Review of Chronic Inflammation and long term effects on health using Machine Learning Algorithms
Abeda Begum1 , Rajeev Kumar2
Section:Review Paper, Product Type: Journal Paper
Volume-9 ,
Issue-6 , Page no. 64-71, Jun-2021
CrossRef-DOI: https://doi.org/10.26438/ijcse/v9i6.6471
Online published on Jun 30, 2021
Copyright © Abeda Begum, Rajeev Kumar . This is an open access article distributed under the Creative Commons Attribution License, which permits unrestricted use, distribution, and reproduction in any medium, provided the original work is properly cited.
View this paper at Google Scholar | DPI Digital Library
How to Cite this Paper
- IEEE Citation
- MLA Citation
- APA Citation
- BibTex Citation
- RIS Citation
IEEE Style Citation: Abeda Begum, Rajeev Kumar, “Review of Chronic Inflammation and long term effects on health using Machine Learning Algorithms,” International Journal of Computer Sciences and Engineering, Vol.9, Issue.6, pp.64-71, 2021.
MLA Style Citation: Abeda Begum, Rajeev Kumar "Review of Chronic Inflammation and long term effects on health using Machine Learning Algorithms." International Journal of Computer Sciences and Engineering 9.6 (2021): 64-71.
APA Style Citation: Abeda Begum, Rajeev Kumar, (2021). Review of Chronic Inflammation and long term effects on health using Machine Learning Algorithms. International Journal of Computer Sciences and Engineering, 9(6), 64-71.
BibTex Style Citation:
@article{Begum_2021,
author = {Abeda Begum, Rajeev Kumar},
title = {Review of Chronic Inflammation and long term effects on health using Machine Learning Algorithms},
journal = {International Journal of Computer Sciences and Engineering},
issue_date = {6 2021},
volume = {9},
Issue = {6},
month = {6},
year = {2021},
issn = {2347-2693},
pages = {64-71},
url = {https://www.ijcseonline.org/full_paper_view.php?paper_id=5350},
doi = {https://doi.org/10.26438/ijcse/v9i6.6471}
publisher = {IJCSE, Indore, INDIA},
}
RIS Style Citation:
TY - JOUR
DO = {https://doi.org/10.26438/ijcse/v9i6.6471}
UR - https://www.ijcseonline.org/full_paper_view.php?paper_id=5350
TI - Review of Chronic Inflammation and long term effects on health using Machine Learning Algorithms
T2 - International Journal of Computer Sciences and Engineering
AU - Abeda Begum, Rajeev Kumar
PY - 2021
DA - 2021/06/30
PB - IJCSE, Indore, INDIA
SP - 64-71
IS - 6
VL - 9
SN - 2347-2693
ER -
![]() |
![]() |
![]() |
236 | 303 downloads | 155 downloads |
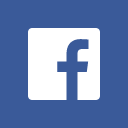
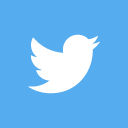
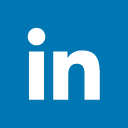
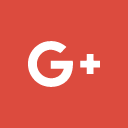
Abstract
The purpose of this review is to find how today`s generation impacted with chronic inflammation and its effects on health and long-term diseases using Machine Learning Algorithms. These diseases are found to be appeared after long time suffering with chronic inflammation. By detecting it early and taking lifestyle changes and healthy diet could potentially avoid the diseases like diabetes, cardiovascular diseases, cancer, arthritis, and bowel diseases. Autoimmune disease symptoms can be minimized by identifying inflammation levels and by taking precautionary measures at any stage of the patient. There are various inflammation markers to detect inflammation in the body by simple blood tests like CRP, ESR which are inexpensive and provide early disease detective mechanisms. These reports can be input to Machine Learning algorithms and train the system to help the patients to identify inflammation levels and alert them to take appropriate actions to prevent further damage from diseases on human organs.
Key-Words / Index Term
Machine Learning, algorithm, Inflammation, Autoimmune
References
[1]. A. Crimarco, et al., “Baseline Markers of Inflammation, Lipids, Glucose, and Dietary Inflammatory Index Scores Do Not Differ between Adults Willing to Participate in an Intensive Inflammation Reduction Intervention and Those Who Do Not.” Nutrition and Health, vol. 25, No. 1, pp. 9–19, 2019.
