Uncertainty Handling In Big Data Analytics: Survey, Opportunities and Challenges
Priya Nagargoje1 , Monali Baviskar2
Section:Survey Paper, Product Type: Journal Paper
Volume-9 ,
Issue-6 , Page no. 59-63, Jun-2021
CrossRef-DOI: https://doi.org/10.26438/ijcse/v9i6.5963
Online published on Jun 30, 2021
Copyright © Priya Nagargoje, Monali Baviskar . This is an open access article distributed under the Creative Commons Attribution License, which permits unrestricted use, distribution, and reproduction in any medium, provided the original work is properly cited.
View this paper at Google Scholar | DPI Digital Library
How to Cite this Paper
- IEEE Citation
- MLA Citation
- APA Citation
- BibTex Citation
- RIS Citation
IEEE Style Citation: Priya Nagargoje, Monali Baviskar, “Uncertainty Handling In Big Data Analytics: Survey, Opportunities and Challenges,” International Journal of Computer Sciences and Engineering, Vol.9, Issue.6, pp.59-63, 2021.
MLA Style Citation: Priya Nagargoje, Monali Baviskar "Uncertainty Handling In Big Data Analytics: Survey, Opportunities and Challenges." International Journal of Computer Sciences and Engineering 9.6 (2021): 59-63.
APA Style Citation: Priya Nagargoje, Monali Baviskar, (2021). Uncertainty Handling In Big Data Analytics: Survey, Opportunities and Challenges. International Journal of Computer Sciences and Engineering, 9(6), 59-63.
BibTex Style Citation:
@article{Nagargoje_2021,
author = {Priya Nagargoje, Monali Baviskar},
title = {Uncertainty Handling In Big Data Analytics: Survey, Opportunities and Challenges},
journal = {International Journal of Computer Sciences and Engineering},
issue_date = {6 2021},
volume = {9},
Issue = {6},
month = {6},
year = {2021},
issn = {2347-2693},
pages = {59-63},
url = {https://www.ijcseonline.org/full_paper_view.php?paper_id=5349},
doi = {https://doi.org/10.26438/ijcse/v9i6.5963}
publisher = {IJCSE, Indore, INDIA},
}
RIS Style Citation:
TY - JOUR
DO = {https://doi.org/10.26438/ijcse/v9i6.5963}
UR - https://www.ijcseonline.org/full_paper_view.php?paper_id=5349
TI - Uncertainty Handling In Big Data Analytics: Survey, Opportunities and Challenges
T2 - International Journal of Computer Sciences and Engineering
AU - Priya Nagargoje, Monali Baviskar
PY - 2021
DA - 2021/06/30
PB - IJCSE, Indore, INDIA
SP - 59-63
IS - 6
VL - 9
SN - 2347-2693
ER -
![]() |
![]() |
![]() |
265 | 382 downloads | 163 downloads |
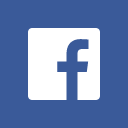
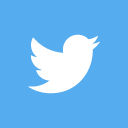
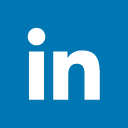
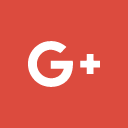
Abstract
Big Data analysis and processing is a popular tool for Artificial Intelligence and Data Science to extract applicable solution from data across a broad range of application domains. Even though Big data is in the mainstream of operations as of 2020, With the increase in data processing and storage capacity, a large amount of data is available and because of that potential issues or challenges the researchers can address, some of these issues overlap with the data science field. One of the key issue is the inevitable existence of uncertainty in stored or missing values. Any uncertainty in a source causes its disadvantageous, complexity or inapplicability to use. It is importance to ensure the reliability and a value of data source. That is why it is crucial to eliminate uncertainty or to lower uncertainty influence because data without any analysis does not have much value. In this paper we review previous work in big data analytics and Survey of many theories and techniques which have been developed to model its various forms. We have described several common techniques such as Bayesian model and fuzzy set, Shannon’s entropy. We present a discussion of open challenges and future directions for handling and eliminating uncertainty in this profile.
Key-Words / Index Term
Big Data, Data Sciences, Data Uncertainty, Uncertainty Elimination, Machine learning, NLP, Computational Intelligence.
References
[1] Andrii Berko,Vladyslav Alieksieiev, “A Method to Solve Uncertainty Problem for Big Data Sources, IEEE Second International Conference on Data Stream Mining & Processing, August 21-25, 2018, Lviv, Ukraine.
