A Novel Ensemble Model for Classification of Chronic Kidney Disease With Selected Features and Components
Sanat Kumar Sahu1
Section:Research Paper, Product Type: Journal Paper
Volume-9 ,
Issue-9 , Page no. 66-69, Sep-2021
CrossRef-DOI: https://doi.org/10.26438/ijcse/v9i9.6669
Online published on Sep 30, 2021
Copyright © Sanat Kumar Sahu . This is an open access article distributed under the Creative Commons Attribution License, which permits unrestricted use, distribution, and reproduction in any medium, provided the original work is properly cited.
View this paper at Google Scholar | DPI Digital Library
How to Cite this Paper
- IEEE Citation
- MLA Citation
- APA Citation
- BibTex Citation
- RIS Citation
IEEE Style Citation: Sanat Kumar Sahu, “A Novel Ensemble Model for Classification of Chronic Kidney Disease With Selected Features and Components,” International Journal of Computer Sciences and Engineering, Vol.9, Issue.9, pp.66-69, 2021.
MLA Style Citation: Sanat Kumar Sahu "A Novel Ensemble Model for Classification of Chronic Kidney Disease With Selected Features and Components." International Journal of Computer Sciences and Engineering 9.9 (2021): 66-69.
APA Style Citation: Sanat Kumar Sahu, (2021). A Novel Ensemble Model for Classification of Chronic Kidney Disease With Selected Features and Components. International Journal of Computer Sciences and Engineering, 9(9), 66-69.
BibTex Style Citation:
@article{Sahu_2021,
author = {Sanat Kumar Sahu},
title = {A Novel Ensemble Model for Classification of Chronic Kidney Disease With Selected Features and Components},
journal = {International Journal of Computer Sciences and Engineering},
issue_date = {9 2021},
volume = {9},
Issue = {9},
month = {9},
year = {2021},
issn = {2347-2693},
pages = {66-69},
url = {https://www.ijcseonline.org/full_paper_view.php?paper_id=5398},
doi = {https://doi.org/10.26438/ijcse/v9i9.6669}
publisher = {IJCSE, Indore, INDIA},
}
RIS Style Citation:
TY - JOUR
DO = {https://doi.org/10.26438/ijcse/v9i9.6669}
UR - https://www.ijcseonline.org/full_paper_view.php?paper_id=5398
TI - A Novel Ensemble Model for Classification of Chronic Kidney Disease With Selected Features and Components
T2 - International Journal of Computer Sciences and Engineering
AU - Sanat Kumar Sahu
PY - 2021
DA - 2021/09/30
PB - IJCSE, Indore, INDIA
SP - 66-69
IS - 9
VL - 9
SN - 2347-2693
ER -
![]() |
![]() |
![]() |
206 | 192 downloads | 135 downloads |
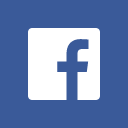
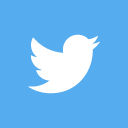
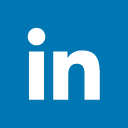
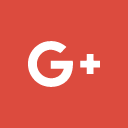
Abstract
Diagnosis of health conditions is an incredibly difficult and significant issue in the field of medical science. Classification, dimension reduction technique (DRT), feature selection techniques (FST) play a very important role in the quick and accurate identification of disease. The chronic kidneys disease (CKD) dataset is connected into three classification methods like RF, J48 and C5.0. The proposed ensemble model (RF, J48 and C5.0) gives better accuracy i.e. 99.75% contrast with all classifiers with selected feature subset. All classification models give a better outcome with proposed PC-DRT and GA-FST when contrasted with without FST. The outcomes showed that utilizing GA-FST has computationally enhanced the classification accuracy.
Key-Words / Index Term
Classification, chronic kidneys disease, dimension reduction technique, ensemble model, feature selection techniques, genetic algorithm, principal component analysis
References
[1] A. Subasi, E. Alickovic, and J. Kevric, “Diagnosis of Chronic Kidney Disease by Using Random Forest,” C. 2017 Proc. Int. Conf. Med. Biol. Eng. 2017, vol. 7, no. 1, pp. 589–594, 2017.
[2] H. Polat, H. Danaei Mehr, and A. Cetin, “Diagnosis of Chronic Kidney Disease Based on Support Vector Machine by Feature Selection Methods,” J. Med. Syst., vol. 41, no. 4, 2017.