[2]. H. Clevers, “At the Crossroads of Inflammation and Cancer”, Cell Press, Vol. 118, pp. 671–674, 2004.
[3]. A. M. Minihane, “Low-grade inflammation, diet composition and health: current research evidence and its translation”, British Journal of Nutrition, Vol. 114, pp. 999–1012, 2015.
[4]. M.C. Faleiros et al., “Machine learning techniques for computer-aided classification of active inflammatory sacroiliitis in magnetic resonance imaging”, Advances in Rheumatology, Vol. 60, No. 25, pp. 1-3, 2020.
[5]. B. F. Erickson, P. Korfiatis, Z. Akkus, T.L. Kline, “Machine Learning for Medical Imaging", Radio Graphics, Vol. 37, No. 2, pp.505-515, 2017.
[6]. T. D. Maarseveen, “Machine Learning Electronic Health Record Identification of Patients with Rheumatoid Arthritis: Algorithm Pipeline Development and Validation Study”, JMIR Medical Informatics, Vol. 8, No. 11, pp. 1-3, 2020.
[7]. Z. Odibat, S. Momanib, H. Xuc, “A reliable algorithm of homotopy analysis method for solvingnonlinear fractional differential equations”, Elsevier Applied Mathematical Modelling, Vol. 34, pp. 593–600, 2010.
[8]. S. Jung, W. J. Yun, J. Kim, J. Kim, “Coordinated Multi-Agent Deep Reinforcement Learning for Energy-Aware UAV-Based Big-Data Platforms”, Electronics, Vol. 10, No. 543, pp. 1-5, 2021.
[9]. K. Pieszko et al., “Machine-learned models using hematological inflammation markers in the prediction of short-term acute coronary syndrome outcomes”, Journal of Translational Medicine, Vol. 16, No. 334, pp. 1-3, 2018.
[10]. N. S. S. Tabib et al., “Big data in IBD: big progress for clinical practice.”, Gut, Vol. 69, No. 8, pp. 1520-1532, 2020.
[11]. G. Chartrand et al., “Deep Learning: A Primer for Radiologists”. Radio Graphics, Vol. 37, pp.2113–2131, 2017.
[12]. R. Forlano et al., “High-Throughput, Machine Learning-Based Quantification of Steatosis, Inflammation, Ballooning, and Fibrosis in Biopsies from Patients with Nonalcoholic Fatty Liver Disease”, Clinical Gastroenterology and Hepatology, Vol. 18, No. 9, pp. 2081-2090, 2020.
[13]. L. Yu, J. He, L. Wang, H. Yi, “Inflammatory profiles revealed the dysregulation of cytokines in adult patients of HFMD”, International Journal of Infectious Diseases, Vol. 79, pp.12–20, 2019.
[14]. B. Kegerreis et al., “Machine learning approaches to predict lupus disease activity from gene expression data”. Scientific Reports, Vol. 9, No. 9617, pp. 1-3, 2019.
[15]. S. Le et al., “Supervised machine learning for the early prediction of acute respiratory distress syndrome (ARDS)”, Journal of Critical Care, Vol. 60, No. 102, pp. 1-3, 2020.
[16]. A. M. D. L. Villehuchet et al., “A machine-learning approach to the prediction of oxidative stress in chronic inflammatory disease”, Redox Report, Vol.14, No.1, pp. 23-33, 2009.
[17]. K. Kim, I. Tagkopoulos, “Application of machine learning in rheumatic disease research”, The Korean Journal of Internal Medicine, Vol. 34, No. 4, pp. 708–722, 2019.
[18]. I.S. Stafford et al., “A systematic review of the applications of artificial intelligence and machine learning in autoimmune diseases”. npj Digital Medicine Vol. 3, No. 30, pp.1-3, 2020.
[19]. B. Rush et al. “Applying machine learning to continuosly monitored physiological data.” Journal of clinical monitoring and computing, Vol. 33, No. 5, pp. 887–893, 2019.
[20]. P. K. Kondeti et al. “Applications of machine learning techniques to predict filariasis using socio-economic factors”, Epidemiology and Infection, Vol. 147, No. 260, pp.1-11, 2019.
[21]. A. Romagnoni et al., “Comparative performances of machine learning methods for classifying Crohn Disease patients using genome-wide genotyping data”, Scientific Reports, Vol. 9, No. 10351, pp. 1-3, 2019.