[2] Scott Bruce, , Zeda Li, Hsiang-Chieh Yang, Subhadeep Mukhopadhyay ” Nonparametric Distributed Learning Architecture for Big Data: Algorithm and Applications” IEEE TRANSACTIONS ON BIG DATA, NOVEMBER 2017.
[3] K. Sree Divya, P.Bhargavi, S. Jyothi,” Machine Learning Algorithms in Big data Analytics” INTERNATIONAL JOURNAL OF COMPUTER SCIENCES AND ENGINEERING • January 2018.
[4] Reihaneh H. Hariri, Erik M. Fredericks and Kate M. Bowers,” Uncertainty in big data analytics: survey, opportunities, and challenges” Hariri et al. J Big Data Springer open source journal 2019.
[5] Athmaja S, Hanumanthappa M, Vasantha Kavitha,” A SURVEY OF MACHINE LEARNING ALGORITHMS FOR BIG DATA ANALYTICS” IEEE International Conference on Innovations in Information, Embedded and Communication Systems 2017.
[6] M. U. Bokhari, M. Zeyauddin and M. A. Siddiqui, “An effective model for big data analytics”, 3rd International Conference on Computing for Sustainable Global Development, pp. 3980-3982, 2016.
[7] Monali R. Baviskar, Priya N Nagargoje, Priyanka A. Deshmukh, Rina R. Baviskar, “A Survey of Data Science Techniques and Available Tools”, International Research Journal of Engineering and Technology (IRJET) e-ISSN: 2395-0056 Volume: 08 Issue: 04 | Apr 2021 p-ISSN: 2395-0072
[8] R. Farias and E. Clua,” Parallel Image Segmentation using Reduction-Sweeps on Multi-core Processors and GPUs IEEE” 26th Conference on Graphics, Patterns and Images (SIBGRAPI), Rio de Janeiro, Brazil.
[6] Z. Yang, Y. Zhu, and Y. Pu, Parallel image processing based on CUDA, In Proceedings of the 2008 ACM International Conference on Computer Science and Software Engineering - Volume 03 (CSSE ’08) , 2008, Vol. 3, pp. 198-201.
[7] J. L. Berral-Garcia, “A quick view on current techniques and machine learning algorithms for big data analytics”, 18th International Conf. on Transparent Optical Networks, pp.1-4.
2016. DOI: 10.1109/ICTON.2016.7550517.
[8] B. Kulis and M. I. Jordan A New k-Means algorithms via Bayesian Non parametrics Proceedings of the 29th International Conference on Machine Learning, Edinburgh, Scotland, UK, 2012.
[9] N. Marz , and J. Warren, Big Data: Principles and best practices of scalable real time data systems. Manning Publications, 2015.
[10] J. Chen, D. Dosyn, V. Lytvyn, and A. Sachenko, “Smart Data Integration by Goal Driven Ontology Learning. Advances in Big Data,” Advances in Intelligent Systems and Computing, Springer.
[11] Saidulu D, Sasikala R. Machine learning and statistical approaches for Big Data: issues, challenges and research directions. Int J Appl Eng Res. 2017;12(21):11691–9.
[12] J. L. Berral-Garcia, “A quick view on current techniques and machine learning algorithms for big data analytics”, 18th International Conf. on Transparent Optical Networks, pp.1-4, 2016. DOI: 10.1109/ICTON.2016.7550517.
[13] Parmar, V. & Gupta, I., 2015. Big data analytics vs Data Mining analytics. IJITE, 3(3), pp.258–263.
[14] G. Cavallaro, M. Riedel, M. Richerzhagen, J. A. Benediktsson, and A. Plaza, "On Understanding Big Data Impacts in Remotely Sensed Image Classification Using Support Vector Machine Methods," IEEE Journal of Selected Topics in Applied Earth Observations and Remote Sensing, vol. 8, pp. 4634-4646, 2015.
[15] Joseph D. Prusa* andTaghi M. Khoshgoftaar, ”Improving deep neural network design with new text data representations” J Big Data (2017) 4:7DOI 10.1186/s40537-017-0065-8.
[16] Karl Weiss* , Taghi M. Khoshgoftaar and Ding Ding Wang Weiss,” A survey of transfer learning” et al. J Big Data (2016) 3:9 DOI 10.1186/s40537-016-0043-6.
[17] Priya nagargoje, vandna jagtap, “time-efficient fracture detection using multi-core parallel processing”, international journal of current engineering and scientific research (ijcesr) issn (print): 2393-8374, (online): 2394-0697, volume-3, issue-9, 2016.