A Transfer Learning-Based Efficient Deep-Learning Methodology for Multi-Class Classification of Endoscopy Frames
Madhura Prakash M.1 , L. Krishnamurthy G.N.2
Section:Research Paper, Product Type: Journal Paper
Volume-10 ,
Issue-10 , Page no. 23-28, Oct-2022
CrossRef-DOI: https://doi.org/10.26438/ijcse/v10i10.2328
Online published on Oct 31, 2022
Copyright © Madhura Prakash M., L. Krishnamurthy G.N. . This is an open access article distributed under the Creative Commons Attribution License, which permits unrestricted use, distribution, and reproduction in any medium, provided the original work is properly cited.
View this paper at Google Scholar | DPI Digital Library
How to Cite this Paper
- IEEE Citation
- MLA Citation
- APA Citation
- BibTex Citation
- RIS Citation
IEEE Style Citation: Madhura Prakash M., L. Krishnamurthy G.N., “A Transfer Learning-Based Efficient Deep-Learning Methodology for Multi-Class Classification of Endoscopy Frames,” International Journal of Computer Sciences and Engineering, Vol.10, Issue.10, pp.23-28, 2022.
MLA Style Citation: Madhura Prakash M., L. Krishnamurthy G.N. "A Transfer Learning-Based Efficient Deep-Learning Methodology for Multi-Class Classification of Endoscopy Frames." International Journal of Computer Sciences and Engineering 10.10 (2022): 23-28.
APA Style Citation: Madhura Prakash M., L. Krishnamurthy G.N., (2022). A Transfer Learning-Based Efficient Deep-Learning Methodology for Multi-Class Classification of Endoscopy Frames. International Journal of Computer Sciences and Engineering, 10(10), 23-28.
BibTex Style Citation:
@article{M._2022,
author = {Madhura Prakash M., L. Krishnamurthy G.N.},
title = {A Transfer Learning-Based Efficient Deep-Learning Methodology for Multi-Class Classification of Endoscopy Frames},
journal = {International Journal of Computer Sciences and Engineering},
issue_date = {10 2022},
volume = {10},
Issue = {10},
month = {10},
year = {2022},
issn = {2347-2693},
pages = {23-28},
url = {https://www.ijcseonline.org/full_paper_view.php?paper_id=5523},
doi = {https://doi.org/10.26438/ijcse/v10i10.2328}
publisher = {IJCSE, Indore, INDIA},
}
RIS Style Citation:
TY - JOUR
DO = {https://doi.org/10.26438/ijcse/v10i10.2328}
UR - https://www.ijcseonline.org/full_paper_view.php?paper_id=5523
TI - A Transfer Learning-Based Efficient Deep-Learning Methodology for Multi-Class Classification of Endoscopy Frames
T2 - International Journal of Computer Sciences and Engineering
AU - Madhura Prakash M., L. Krishnamurthy G.N.
PY - 2022
DA - 2022/10/31
PB - IJCSE, Indore, INDIA
SP - 23-28
IS - 10
VL - 10
SN - 2347-2693
ER -
![]() |
![]() |
![]() |
289 | 372 downloads | 143 downloads |
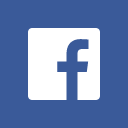
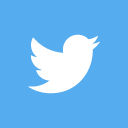
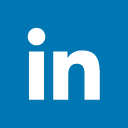
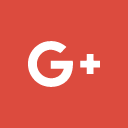
Abstract
Computer-aided detection and analysis of anatomical structures and pathology with the support of Artificial Intelligence aids the medical experts by contributing to better utilization of the expert’s focus time. The gastrointestinal (GI) tract can be assessed for the presence of several irregularities like ulcers, bleeding, inflammation, polyps, and tumor that can be present in several diseases ranging from precancerous lesions to cancer. These abnormalities differ in their appearance by having a different shape, size variation, color and texture differences, and they generally show up to be outwardly comparable to the regular regions in the GI tract. This presents a challenge in designing an efficient classifier that can handle intra-class variations. An endoscopy procedure is performed to detect and diagnose GI abnormalities and to observe the GI pathology. A sequence of video frames of the GI region is captured during the investigation of the tract. A flexible tube with a camera-fitted at the end is injected into the patient’s body through the oral or rectum during the procedure. The frames captured can be analyzed for abnormality classification and lesion segmentation. The analysis is challenging because the frames may have low contrast, uniform background, color variations and indefinite lesion shapes. This makes the segmentation and classification on these frames a challenging task. In this effort, a transfer learning-based deep learning architecture has been employed for performing the multi-class classification of endoscopy frames. The proposed model has been trained and tested on the widely available KVASIR dataset and an average accuracy of 81% has been achieved.