[22]. E. O. Dana et al., “Identification of Three Rheumatoid Arthritis Disease Subtypes by Machine Learning Integration of Synovial Histologic Features and RNA Sequencing Data”, Arthritis Rheumatol., Vol. 70, No. 5, pp. 690-701, 2018.
[23]. A. Awaysheh et al., “Evaluation of supervised machine-learning algorithms to distinguish between inflammatory bowel disease and alimentary lymphoma in cats.”, Journal of Veterinary Diagnostic Investigation, Vol. 28, No. 6, pp. 679-687, 2016.
[24]. I. R. Cohen, S. Efroni, “The Immune System Computes the State of the Body: Crowd Wisdom, Machine Learning, and Immune Cell Reference Repertoires Help Manage Inflammation”, Frontiers in immunology, Vol. 10, No. 10, pp. 1-3, 2019.
[25]. A. Aravind et al., “Machine Learning Technology for Evaluation of Liver Fibrosis, Inflammation Activity and Steatosis (LIVERFAStTM)”, Journal of Intelligent Learning Systems and Applications, Vol. 12, pp.31-49, 2020.
[26]. D. Panaretos et al., “A comparison of statistical and machine-learning techniques in evaluating the association between dietary patterns and 10-year cardiometabolic risk (2002–2012): the ATTICA study”, British Journal of Nutrition, Vol. 120, pp.326-334, 2018.
[27]. C. M. Lynch et al., “Prediction of lung cancer patient survival via supervised machine learning classification techniques”, Int J Med Inform, Vol. 108, pp. 1–8, 2017.
[28]. J. Neumann et al., “Chronic Inflammation Under the Microscope”, Microscopy Today, Vol. 24, No. 6, pp. 38–45, 2016.
[29]. E. Dean, R. G. Hansen, “Prescribing Optimal Nutrition and Physical Activity as “First-Line” Interventions for Best Practice Management of Chronic Low-Grade Inflammation Associated with Osteoarthritis: Evidence Synthesis”, Hindawi Publishing Corporation Arthritis, Vol. 2012, Article ID 560634, 2012.
[30]. B. CHASSAING, A. T. GEWIRTZ, “Gut Microbiota, Low-grade Inflammation, and Metabolic Syndrome”. Toxicologic Pathology, Vol. 42, pp. 49-53, 2014.
[31]. J. Lötsch et al., “Machine-learning based lipid mediato serum concentration patterns allow identification of multiple sclerosis patients with high accuracy”, Scientific Reports, Vol. 8, No. 14884, pp. 1-3, 2018.
[32]. A. Jaiswal, R. Kumar, “Review of Machine Learning Algorithm on Cancer Classification for Cancer Prediction and Detection”, The International journal of analytical and experimental modal analysis, Vol. XI, No. XII, pp. 1-3, 2019.
[33]. T. Panch, P. Szolovits, R. Atun, “Artificial intelligence, machine learning and health systems”, Journal of Global Health, Vol. 8, No. 2, 2018.
[34]. N. Perakakis, A. Yazdani, G. E. Karniadakis, C. Mantzoros, “Omics, big data and machine learning as tools to propel understanding of biological mechanisms and to discover novel diagnostics and therapeutics”, Metabolism, Vol. 87, pp. A1-A9, 2018.
[35]. J. Hu, H. Niu, J. Carrasco, B. Lennox, F. Arvin, “Voronoi-Based Multi-Robot Autonomous Exploration in Unknown Environments via Deep Reinforcement Learning”, IEEE Transactions on Vehicular Technology, Vol. 69, No. 12, pp. 14413-14423, 2020.
[36]. S. Huang et al., “Applications of Support Vector Machine (SVM) Learning in Cancer Genomics”, Cancer Genomics & Proteomics, Vol. 15, pp. 41-51, 2018.
[37]. J. Tolles, W. J. Meurer, “Logistic Regression: Relating Patient Characteristics to Outcomes”, JAMA Guide to Statistics and Methods, Vol. 316, No. 5, pp. 533-4, 2016.
[38]. Y. Li, H. G. Hong, Y. Li, “Multiclass Linear Discriminant Analysis with Ultrahigh-Dimensional Features”, Biometrics, Vol. 75, No. 4, pp. 1086–1097, 2019.
[39]. G. J. McLachlan, “Discriminant Analysis and Statistical Pattern Recognition”, A John Wiley & Sons Inc. Publication, Hoboken, New Jersey, 2004, ISBN 978-0-471-69115-0, MR 1190469.
