Emotion Analysis and Performance Perdiction Using Cluster Based LDA
Kajal Devi1 , Harjinder Kaur2
Section:Research Paper, Product Type: Journal Paper
Volume-9 ,
Issue-9 , Page no. 16-24, Sep-2021
CrossRef-DOI: https://doi.org/10.26438/ijcse/v9i9.1624
Online published on Sep 30, 2021
Copyright © Kajal Devi, Harjinder Kaur . This is an open access article distributed under the Creative Commons Attribution License, which permits unrestricted use, distribution, and reproduction in any medium, provided the original work is properly cited.
View this paper at Google Scholar | DPI Digital Library
How to Cite this Paper
- IEEE Citation
- MLA Citation
- APA Citation
- BibTex Citation
- RIS Citation
IEEE Style Citation: Kajal Devi, Harjinder Kaur, “Emotion Analysis and Performance Perdiction Using Cluster Based LDA,” International Journal of Computer Sciences and Engineering, Vol.9, Issue.9, pp.16-24, 2021.
MLA Style Citation: Kajal Devi, Harjinder Kaur "Emotion Analysis and Performance Perdiction Using Cluster Based LDA." International Journal of Computer Sciences and Engineering 9.9 (2021): 16-24.
APA Style Citation: Kajal Devi, Harjinder Kaur, (2021). Emotion Analysis and Performance Perdiction Using Cluster Based LDA. International Journal of Computer Sciences and Engineering, 9(9), 16-24.
BibTex Style Citation:
@article{Devi_2021,
author = {Kajal Devi, Harjinder Kaur},
title = {Emotion Analysis and Performance Perdiction Using Cluster Based LDA},
journal = {International Journal of Computer Sciences and Engineering},
issue_date = {9 2021},
volume = {9},
Issue = {9},
month = {9},
year = {2021},
issn = {2347-2693},
pages = {16-24},
url = {https://www.ijcseonline.org/full_paper_view.php?paper_id=5389},
doi = {https://doi.org/10.26438/ijcse/v9i9.1624}
publisher = {IJCSE, Indore, INDIA},
}
RIS Style Citation:
TY - JOUR
DO = {https://doi.org/10.26438/ijcse/v9i9.1624}
UR - https://www.ijcseonline.org/full_paper_view.php?paper_id=5389
TI - Emotion Analysis and Performance Perdiction Using Cluster Based LDA
T2 - International Journal of Computer Sciences and Engineering
AU - Kajal Devi, Harjinder Kaur
PY - 2021
DA - 2021/09/30
PB - IJCSE, Indore, INDIA
SP - 16-24
IS - 9
VL - 9
SN - 2347-2693
ER -
![]() |
![]() |
![]() |
268 | 389 downloads | 124 downloads |
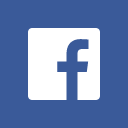
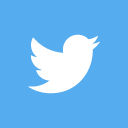
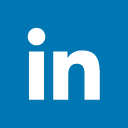
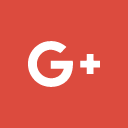
Abstract
Kajal Devi, Harjinder Kaur, for a productive life, Marketing plays a critical role to fill individual life with value and excellence. Marketing is compulsory to provide things that individuals partake in to compete in the modern world. Predicting the academic performance of the Business is the most successive research in this era. A different set of approaches and methods are incorporated to increase Business performance. However, this is a challenging task due to the wrong course selection. In this paper, we have used the Cluster-based Linear Discriminant Analysis (CLDA) and Artificial Neural Network (ANN) based approaches for the prediction and classification of Business performance. The proposed study will provide the prospective business with the motivational comments and the video recommendations by which Business can choose the right subject and the comments will facilitate the Business with the insight reasons of dropout opted by other Business for this course. The outcomes of this study will help in the reduction of the number of dropouts. The Business will be able to choose an appropriate course for performance enhancement and carrier excel.
Key-Words / Index Term
Cluster-based Linear Discriminant Analysis (CLDA), Business performance, Dropouts, Classification, Prediction, and machine learning
References
[1]. Aleman, L., & Garza, D. E. L. A.. RESEARCH ANALYSIS ON MOOC COURSE DROPOUT AND. IEEE Access, April, 3–14, 2016.
