "Rough Sets - Theoretical Aspect of Reasoning About Data", Kluwer Academic Publishers, The Netherlands, 1991.
[20] D. Dubois and H. Prade, "Rough Fuzzy Sets and Fuzzy Rough Sets", International Journal of General Systems, Vol.17, pp.191-209, 1990.
[21] J. S. Mi, Y. Leung and T. Feng, "Generalized Fuzzy Rough Sets Determined by aTriangular Norm", Information Sciences, Vol.178, pp.3203-3213, 2008.
[22] S. Vluymans, L. D`eer, Y. Saeys and C. Cornelis, "Applications of Fuzzy Rough Set Theory in Machine Learning: a Survey", Fundamenta Informaticae, pp.1-34, 2015.
[23] W. Z. Wu, J. S. Mi and W. X. Zhang, "Generalized Fuzzy Rough Sets", Information Sciences, Vol.151, pp.263-282, 2003.
[24] Sheeja T. K. and A. Sunny Kuriakose, "Divergence Based Generalized Fuzzy Rough Sets", International Journal of Computer Sciences and Engineering, Vol.6(6), pp.809 – 815, 2018.
[25] M. J. Wierman, “Measuring Uncertainty in Rough Set Theory”, International Journal of General System Vol.28, pp.283 – 297, 1999.
[26] J. Y. Zhao, Z. Zhang, C. Han, and Z. Zhou, "Complement information entropy for uncertainty measure in fuzzy rough set and its applications", Soft Computing, Vol.19 (7), pp.1997– 2010, 2015.