Crime Analysis and Prediction Model Using Data Mining and Machine Learning Techniques: Comparative Analysis
Neetu Singh1 , Tripti Ajariya2 , Shailesh Raghuvanshi3 , Neha Singh4
Section:Research Paper, Product Type: Journal Paper
Volume-9 ,
Issue-6 , Page no. 97-104, Jun-2021
CrossRef-DOI: https://doi.org/10.26438/ijcse/v9i6.97104
Online published on Jun 30, 2021
Copyright © Neetu Singh, Tripti Ajariya, Shailesh Raghuvanshi, Neha Singh . This is an open access article distributed under the Creative Commons Attribution License, which permits unrestricted use, distribution, and reproduction in any medium, provided the original work is properly cited.
View this paper at Google Scholar | DPI Digital Library
How to Cite this Paper
- IEEE Citation
- MLA Citation
- APA Citation
- BibTex Citation
- RIS Citation
IEEE Style Citation: Neetu Singh, Tripti Ajariya, Shailesh Raghuvanshi, Neha Singh, “Crime Analysis and Prediction Model Using Data Mining and Machine Learning Techniques: Comparative Analysis,” International Journal of Computer Sciences and Engineering, Vol.9, Issue.6, pp.97-104, 2021.
MLA Style Citation: Neetu Singh, Tripti Ajariya, Shailesh Raghuvanshi, Neha Singh "Crime Analysis and Prediction Model Using Data Mining and Machine Learning Techniques: Comparative Analysis." International Journal of Computer Sciences and Engineering 9.6 (2021): 97-104.
APA Style Citation: Neetu Singh, Tripti Ajariya, Shailesh Raghuvanshi, Neha Singh, (2021). Crime Analysis and Prediction Model Using Data Mining and Machine Learning Techniques: Comparative Analysis. International Journal of Computer Sciences and Engineering, 9(6), 97-104.
BibTex Style Citation:
@article{Singh_2021,
author = {Neetu Singh, Tripti Ajariya, Shailesh Raghuvanshi, Neha Singh},
title = {Crime Analysis and Prediction Model Using Data Mining and Machine Learning Techniques: Comparative Analysis},
journal = {International Journal of Computer Sciences and Engineering},
issue_date = {6 2021},
volume = {9},
Issue = {6},
month = {6},
year = {2021},
issn = {2347-2693},
pages = {97-104},
url = {https://www.ijcseonline.org/full_paper_view.php?paper_id=5355},
doi = {https://doi.org/10.26438/ijcse/v9i6.97104}
publisher = {IJCSE, Indore, INDIA},
}
RIS Style Citation:
TY - JOUR
DO = {https://doi.org/10.26438/ijcse/v9i6.97104}
UR - https://www.ijcseonline.org/full_paper_view.php?paper_id=5355
TI - Crime Analysis and Prediction Model Using Data Mining and Machine Learning Techniques: Comparative Analysis
T2 - International Journal of Computer Sciences and Engineering
AU - Neetu Singh, Tripti Ajariya, Shailesh Raghuvanshi, Neha Singh
PY - 2021
DA - 2021/06/30
PB - IJCSE, Indore, INDIA
SP - 97-104
IS - 6
VL - 9
SN - 2347-2693
ER -
![]() |
![]() |
![]() |
389 | 324 downloads | 146 downloads |
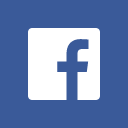
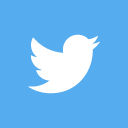
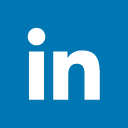
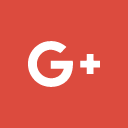
Abstract
Crime is the illegal task perform by human system for perform unauthorise3d access of various services without legal permission. in computer system there are many categories of crime related with cybercrime. the crime is basically relating to offence which is happen by illegal task and permission. N this computer system the many crime is describing various cybercrime. Like malware, hacking, cyber-attacks, cyber illegal access, IOT hacking, phishing scams, ransom ware, etc. These are described various types of cybercrime in computer technology. for determine the any one we have used various algorithm and simulation tool performance. Thyis is destroy our human society transaction system. It is also created by some human; many companies also do this illegal work for our business growth. This cyber various crime is also impact of our country growth and development also decreased. In this research work we have determinate cybercrime attacks detection and prediction model of determine the cyber-attacks using data mining and machine learning techniques. For implementation of this proposed work we are using WEKA simulation tool for execute data mining algorithms and Jupiter anaconda navigator simulate tool for machine learning algorithms for determinate accuracy of data mining and design production model using machine learning algorithms. The attacks categories by cybercrime are ddos attacks, U2r, R2L, probe, ICMP attacks, UDP attacks, TCP attacks, FAR high false alarm rate, Dos attacks, these are cyber-attacks detection by data mining and machine learning algorithms based determinate accuracy and efficiency of data mining algorithms and prediction model by machine learning algorithms
Key-Words / Index Term
cybercrime, cyber-attacks, data mining, KDD cup data set, machine learning, weak tool, Jupiter anaconda navigator simulate tool
References
[1]. Halder, D., &Jaishankar, K. (2011) Cybercrime and the Victimization of Women: Laws, Rights, and Regulations. Hershey, PA, USA: IGI Global. ISBN 978-1-60960-830-9, 2011.
