Machine Learning for Mars Exploration
Ali Momennasab1
Section:Review Paper, Product Type: Journal Paper
Volume-9 ,
Issue-11 , Page no. 29-38, Nov-2021
CrossRef-DOI: https://doi.org/10.26438/ijcse/v9i11.2938
Online published on Nov 30, 2021
Copyright © Ali Momennasab . This is an open access article distributed under the Creative Commons Attribution License, which permits unrestricted use, distribution, and reproduction in any medium, provided the original work is properly cited.
View this paper at Google Scholar | DPI Digital Library
How to Cite this Paper
- IEEE Citation
- MLA Citation
- APA Citation
- BibTex Citation
- RIS Citation
IEEE Style Citation: Ali Momennasab, “Machine Learning for Mars Exploration,” International Journal of Computer Sciences and Engineering, Vol.9, Issue.11, pp.29-38, 2021.
MLA Style Citation: Ali Momennasab "Machine Learning for Mars Exploration." International Journal of Computer Sciences and Engineering 9.11 (2021): 29-38.
APA Style Citation: Ali Momennasab, (2021). Machine Learning for Mars Exploration. International Journal of Computer Sciences and Engineering, 9(11), 29-38.
BibTex Style Citation:
@article{Momennasab_2021,
author = {Ali Momennasab},
title = {Machine Learning for Mars Exploration},
journal = {International Journal of Computer Sciences and Engineering},
issue_date = {11 2021},
volume = {9},
Issue = {11},
month = {11},
year = {2021},
issn = {2347-2693},
pages = {29-38},
url = {https://www.ijcseonline.org/full_paper_view.php?paper_id=5415},
doi = {https://doi.org/10.26438/ijcse/v9i11.2938}
publisher = {IJCSE, Indore, INDIA},
}
RIS Style Citation:
TY - JOUR
DO = {https://doi.org/10.26438/ijcse/v9i11.2938}
UR - https://www.ijcseonline.org/full_paper_view.php?paper_id=5415
TI - Machine Learning for Mars Exploration
T2 - International Journal of Computer Sciences and Engineering
AU - Ali Momennasab
PY - 2021
DA - 2021/11/30
PB - IJCSE, Indore, INDIA
SP - 29-38
IS - 11
VL - 9
SN - 2347-2693
ER -
![]() |
![]() |
![]() |
386 | 324 downloads | 158 downloads |
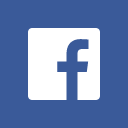
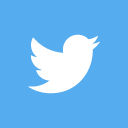
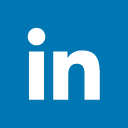
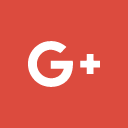
Abstract
Risk to human astronauts and interplanetary distance causing slow and limited communication drives scientists to pursue an autonomous approach to exploring distant planets, such as Mars. A portion of exploration of Mars has been conducted through the autonomous collection and analysis of Martian data by spacecraft such as the Mars rovers and the Mars Express Orbiter. The autonomy used on these Mars exploration spacecraft and on Earth to analyze data collected by these vehicles mainly consist of machine learning, a field of artificial intelligence where algorithms collect data and self-improve with the data. Additional applications of machine learning techniques for Mars exploration have potential to resolve communication limitations and human risks of interplanetary exploration. In addition, analyzing Mars data with machine learning has the potential to provide a greater understanding of Mars in numerous domains such as its climate, atmosphere, and potential future habitation. In order to explore further utilizations of machine learning techniques for Mars exploration, this paper will first summarize the general features and phenomena of Mars to provide a general overview of the planet, elaborate upon uncertainties of Mars that would be beneficial to explore and understand, summarize every current or previous usage of machine learning techniques in the exploration of Mars, explore implementations of machine learning that will be utilized in future Mars exploration missions, and explore machine learning techniques used in Earthly domains to provide solutions to the previously described uncertainties of Mars.
Key-Words / Index Term
machine learning applications, Mars, autonomy
References
[1] I. El Naqa and M. J. Murphy, “What Is Machine Learning?,” Machine Learning in Radiation Oncology. Springer International Publishing, pp. 3–11, 2015. doi: 10.1007/978-3-319-18305-3_1.
[2] A. McGovern and K. L. Wagstaff, “Machine learning in space: extending our reach,” Machine Learning, vol. 84, no. 3. Springer Science and Business Media LLC, pp. 335–340, Apr. 30, 2011. doi: 10.1007/s10994-011-5249-4.