[2]. Battin-Pearson, S., Newcomb, M. D., Abbott, R. D., Hill, K. G., Catalano, R. F., & Hawkins, J. D. (2000). Predictors of early high school dropout: A test of five theories. Journal of Marketingal Psychology, 92(3), 568–582, 2000. https://doi.org/10.1037/0022-0663.92.3.568
[3]. Chen, J., Feng, J., Sun, X., Wu, N., Yang, Z., & Chen, S. (2019). MOOC Dropout Prediction Using a Hybrid Algorithm Based on Decision Tree and Extreme Learning Machine. IEEE Access, 2019.
[4]. De Santos, K. J. O., Menezes, A. G., De Carvalho, A. B., & Montesco, C. A. E. (2019). Supervised learning in the context of Marketingal data mining to avoid university Businesss dropout. Proceedings - IEEE 19th International Conference on Advanced Learning Technologies, ICALT 2019, 207–208. https://doi.org/10.1109/ICALT.2019.00068
[5]. Feng, W., Tang, J., & Liu, T. X. (2015). Understanding Dropouts in MOOCs. IEEE Access.
[6]. Fortin, L., Lessard, A., & Marcotte, D. (2010). Comparison by gender of Businesss with behavior problems who dropped out of school. Procedia - Social and Behavioral Sciences, 2(2), 5530–5538. https://doi.org/10.1016/j.sbspro.2010.03.902
[7]. Fox, C. K., Barr-Anderson, D., Neumark-Sztainer, D., & Wall, M. (2010). Physical activity and sports team participation: Associations with academic outcomes in middle school and high school Businesss. Journal of School Health, 80(1), 31–37. https://doi.org/10.1111/j.1746-1561.2009.00454.x
[8]. Haraty, R. A., Dimishkieh, M., & Masud, M. (2014). An Enhanced k -means Clustering Algorithm for Pattern Discovery in Healthcare Data. International Journal of Distributed Sensor Networks, 1–18, 2015.
[9]. Hasbun, T., Araya, A., & Villalon, J. (2016). Extracurricular activities as dropout prediction factors in higher Marketing using decision trees. Proceedings - IEEE 16th International Conference on Advanced Learning Technologies, ICALT 2016, 242–244. https://doi.org/10.1109/ICALT.2016.66
[10]. Kingdom, U. (2015). DROPOUT RATES OF MASSIVE OPEN ONLINE COURSES?: BEHAVIOURAL PATTERNS MOOC Dropout and Completion?: Existing Evaluations. IEEE Access.
[11]. Kizilcec, R. F., Piech, C., & Schneider, E. (2013). Deconstructing disengagement: Analyzing learner subpopulations in massive open online courses. ACM International Conference Proceeding Series, 170–179. https://doi.org/10.1145/2460296.2460330
[12]. Kloft, M., Stiehler, F., Zheng, Z., & Pinkwart, N. (2014). Predicting MOOC Dropout over Weeks Using Machine Learning Methods. IEEE Access, 60–65.
[13]. Kuo, J. Y., Pan, C. W., & Lei, B. (2017). Using Stacked Denoising Autoencoder for the Business Dropout Prediction. Proceedings - 2017 IEEE International Symposium on Multimedia, ISM 2017, 2017-January, 483–488. https://doi.org/10.1109/ISM.2017.96
[14]. Liang, J., Yang, J., Wu, Y., Li, C., & Zheng, L. (2016). Big data application in Marketing: Dropout prediction in Marketing MOOCs. Proceedings - 2016 IEEE 2nd International Conference on Multimedia Big Data, BigMM 2016, 440–443. https://doi.org/10.1109/BigMM.2016.70
[15]. Limsathitwong, K., Tiwatthanont, K., & Yatsungnoen, T. (2018). Dropout prediction system to reduce discontinue study rate of information technology Businesss. Proceedings of 2018 5th International Conference on Business and Industrial Research: Smart Technology for Next Generation of Information, Engineering, Business, and Social Science, ICBIR 2018, 110–114. https://doi.org/10.1109/ICBIR.2018.8391176
[16]. Liu, F., Wang, L., Qian, Y., & Wu, Y.. Analysis of MOOCs Courses Dropout Rate Based on Businesss ’ Studying Behaviors. IEEE Access, 83(Hss), 139–144, 2017.