[2]. Lambert, Glenn M. II, "Security Analytics: Using Deep Learning to Detect Cyber Attacks" (2017). UNF Graduate Theses and Dissertations. 728, https://digitalcommons.unf.edu/etd/728
[3]. ManjeetRege& Raymond Blanch K. Mbah, Machine Learning for Cyber Defense and Attack , DATA ANALYTICS 2018 : The Seventh International Conference on Data Analytics, Copyright (c) IARIA, 2018. ISBN: 978-1-61208-681-1 , pp.73–78., 2018.
[4]. Dmitri Koteshov, How Can Ai Change The State Of Cybersecurity, March 7, 2018, https://www.elinext.com/industries/financial/trends/aiand-security/.
[5]. Anti-Phishing Working Group, “Phishing and Fraud solutions”. [Online], [Accesses: March 18, 2019] http://www.antiphishing.org/.
[6]. S. Abu-Nimeh, D. Nappa, X. Wang, and S. Nair, “A Comparison of Machine Learning Techniques for Phishing Detection”, APWG eCrime Researchers Summit, Pittsburg, PA. October 4-5, 2007,
[7]. N. Lu, S. Mabu, T. Wang, and K. Hirasawa, “An Efficient Class Association Rule-Pruning Method for Unified Intrusion Detection System using Genetic Algorithm”, in IEEJ Transactions on Electrical and Electronic Engineering, Vol. 8, Issue 2, pp. 164 – 172, January 2, 2013.
[8]. Knowledge Discovery and Data Mining group, “KDD cup 1999” [Online], [Accessed:March 18, 2019], http://www.kdd.org/kddcup/index.php.
[9]. K. Lee, J. Caverlee, and S. Webb, “Uncovering social spammers: social honeypots + machine learning”, SIGIR’10, Geneva, Switzerland, July 19-23, 2010.
[10]. Nilaykumar Kiran Sangani & HarootZarger, Machine Learning in Application Security, [Accessed: March 18, 2019] http://dx.doi.org/10.5772/intechopen.68796.
[11]. Security Week Network. Symantec Adds Machine Learning to Endpoint Security Lineup [Internet]. 2016. Available from: http://www.securityweek.com/symantec-addsmachine-learning-endpoint-security-lineup .
[12]. Ozlem Yavanoglu & Murat Aydos, A Review on Cyber Security Datasets for Machine Learning Algorithms, 11-14 Dec. 2017, 2017 IEEE International Conference on Big Data (Big Data), INSPEC Accession Number: 17504859.
[13]. Md. Zeeshan Siddiqui &Sonali Yadav, Application Of Artificial Intelligence In Fighting Against Cyber Crimes: A Review, International Journal of Advanced Research in Computer Science April 2018 , (ISSN: 0976-5697), ISBN: 978-93-5311-643-9, page[118-121].
[14]. Nilaykumar Kiran Sangani&HarootZarger, Machine Learning in Application Security, [Accessed: March 18, 2019] http://dx.doi.org/10.5772/intechopen.68796 [15] Dmitri Koteshov, How Can Ai Change The State Of Cybersecurity, March 7, 2018, https://www.elinext.com/industries/financial/trends/aiand-security/.
[15]. Richard Power, "1999 CSI/FBI Computer Crime and Security Survey,” Computer Security Issues & Trends, Computer Security Institute, winter 1999.
[16]. Denning D E, “An Intrusion-Detection Model,” In IEEE Transaction on Software Engineering, Vol. Se-13, No. 2, pp. 222-232, February 1987.
[17]. Lee, W, Stolfo S and Mok K , “Adaptive Intrusion Detection: A Data Mining Approach,” In Artificial Intelligence Review, Kluwer Academic Publishers, 14(6), pp. 533 - 567, December 2000.
[18]. Satinder Singh, Guljeet Kaur, “Unsupervised Anomaly Detection In Network Intrusion Detection Using Clusters,” Proceedings of National Conference on Challenges & Opportunities in Information Technology RIMT-IET, Mandi Gobindgarh. March 23, 2007.
[19]. Eric Bloedorn , Alan D. Christiansen , William Hill , Clement Skorupka , Lisa M. Talbot , Jonathan Tivel, “Data Mining for Network Intrusion Detection: How to Get Started,” CiteSeer, 2001
[20]. L. Portnoy, “Intrusion Detection with Unlabeled Data Using Clustering,” Undergraduate Thesis, Columbia University, 2000.