[40]. X. Wu et al., “Top 10 algorithms in data mining”, Knowl Inf Syst, Vol. 14, pp. 1–37, 2008.
[41]. S. M. Piryonesi, T. E. El-Diraby, “Data Analytics in Asset Management: Cost-Effective Prediction of the Pavement Condition Index”, Journal of Infrastructure Systems, Vol. 26, No. 1, pp. 1-3, 2019.
[42]. H. Rajaguru, S. Chakravarthy S R, “Analysis of Decision Tree and K-Nearest Neighbor Algorithm in the Classification of Breast Cancer”, Asian Pac J Cancer Prev., Vol. 20, No. 12, pp. 3777-3781, 2019.
[43]. Y. Chen, Y. Lin, C. Kung, M. Chung, I. Yen, “Design and Implementation of Cloud Analytics-Assisted Smart Power Meters Considering Advanced Artificial Intelligence as Edge Analytics in Demand-Side Management for Smart Homes”, Sensors, Vol. 19, No. 2047, 2019.
[44]. G. Chechik, V. Sharma, U. Shalit, S. Bengio, “Large Scale Online Learning of Image Similarity Through Ranking”, Journal of Machine Learning Research, Vol. 11, pp.1109–1135, 2010.
[45]. T. Hastie, R. Tibshirani, J. Friedman, “The Elements of Statistical Learning: Data mining, Inference, and Prediction”, Springer, New York, pp. 485–586, 2009, ISBN 978-0-387-84857-0.
[46]. Y. Ye, X. Liu, Q. Liu, J. Yin, “Consensus Kernel K-Means Clustering for Incomplete Multiview Data”, Computational intelligence and neuroscience, Vol. 2017, No. 3961718, pp. 1-11, 2017.
[47]. U. U. Tamhane et al, “Association between admission neutrophil to lymphocyte ratio and outcomes in patients with acute coronary syndrome”, The American journal of cardiology, Vol. 102, No. 6, pp. 653-7, 2008.
[48]. J. He, J. Li, Y. Wang, P. Hao, Q. Hua, “Neutrophil-to-lymphocyte ratio (NLR) predicts mortality and adverse-outcomes after ST-segment elevation myocardial infarction in Chinese people”, International journal of clinical and experimental pathology, Vol. 7, No. 7, pp. 4045-56, 2014.
[49]. C. Maaser et al., “ECCO-ESGAR guideline for diagnostic assessment in IBD Part 1: initial diagnosis, monitoring of known IBD, detection of complications”, J Crohn’s Colitis, Vol. 13, No. 2, pp. 144–64, 2019.
[50]. A. Sturm et al., “ECCO-ESGAR guideline for diagnostic assessment in IBD Part 2: IBD scores and general principles and technical aspects”, J Crohn’s Colitis, Vol. 13, No. 3, pp. 273–84, 2019.
[51]. H. W. Hernandez et al., “High Throughput and Computational Repurposing for Neglected Diseases”, Pharm Res., Vol. 36, No. 27, pp. 1-20, 2018.
[52]. I. G. Medeiros, J. C. Xavier and A. M. P. Canuto, “Applying the Coral Reefs Optimization algorithm to clustering problems”, 2015 International Joint Conference on Neural Networks (IJCNN), Killarney, Ireland, pp. 1-8, 2015.
[53]. A. Mouzaki et al., “Immune Parameters That Distinguish Multiple Sclerosis Patients from Patients with Other Neurological Disorders at Presentation”, PLOS One, Vol. 10, No, 8, pp. 1-3, 2015.
[54]. A. K. Dwivedi, S. A. Imtiaz, E. rodriguez-villegas, “Algorithms for Automatic Analysis and Classification of Heart Sounds—A Systematic Review”, Vol. 7, No. 724334, pp. 8316-8340, 2019.
[55]. T. Alsuliman, D. Humaidan, L. Sliman, "Machine learning and artificial intelligence in the service of medicine: Necessity or potentiality?", Current Research in Translational Medicine, Vol. 68, No. 4, pp. 245-251, 2020.
[56]. S. Kim, J. Gil, “Research paper classification systems based on TF?IDF and LDA schemes”, Human-Centric Computing and Information Sciences, Vol. 9, No. 30, pp. 1-19, 2019.