[3] G. Genta, “Reasons for human Mars exploration,” Next Stop Mars. Springer International Publishing, pp. 38–52, Dec. 31, 2016. doi: 10.1007/978-3-319-44311-9_2.
[4] C. Leovy, “Weather and climate on Mars,” Nature, vol. 412, no. 6843. Springer Science and Business Media LLC, pp. 245–249, Jul. 2001. doi: 10.1038/35084192.
[5] N. Barlow, “Mars: An Introduction to its Interior, Surface and Atmosphere.” Cambridge University Press, 2008. doi: 10.1017/cbo9780511536069.
[6] D. M. Hassler et al., “Mars’ Surface Radiation Environment Measured with the Mars Science Laboratory’s Curiosity Rover,” Science, vol. 343, no. 6169. American Association for the Advancement of Science (AAAS), Jan. 24, 2014. doi: 10.1126/science.1244797.
[7] B. M. Jakosky and R. J. Phillips, “Mars’ volatile and climate history,” Nature, vol. 412, no. 6843. Springer Science and Business Media LLC, pp. 237–244, Jul. 2001. doi: 10.1038/35084184.
[8] T. Estlin et al., “Automated Targeting for the MER Rovers,” 2009 Third IEEE International Conference on Space Mission Challenges for Information Technology. IEEE, Jul. 2009. doi: 10.1109/smc-it.2009.38.
[9] M. G. Trainer et al., “Seasonal Variations in Atmospheric Composition as Measured in Gale Crater, Mars,” Journal of Geophysical Research: Planets, vol. 124, no. 11. American Geophysical Union (AGU), pp. 3000–3024, Nov. 2019. doi: 10.1029/2019je006175.
[10] L. M. Calle, W. Li, J. W. Buhrow, M. R. Johansen, and C. I. Calle, “Corrosion on Mars: An Investigation of Corrosion under Relevant Simulated Martian Environments,” in 48th International Conference on Environmental Systems, 2018.
[11] D. M. Hassler et al., “Mars’ Surface Radiation Environment Measured with the Mars Science Laboratory’s Curiosity Rover,” Science, vol. 343, no. 6169. American Association for the Advancement of Science (AAAS), Jan. 24, 2014. doi: 10.1126/science.1244797.
[12] B. M. Jakosky and R. J. Phillips, “Mars’ volatile and climate history,” Nature, vol. 412, no. 6843. Springer Science and Business Media LLC, pp. 237–244, Jul. 2001. doi: 10.1038/35084184.
[13] J. A. Whiteway et al., “Mars Water-Ice Clouds and Precipitation,” Science, vol. 325, no. 5936. American Association for the Advancement of Science (AAAS), pp. 68–70, Jul. 03, 2009. doi: 10.1126/science.1172344.
[14] H. Wang, “Martian clouds observed by Mars Global Surveyor Mars Orbiter Camera,” Journal of Geophysical Research, vol. 107, no. E10. American Geophysical Union (AGU), 2002. doi: 10.1029/2001je001815.
[15] M. A. Mischna and S. Piqueux, “The role of atmospheric pressure on Mars surface properties and early Mars climate modeling,” Icarus, vol. 342. Elsevier BV, p. 113496, May 2020. doi: 10.1016/j.icarus.2019.113496.
[16] S. Clifford, “The State and Future of Mars Polar Science and Exploration,” Icarus, vol. 144, no. 2. Elsevier BV, pp. 210–242, Apr. 2000. doi: 10.1006/icar.1999.6290.
[17] C. R. Webster et al., “Mars methane detection and variability at Gale crater,” Science, vol. 347, no. 6220. American Association for the Advancement of Science (AAAS), pp. 415–417, Dec. 16, 2014. doi: 10.1126/science.1261713.
[18] C. Oze, “Have olivine, will gas: Serpentinization and the abiogenic production of methane on Mars,” Geophysical Research Letters, vol. 32, no. 10. American Geophysical Union (AGU), 2005. doi: 10.1029/2005gl022691
[19] S. E. Lauro et al., “Multiple subglacial water bodies below the south pole of Mars unveiled by new MARSIS data,” Nature Astronomy, vol. 5, no. 1. Springer Science and Business Media LLC, pp. 63–70, Sep. 28, 2020. doi: 10.1038/s41550-020-1200-6.