[21]. Theodoros Lappas and Konstantinos Pelechrinis, “Data Mining Techniques for (Network) Intrusion Detection Systems,” http://citeseerx.ist.psu.edu/viewdoc/download? doi=10.1.1.120.2533&rep=rep1&type=pdf.
[22]. Dewan Md. Farid, Nouria Harbi, Suman Ahmmed, Md. Zahidur Rahman, and Chowdhury Mofizur Rahman, “Mining Network Data for Intrusion Detection through Naïve Bayesian with Clustering”, World Academy of Science, Engineering and Technology, 2010.
[23]. The KDD Archive. KDD99 cup dataset, 1999. http://kdd.ics.uci.edu/databases/kddcup99/kddcup99.html
[24]. X. Li and N. Ye., “A supervised clustering algorithm for computer intrusion detection,” Knowledge and Information Systems, 8, pp498-509, ISSN 0219-1377, 2005
[25]. Kruegel C., Mutz D., Robertson W., Valeur F., “Bayesian event classification for intrusion detection,” In: Proceedings of the 19th Annual Computer Security Applications Conference; 2003.
[26]. Portnoy L., Eskin E., Stolfo S.J., “Intrusion detection with unlabeled data using clustering,” In: Proceedings of The ACM Workshop on Data Mining Applied to Security; 2001.
[27]. Paxson V., “Bro: A System for Detecting Network Intruders in Real-Time”, Computer Networks, 31(23-24), pp. 2435-2463, 14 Dec. 1999.
[28]. D.Barbara, J.Couto, S.Jajodia, and N.Wu, "ADAM: A test bed for exploring the use of data mining in intrusion detection”, SIGMOD, vol30, no.4, pp 15-24, 2001.
[29]. P.Domingos, and M.J. Pizzani, "On the optimality of the simple Bayesian classifier under zero-one loss”, m/c learning, Vol.29, no2-3, pp 103-130, 1997.
[30]. F. Provost, and T. Fawcett, “Robust classification for imprecise environment,” Machine Learning, vol. 42/3, pp. 203-231. 2001.
[31]. Athanasios Papoulis and S. Unnikrishna Pillai,. "Probability, Random Variables and stochastic Processes ", McGraw-Hill, Fourth Edition, ISBN 0073660116, 2002.
[32]. P. Kabiri and A.A. Ghorbani, “Research on Intrusion Detection and Response: A Survey,” International Journal of Network Security, 1, 84-102, September 2005.
[33]. A. Patcha and J-M. Park, “An overview of anomaly detection techniques: Existing solutions and latest technological trends,” Comput. Netw., 51, 3448-3470. ISSN 1389-1286. 2007.
[34]. T.M. Mitchell. Machine Learning. McGraw-Hill. ISBN: 0-07-115467-1, 1997.
[35]. N. Ben Amor, S. Benferhat and Z. Elouedi, “Naive Bayes vs Decision Trees in Intrusion Detection Systems,” Proceedings of the ACM symposium on Applied computing, ISBN 1-58113-812-1, pages 420-424, New York, USA, 2004.
[36]. M. Panda and M.R. Patra, “Network intrusion detection using naive bayes,” IJCSNS International Journal of Computer Science and Network Security, 7, 258-263, 2007
[37]. F. Gharibian and A.A. Ghorbani, “Comparative Study of Supervised Machine Learning Techniques for Intrusion Detection,” In CNSR ’07: Proceedings of the Fifth Annual Conference on Communication Networks and Services Research, Pages 350-358, Washington, DC, USA, 2007
[38]. L. Portnoy, E. Eskin and S. Stolfo, “Intrusion Detection With Unlabeled Data Using Clustering,” In Proceedings of the ACM Workshop on Data Mining Applied to Security, 2001.
[39]. K. Leung and C. Leckie, “Unsupervised anomaly detection in network intrusion detection using clusters,” Proceedings of the 28th Australasian conference on Computer Science, ISBN 1-920-68220-1, pages 333-342, Darlinghurst, Australia, Australia, 2005.
[40]. W. Wang, X. Guan and X. Zhang, “Processing of massive audit data streams for real-time anomaly intrusion detection,” Comput. Commun., 31, 58- 72. ISSN 0140-3664, 2008
[41]. J. Song, K. Ohira, H. Takakura, Y. Okabe and Y. Kwon, “A Clustering Method for Improving Performance of Anomaly-Based Intrusion Detection System,” IEICE Transactions on Information and Systems, E91-D, 1282-1291. ISSN 0916-8532, 2008
[42]. E.J. Spinosa, A.P. de Leon F. de Carvalho and J. Gama, “Cluster-based novel concept detection in data streams applied to intrusion detection in computer networks,” Proceedings of the ACM symposium on Applied computing, pages 97.