TLA: Twitter Linguistic Analysis
Tushar Sarkar1 , Nishant Rajadhyaksha2
Section:Research Paper, Product Type: Journal Paper
Volume-9 ,
Issue-8 , Page no. 34-37, Aug-2021
CrossRef-DOI: https://doi.org/10.26438/ijcse/v9i8.3437
Online published on Aug 31, 2021
Copyright © Tushar Sarkar, Nishant Rajadhyaksha . This is an open access article distributed under the Creative Commons Attribution License, which permits unrestricted use, distribution, and reproduction in any medium, provided the original work is properly cited.
View this paper at Google Scholar | DPI Digital Library
How to Cite this Paper
- IEEE Citation
- MLA Citation
- APA Citation
- BibTex Citation
- RIS Citation
IEEE Citation
IEEE Style Citation: Tushar Sarkar, Nishant Rajadhyaksha, “TLA: Twitter Linguistic Analysis,” International Journal of Computer Sciences and Engineering, Vol.9, Issue.8, pp.34-37, 2021.
MLA Citation
MLA Style Citation: Tushar Sarkar, Nishant Rajadhyaksha "TLA: Twitter Linguistic Analysis." International Journal of Computer Sciences and Engineering 9.8 (2021): 34-37.
APA Citation
APA Style Citation: Tushar Sarkar, Nishant Rajadhyaksha, (2021). TLA: Twitter Linguistic Analysis. International Journal of Computer Sciences and Engineering, 9(8), 34-37.
BibTex Citation
BibTex Style Citation:
@article{Sarkar_2021,
author = {Tushar Sarkar, Nishant Rajadhyaksha},
title = {TLA: Twitter Linguistic Analysis},
journal = {International Journal of Computer Sciences and Engineering},
issue_date = {8 2021},
volume = {9},
Issue = {8},
month = {8},
year = {2021},
issn = {2347-2693},
pages = {34-37},
url = {https://www.ijcseonline.org/full_paper_view.php?paper_id=5375},
doi = {https://doi.org/10.26438/ijcse/v9i8.3437}
publisher = {IJCSE, Indore, INDIA},
}
RIS Citation
RIS Style Citation:
TY - JOUR
DO = {https://doi.org/10.26438/ijcse/v9i8.3437}
UR - https://www.ijcseonline.org/full_paper_view.php?paper_id=5375
TI - TLA: Twitter Linguistic Analysis
T2 - International Journal of Computer Sciences and Engineering
AU - Tushar Sarkar, Nishant Rajadhyaksha
PY - 2021
DA - 2021/08/31
PB - IJCSE, Indore, INDIA
SP - 34-37
IS - 8
VL - 9
SN - 2347-2693
ER -
![]() |
![]() |
![]() |
716 | 539 downloads | 218 downloads |
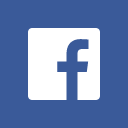
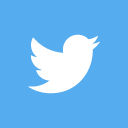
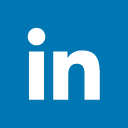
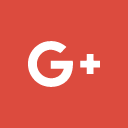
Abstract
Linguistics have been instrumental in developing a deeper understanding of human nature. Words are indispensable to bequeath the thoughts, emotions, and purpose of any human interaction, and critically analyzing these words can elucidate the social and psychological behavior and characteristics of these social animals. Social media has become a platform for human interaction on a large scale and thus gives us scope for collecting and using that data for our study. However, this entire process of collecting, labeling, and analyzing this data iteratively makes the entire procedure cumbersome. To make this entire process easier and structured, we would like to introduce TLA (Twitter Linguistic Analysis). In this paper, we describe TLA and provide a basic understanding of the framework and discuss the process of collecting, labeling, and analyzing data from Twitter for a corpus of languages while providing detailed labeled datasets for all the languages and the models are trained on these datasets. The analysis provided by TLA will also go a long way in understanding the sentiments of different linguistic communities and come up with new and innovative solutions for their problems based on the analysis.
Key-Words / Index Term
TLA, Machine Learning, Analysis, NLP
References
[1] W. Downes, S. F. W. Downes, “Language and society”, Vol. 10, Cambridge university press, Vol.10, 1998.
