Unsupervised Context-Based Probabilistic Text Classification
Ananya Srivastava1 , Lavanya Gunasekar2 , Bagya Lakshmi V.3 , Navneeth Devaraj4
Section:Research Paper, Product Type: Journal Paper
Volume-9 ,
Issue-12 , Page no. 9-14, Dec-2021
CrossRef-DOI: https://doi.org/10.26438/ijcse/v9i12.914
Online published on Dec 31, 2021
Copyright © Ananya Srivastava, Lavanya Gunasekar, Bagya Lakshmi V., Navneeth Devaraj . This is an open access article distributed under the Creative Commons Attribution License, which permits unrestricted use, distribution, and reproduction in any medium, provided the original work is properly cited.
View this paper at Google Scholar | DPI Digital Library
How to Cite this Paper
- IEEE Citation
- MLA Citation
- APA Citation
- BibTex Citation
- RIS Citation
IEEE Style Citation: Ananya Srivastava, Lavanya Gunasekar, Bagya Lakshmi V., Navneeth Devaraj, “Unsupervised Context-Based Probabilistic Text Classification,” International Journal of Computer Sciences and Engineering, Vol.9, Issue.12, pp.9-14, 2021.
MLA Style Citation: Ananya Srivastava, Lavanya Gunasekar, Bagya Lakshmi V., Navneeth Devaraj "Unsupervised Context-Based Probabilistic Text Classification." International Journal of Computer Sciences and Engineering 9.12 (2021): 9-14.
APA Style Citation: Ananya Srivastava, Lavanya Gunasekar, Bagya Lakshmi V., Navneeth Devaraj, (2021). Unsupervised Context-Based Probabilistic Text Classification. International Journal of Computer Sciences and Engineering, 9(12), 9-14.
BibTex Style Citation:
@article{Srivastava_2021,
author = {Ananya Srivastava, Lavanya Gunasekar, Bagya Lakshmi V., Navneeth Devaraj},
title = {Unsupervised Context-Based Probabilistic Text Classification},
journal = {International Journal of Computer Sciences and Engineering},
issue_date = {12 2021},
volume = {9},
Issue = {12},
month = {12},
year = {2021},
issn = {2347-2693},
pages = {9-14},
url = {https://www.ijcseonline.org/full_paper_view.php?paper_id=5420},
doi = {https://doi.org/10.26438/ijcse/v9i12.914}
publisher = {IJCSE, Indore, INDIA},
}
RIS Style Citation:
TY - JOUR
DO = {https://doi.org/10.26438/ijcse/v9i12.914}
UR - https://www.ijcseonline.org/full_paper_view.php?paper_id=5420
TI - Unsupervised Context-Based Probabilistic Text Classification
T2 - International Journal of Computer Sciences and Engineering
AU - Ananya Srivastava, Lavanya Gunasekar, Bagya Lakshmi V., Navneeth Devaraj
PY - 2021
DA - 2021/12/31
PB - IJCSE, Indore, INDIA
SP - 9-14
IS - 12
VL - 9
SN - 2347-2693
ER -
![]() |
![]() |
![]() |
441 | 447 downloads | 162 downloads |
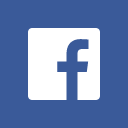
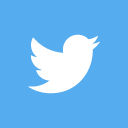
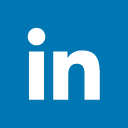
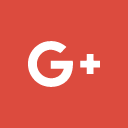
Abstract
Text classification is the one of the primary tasks in Natural Language Processing (NLP). Key phrase extraction is the fundamental component that aids the mapping of documents to a set of emblematic phrases. For example, a category that includes IT documents can be described as “Information and Computer” or “Information and Technology”. If a text document includes keywords such as “issue” and “order”, then it belongs to “Issue Category”. Multiple pre-trained and deep learning approaches are available now-a-days for semantic analysis. Word embeddings are predominant technique that provides light to find the semantic similarity between tokens/phrases using word vectors. The most widely used word embeddings are GloVe, Word2vec, BERT etc. Experimental results show that the strategy produced by this study have more precision and simplicity than that of other methods.
Key-Words / Index Term
Document Categorization, Keywords Extraction, Concept Learning, Multi-class Probabilistic Classification, Content Mining
References
[1] An Jiyuan and Chen, Yi?Ping Phoebe 2005, "Keyword text extraction for text categorization", in Proceedings of the 2005 International Conference on Active Media Technology, pp.556?56 June 2005
[2] Bhumika, Prof Sehra SS, Prof Nayyar A, “A review paper on algorithms used for text classification,” International Journal of Application or Innovation in Engineering & Management (IJAIEM), vol. 2, Issue 3, March 2013.
[3] R Shrihari C, Desai A, “A review on knowledge discovery using text classification techniques in text mining,” International Journal of Computer Applications (0975 – 8887), vol. 111 – No 6, February 2015.
[4] Dang, S., & Ahmad, P.H ,”A Review of Text Mining Techniques Associated with Various Application Areas”, International Journal of Science and Research (IJSR), Vol.4, No.2, pp.2461-2466,2015.
[5] Mehmet Fatih KARACA and Safak BAYIR "Examining the Impact of Feature Selection Methods on Text Classification" Published by : (IJACSA) International Journal of Advanced Computer Science and Applications, Vol. 8, No. 12 , 2017.
[6] R. Janani1, S. Vijayarani "Text Classification: A Comparative Analysis of Word Embedding Algorithms", International Journal of Computer Sciences and Engineering, Vol.7, Issue-4, April 2019.
[7] Riya Tyagi "Review of Extraction and Classification of Key-Phrases in Scientific Publications using CRF and WEDP" Published by : International Journal of Engineering Research & Technology, Volume 09, Paper ID : IJERTV9IS060480, 2020.
[8] Rafly Indra and Abba Suganda Girsang "Classification of User Comment Using Word2vec and Deep Learning" Published by : Advances in Science, Technology and Engineering Systems Journal, Volume 9 No.1 , Page 643– 648 , 2021.
[9] S. Sreedhar, S. Ahmed, P. Flora, LS Hemanth, J. Aishwarya, R. Naik "An Improved Approach of Unstructured Text Document Classification Using Predetermined Text Model and Probability Technique",Published by : International Journal of Recent Technology and Engineering, ISSN: 2277-3878, Volume-8 Issue-2S10. January 2021.