[20] Committee on Precursor Measurements Necessary to Support Human Operations on the Surface of Mars, “Physical Environmental Hazards” in Safe on Mars: Precursor Measurements Necessary to Support Human Operations on the Martian Surface, 2002.
[21] I. Molloy and T. F. Stepinski, “Automatic mapping of valley networks on Mars,” Computers & Geosciences, vol. 33, no. 6. Elsevier BV, pp. 728–738, Jun. 2007. doi: 10.1016/j.cageo.2006.09.009.
[22] S. M. Milkovich, “North polar cap of Mars: Polar layered deposit characterization and identification of a fundamental climate signal,” Journal of Geophysical Research, vol. 110, no. E1. American Geophysical Union (AGU), 2005. doi: 10.1029/2004je002349.
[23] A. Gleyzer, M. Denisyuk, A. Rimmer, and Y. Salingar, “A FAST RECURSIVE GIS ALGORITHM FOR COMPUTING STRAHLER STREAM ORDER IN BRAIDED AND NONBRAIDED NETWORKS,” Journal of the American Water Resources Association, vol. 40, no. 4. Wiley, pp. 937–946, Aug. 2004. doi: 10.1111/j.1752-1688.2004.tb01057.x.
[24] F. Stepinski, M. P. Mendenhall, and B. D. Bue, “Machine cataloging of impact craters on Mars,” Icarus, vol. 203, no. 1. Elsevier BV, pp. 77–87, Sep. 2009. doi: 10.1016/j.icarus.2009.04.026.
[25] B. Smith, N. E. Putzig, J. W. Holt, and R. J. Phillips, “An ice age recorded in the polar deposits of Mars,” Science, vol. 352, no. 6289. American Association for the Advancement of Science (AAAS), pp. 1075–1078, May 27, 2016. doi: 10.1126/science.aad6968.
[26] K. Herkenhoff, “Surface Ages and Resurfacing Rates of the Polar Layered Deposits on Mars,” Icarus, vol. 144, no. 2. Elsevier BV, pp. 243–253, Apr. 2000. doi: 10.1006/icar.1999.6287.
[27] R. Castano et al., “Oasis: Onboard autonomous science investigation system for opportunistic rover science,” Journal of Field Robotics, vol. 24, no. 5. Wiley, pp. 379–397, 2007. doi: 10.1002/rob.20192.
[28] R. Francis et al., “AEGIS autonomous targeting for ChemCam on Mars Science Laboratory: Deployment and results of initial science team use,” Science Robotics, vol. 2, no. 7. American Association for the Advancement of Science (AAAS), Jun. 28, 2017. doi: 10.1126/scirobotics.aan4582.
[29] N. Abcouwer et al., “Machine Learning Based Path Planning for Improved Rover Navigation,” 2021 IEEE Aerospace Conference (50100). IEEE, Mar. 06, 2021. doi: 10.1109/aero50100.2021.9438337.
[30] K. Otsu, G. Matheron, S. Ghosh, O. Toupet, and M. Ono, “Fast approximate clearance evaluation for rovers with articulated suspension systems,” Journal of Field Robotics, vol. 37, no. 5. Wiley, pp. 768–785, Jul. 09, 2019. doi: 10.1002/rob.21892.
[31] A. Castano et al., “Automatic detection of dust devils and clouds on Mars,” Machine Vision and Applications, vol. 19, no. 5–6. Springer Science and Business Media LLC, pp. 467–482, Jun. 20, 2007. doi: 10.1007/s00138-007-0081-3.
[32] G. Doran et al., “COSMIC: Content-based Onboard Summarization to Monitor Infrequent Change,” 2020 IEEE Aerospace Conference. IEEE, Mar. 2020. doi: 10.1109/aero47225.2020.9172337.
[33] M. Dundar, B. L. Ehlmann, and E. Leask, “Machine-Learning-Driven New Geologic Discoveries at Mars Rover Landing Sites: Jezero Crater and NE Syrtis.” Wiley, Dec. 18, 2019. doi: 10.1002/essoar.10501294.1
[34] B. Rothrock, R. Kennedy, C. Cunningham, J. Papon, M. Heverly, and M. Ono, “SPOC: Deep Learning-based Terrain Classification for Mars Rover Missions,” AIAA SPACE 2016. American Institute of Aeronautics and Astronautics, Sep. 09, 2016. doi: 10.2514/6.2016-5539.