[17]. Manhães, L. M. B., Da Cruz, S. M. S., & Zimbrão, G. (2014). WAVE: An architecture for predicting dropout in undergraduate courses using EDM. Proceedings of the ACM Symposium on Applied Computing, 243–245. https://doi.org/10.1145/2554850.2555135
[18]. Márquez-Vera, C., Cano, A., Romero, C., & Ventura, S. (2013). Predicting Business failure at school using genetic programming and different data mining approaches with high dimensional and imbalanced data. Applied Intelligence, 38(3), 315–330. https://doi.org/10.1007/s10489-012-0374-8
[19]. Mohan, A., Sun, H., Lederman, O., Full, K., & Pentland, A. (2018). Measurement and Feedback of Group Activity Using Wearables for Face-to-Face Collaborative Learning. 2018 IEEE 18th International Conference on Advanced Learning Technologies (ICALT), 163–167. https://doi.org/10.1109/ICALT.2018.00046
[20]. Onah, D., & Sinclair, J. (2014). Dropout Rates of Massive Open Online Courses?: Behavioural Patterns DROPOUT RATES OF MASSIVE OPEN ONLINE COURSES?: BEHAVIOURAL PATTERNS. IEEE Access, July. https://doi.org/10.13140/RG.2.1.2402.0009
[21]. Osmanbegovic, E., Suljic, M. (2012). Data mining approach for predicting Business performance. Journal of Economics and Business, X(1), 3–12.
[22]. Pal, S. (2012). Mining Marketingal Data to Reduce Dropout Rates of Engineering Businesss. International Journal of Information Engineering and Electronic Business, 4(2), 1–7. https://doi.org/10.5815/ijieeb.2012.02.01
[23]. Romero, C., & Ventura, S. (2007). Marketingal data mining: A survey from 1995 to 2005. Expert Systems with Applications, 33(1), 135–146. https://doi.org/10.1016/j.eswa.2006.04.005
[24]. Tinto, V. (1975). Dropout from Higher Marketing: A Theoretical Synthesis of Recent Research. Review of Marketingal Research, 45(1), 89–125. https://doi.org/10.3102/00346543045001089
[25]. Tsiakmaki, M., Kostopoulos, G., Kotsiantis, S., & Ragos, O. (2019). Implementing AutoML in Marketingal Data Mining for Prediction Tasks. Applied Sciences, 10(1), 90. https://doi.org/10.3390/app10010090
[26]. Wang, W., & Graduate, I. (2015). Deep Model for Dropout Prediction in MOOCs. IEEE Access.
[27]. Wibisono, A., Jatmiko, W., Wisesa, H. A., Hardjono, B., & Mursanto, P. (2016). Knowledge-Based Systems Traffic big data prediction and visualization using Fast Incremental Model Trees-Drift Detection ( FIMT-DD ). Knowledge-Based Systems, 93, 33–46. https://doi.org/10.1016/j.knosys.2015.10.028
[28]. Zepke, N., Leach, L., & Prebble, T. (2006). Being learner-centered: One way to improve Business retention? Studies in Higher Marketing, 31(5), 587–600. https://doi.org/10.1080/03075070600923418
[29]. Zheng, X., Member, S., Chen, C., Hung, J., He, W., Hong, F., & Lin, Z. (2015). A Hybrid Trust-based Recommender System for Online Communities of Practice. IEEE Transactions on Learning Technologies, 1382(c), 1–13. https://doi.org/10.1109/TLT.2015.2419262
[30]. Hector Varga, Ruben Heradio, Jesus Chacon, Luis LA Torre, Gonzalo Faria (2019)"Automated Assessment and Monitoring Support for Competency-Based Courses" IEEE.
[31]. Cios, K.J., Pedrycz W., Swiniarski, R.W. & Kurgan, L.A. (2007), Data Mining: A Knowledge Discovery Approach, Springer, New York.
[32]. Klosgen, W. & Zytkow, J. (2002), Handbook of data mining and knowledge discovery oxford university press, New York.
[33]. Edin Osmanbegovi? , Mirza Sulji? DATA MINING APPROACH FOR PREDICTING BUSINESS PERFORMANCE
[34]. Kalpesh P. Chaudhari1, Riya A. Sharma2, Shreya S. Jha3, Rajeshwari J. Bari4 Business Performance Prediction System using Data Mining Approach.
[35]. Hector Varga, Ruben Heradio, Jesus chacon, Luis LA Torre, Gonzalo Faria,"Automated Assessment and Monitoring Support for Competency-Based Courses" IEEE,2019.