[2] S. R. Anderson, “How many languages are there in the world”, Linguistic Society of America, 2010
[3] C. C. Miller, “Who`s driving twitter`s popularity? not teens”, New York Times, Vol. 25, pp.2009, 2009.
[4] W. Weerkamp, S. Carter, M. Tsagkias, “How people use twitter in different languages.”,Citeseer, 2011
[5] D. Tatar,“Word sense disambiguation by machine learning approach: A short survey”, Fundamenta Informaticae, Vol. 64, No.1-4, pp.433-442, 2005
[6] H. Saif, Y. He, H. Alani,”Semantic sentiment analysis of twitter”, In the Proceedings of the 2012 Inter-national semantic web conference, Springer, pp. 508-524, 2012
[7] B. Wang, N. Z. Gong, H. Fu, Gang: “Detecting fraudulent users in online social networks via guilt-by-association on directed graphs”, in the proceedingd of the 2017 IEEE International Conference on Data Mining (ICDM), IEEE, pp. 465-474 , 2017
[8] A. M. Founta, C. Djouvas, D. Chatzakou, I. Leontiadis, J. Blackburn, G. Stringhini, A. Vakali, M. Sirivianos, N. Kourtellis, ”Large scale crowdsourcing and characterization of twitter abusive behavior”, in the Proceedings of the Twelfth International AAAI Conference on Web and Social Media, 2018.
[9] Richard Socher, Alex Perelygin, Jean Wu, JasonChuang, Christopher D. Manning, Andrew Y. Ng, and Christopher Potts. 2013.”Recursive deep models or semantic compositionality over a sentiment treebank”.In the Proceedings of the Conference on Empirical Methods in Natural Language Processing, pp. 1631–1642. 2013
[10] V. Svetnik, A. Liaw, C. Tong, J. C. Culberson, R. P. Sheridan, B. P. Feuston, “Random forest: a classication and regression tool for compound classication and qsar modeling”, Journal of chemical information and computer sciences, Vol.43, No.6, pp. 1947-1958, 2003
[11] Balahur, Alexandra. "Sentiment analysis in social media texts.",In the Proceedings of the 4th workshop on computational approaches to subjectivity, sentiment and social media analysis, pp. 120-128, 2013.
[12] Segura-Bedmar, Isabel, Ricardo Revert, and Paloma Martínez. "Detecting drugs and adverse events from Spanish social media streams." In the Proceedings of the 5th international workshop on health text mining and information analysis (LOUHI), pp. 106-115, 2014.
[13] S. Amrita, Jobin Joseph, Rona Shaji, Athul Prasad, Rahul Gopal, "E-Stress Detector", International Journal of Computer Sciences and Engineering, Vol.8, Issue.6, pp.25-29, 2020.
[14] Taboada, Maite. "Sentiment analysis: An overview from linguistics." Annual Review of Linguistics, Vol. 2, No.1,, pp. 325-347, 2016
[15] P. J. Tighe, R. C. Goldsmith, M. Gravenstein, H. R. Bernard, R. B. Fillingim, “The painful tweet: text, sentiment, and community structure analyses of tweets pertaining to pain”, Journal of medical Internet research, vol. 17, No.4, pp. e84, 2015
[16] J. Blair, C.-Y. Hsu, L. Qiu, S.-H. Huang, T.-H. K. Huang, S. Abdullah,”Using tweets to assess mental well-being of essential workers during thecovid-19 pandemic”,In the Proceedings of the 2021 CHI Conference on Human Factors in Computing Systems, pp. 1-6, 2021
[17] E. Loper, S. Bird, “Nltk: The natural language toolkit”, arXiv preprintcs/0205028.
[18] J. Devlin, M.-W. Chang, K. Lee, K. Toutanova, ”Bert: Pre-training of deep bidirectional transformers for language understanding”, arXiv preprint arXiv:1810.04805.
[19] Saurav Singla, Vikash Kumar, "Multi-Class Sentiment Classification using Machine Learning and Deep Learning Techniques", International Journal of Computer Sciences and Engineering, Vol.8, Issue.11, pp.14-20, 2020.