[35] M. Ono et al., “MAARS: Machine learning-based Analytics for Automated Rover Systems,” 2020 IEEE Aerospace Conference. IEEE, Mar. 2020. doi: 10.1109/aero47225.2020.9172271
[36] R. M. Swan et al., “AI4MARS: A Dataset for Terrain-Aware Autonomous Driving on Mars,” 2021 IEEE/CVF Conference on Computer Vision and Pattern Recognition Workshops (CVPRW). IEEE, Jun. 2021. doi: 10.1109/cvprw53098.2021.00226.
[37] R. Boumghar, L. Lucas, and A. Donati, “Machine Learning in Operations for the Mars Express Orbiter,” 2018 SpaceOps Conference. American Institute of Aeronautics and Astronautics, May 25, 2018. doi: 10.2514/6.2018-2551.
[38] M. Petkovic et al., “Machine Learning for Predicting Thermal Power Consumption of the Mars Express Spacecraft,” IEEE Aerospace and Electronic Systems Magazine, vol. 34, no. 7. Institute of Electrical and Electronics Engineers (IEEE), pp. 46–60, Jul. 01, 2019. doi: 10.1109/maes.2019.2915456.
[39] D. Qiu et al., “SCOTI: Science Captioning of Terrain Images for data prioritization and local image search,” Planetary and Space Science, vol. 188. Elsevier BV, p. 104943, Sep. 2020. doi: 10.1016/j.pss.2020.104943.
[40] S. Higa et al., “Vision-Based Estimation of Driving Energy for Planetary Rovers Using Deep Learning and Terramechanics,” IEEE Robotics and Automation Letters, vol. 4, no. 4. Institute of Electrical and Electronics Engineers (IEEE), pp. 3876–3883, Oct. 2019. doi: 10.1109/lra.2019.2928765.
[41] S. Ghosh, K. Otsu, and M. Ono, “Probabilistic Kinematic State Estimation for Motion Planning of Planetary Rovers,” 2018 IEEE/RSJ International Conference on Intelligent Robots and Systems (IROS). IEEE, Oct. 2018. doi: 10.1109/iros.2018.8593771.
[42] W. Ji et al., “Extreme Learning Machine for Robustness Enhancement of Gas Detection Based on Tunable Diode Laser Absorption Spectroscopy.” MDPI AG, Dec. 28, 2018. doi: 10.20944/preprints201812.0331.v1.
[43] W. Ji et al., “Extreme Learning Machine for Robustness Enhancement of Gas Detection Based on Tunable Diode Laser Absorption Spectroscopy.” MDPI AG, Dec. 28, 2018. doi: 10.20944/preprints201812.0331.v1.
[44] A. Rashno, B. Nazari, S. Sadri, and M. Saraee, “Effective pixel classification of Mars images based on ant colony optimization feature selection and extreme learning machine,” Neurocomputing, vol. 226. Elsevier BV, pp. 66–79, Feb. 2017. doi: 10.1016/j.neucom.2016.11.030.
[45] R. Zurek, “Polar Layered Terrains: Links Between the Martian Volatile and Dust Cycles,” in The 5th International Conference on Mars, 1999.
[46] T. F. and R. Vilalt, “Machine Learning Tools for Geomorphic Mapping of Planetary Surfaces,” Machine Learning. InTech, Feb. 01, 2010. doi: 10.5772/9146.
[47] C. Juliani and E. Juliani, “Deep learning of terrain morphology and pattern discovery via network-based representational similarity analysis for deep-sea mineral exploration,” Ore Geology Reviews, vol. 129. Elsevier BV, p. 103936, Feb. 2021. doi: 10.1016/j.oregeorev.2020.103936.
[48] F. Goesmann et al., “The Mars Organic Molecule Analyzer (MOMA) Instrument: Characterization of Organic Material in Martian Sediments,” Astrobiology, vol. 17, no. 6–7. Mary Ann Liebert Inc, pp. 655–685, Jul. 2017. doi: 10.1089/ast.2016.1551.
[49] V. Da-Poian, E. Lyness, W. Brinckerhoff, R. Danell, X. Li, and M. Trainer, “Science Autonomy and the ExoMars Mission: Machine Learning to Help Find Life on Mars,” Goldschmidt Abstracts. Geochemical Society, 2020. doi: 10.46427/gold2020.522.