[3] N. Boodhun and M. Jayabalan, “Risk prediction in life insurance industry using supervised learning algorithms,” Complex Intell. Syst., no. March, 2018.
[4] M. Dash and H. Liu, “Feature selection for classification,” Intell. Data Anal., vol. 1, no. 3, pp. 131–156, 1997.
[5] C. W. Tsai, S. J. Liu, and Y. C. Wang, “A parallel metaheuristic data clustering framework for cloud,” J. Parallel Distrib. Comput., vol. 116, pp. 39–49, 2018.
[6] M. C. Thomas, W. Zhu, and J. A. Romagnoli, “Data mining and clustering in chemical process databases for monitoring and knowledge discovery,” J. Process Control, vol. 67, pp. 160–175, 2018.
[7] M. K. Najafabadi, M. N. ri Mahrin, S. Chuprat, and H. M. Sarkan, “Improving the accuracy of collaborative filtering recommendations using clustering and association rules mining on implicit data,” Comput. Human Behav., vol. 67, pp. 113–128, 2017.
[8] J. Hou and W. Liu, “Parameter independent clustering based on dominant sets and cluster merging,” Inf. Sci. (Ny)., vol. 405, pp. 1–17, 2017.
[9] A. Subasi, E. Alickovic, and J. Kevric, “Diagnosis of Chronic Kidney Disease by Using Random Forest,” vol. 7, no. 1, pp. 589–594, 2017.
[10] V. Kunwar, K. Chandel, A. S. Sabitha, and A. Bansal, “Chronic Kidney Disease Analysis Using Data Mining Classification,” Cloud Syst. Big Data Eng. (Confluence), 2016 6th Int. Conf. IEEE, pp. 300–305, 2016.
[11] P. Sinha, “Comparative Study of Chronic Kidney Disease Prediction using KNN and SVM,” vol. 4, no. 12, pp. 608–612, 2015.
[12] A. Pujari, Data mining techniques, Third. University press, 2013.
[13] J. Ross, Q. Morgan, and K. Publishers, “Book Review?: C4 . 5?: Programs for Machine Learning,” vol. 240, pp. 235–240, 1994.
[14] Y. Zhang and L. Wu, “Classification of fruits using computer vision and a multiclass support vector machine,” Sensors (Switzerland), vol. 12, no. 9, pp. 12489–12505, 2012.
[15] L. C. Paul, A. Al Suman, and N. Sultan, “Methodological Analysis of Principal Component Analysis (PCA) Method,” IJCEM Int. J. Comput. Eng. Manag. ISSN, vol. 16, no. 2, pp. 2230–7893, 2013.
[16] P. Yildirim, “Filter Based Feature Selection Methods for Prediction of Risks in Hepatitis Disease,” Int. J. Mach. Learn. Comput., vol. 5, no. 4, pp. 258–263, 2015.
[17] M. Einasto et al., “SDSS DR7 superclusters. Principal component analysis,” pp. 1–13, 2011.
[18] P. Verma, V. K. Awasthi, and S. K. Sahu, “A Novel Design of Classification of Coronary Artery Disease Using Deep Learning and Data Mining Algorithms,” Rev. d ’ Intell. Artif., vol. 35, no. 3, pp. 209–215, 2021.
[19] D. E. Goldberg, “Genetic Algorithms in Search Optimization & Machine Learning.” p. 412, 1989.
[20] M. Hall, “Correlation-based Feature Selection for Machine Learning,” Methodology, vol. 21i195-i20, no. April, pp. 1–5, 1999.
[21] P. J. Fleming and C. M. Fonseca, “Genetic Algorithms in Control Systems Engineering,” IFAC Proc. Vol., vol. 26, no. 2, pp. 605–612, 1993.
[22] S. K. Sahu and A. K. Shrivas, “Analysis and Comparison of Clustering Techniques for Chronic Kidney Disease With Genetic Algorithm,” Int. J. Comput. Vis. Image Process., vol. 8, no. 4, pp. 16–25, 2018.
[23] “CKD,” 2015. [Online]. Available: https://archive.ics.uci.edu/ml/datasets/Chronic_Kidney_Disease. [Accessed: 01-Jan-2016].