Key-Words / Index Term
Gastrointestinal tract, Transfer Learning, Deep Learning architecture, Classification
References
[1] Konstantin Pogorelov, Kristin Ranheim Randel, Carsten Griwodz, Sigrun Losada Eskeland, Thomas de Lange, Dag Johansen, Concetto Spampinato, Duc-Tien Dang-Nguyen, Mathias Lux, Peter Thelin Schmidt, Michael Riegler, and Pål Halvorsen. 2017. KVASIR: A Multi-Class Image Dataset for Computer Aided Gastrointestinal Disease Detection. In Proceedings of the 8th ACM on Multimedia Systems Conference (MMSys`17). Association for Computing Machinery, New York, NY, USA, 164–169, 2017.
[2] Khan, U.A., Javed, A. & Ashraf, R. An effective hybrid framework for content based image retrieval (CBIR). Multimed Tools Appl 80, 26911–26937, 2021.
[3] Valério, Maria & Gomes, Sara & Salgado, Marta & Oliveira, Helder & Cunha, António. (2019). Lesions Multiclass Classification in Endoscopic Capsule Frames. Procedia Computer Science. 164. 637-645, 2019.
[4] Vasilakakis, M.D., Diamantis, D., Spyrou, E. et al. Weakly supervised multilabel classification for semantic interpretation of endoscopy video frames. Evolving Systems 11, 409–421, 2020.
[5] Suman, S.; Hussin, F.A.; Malik, A.S.; Ho, S.H.; Hilmi, I.; Leow, A.H.-R.; Goh, K.-L. Feature Selection and Classification of Ulcerated Lesions Using Statistical Analysis for WCE Images. Appl. Sci., 07,1097, 2017.
[6] F. Rustam et al., "Wireless Capsule Endoscopy Bleeding Images Classification Using CNN Based Model," in IEEE Access, Vol. 9, pp. 33675-33688, 2021.
[7] Jain, S., Seal, A., & Ojha, A. (2022). A Hybrid Convolutional Neural Network with Meta Feature Learning for Abnormality Detection in Wireless Capsule Endoscopy Images. ArXiv, abs/2207.09769, 2022.
[8] Mehshan Ahmed Khan, Muhammad Attique Khan, Fawad Ahmed, Mamta Mittal, Lalit Mohan Goyal, D. Jude Hemanth, Suresh Chandra Satapathy, Gastrointestinal diseases segmentation and classification based on duo-deep architectures, Pattern Recognition Letters, ISSN 0167-8655. Vol.131, pp.193-204, 2020.
[9] Journal of Mechanics in Medicine and Biology, automated ulcer and bleeding classification from wce images using multiple features fusion and selectionamna liaqat, muhammad attique khan, jamal hussain shah, muhammad sharif, mussarat yasmin and steven lawrence fernandes, Vol. 18, no. 04.
[10] Mamun, Abdullah & Hossain, Md & Hossain, Motaher & Hasan, Galib. (2019). Discretion Way for Bleeding Detection in Wireless Capsule Endoscopy Images. 1-6. 10.1109/ICASERT.2019.8934589.
[11] Vats, Vanshika, et al. "SURF-SVM based identification and classification of gastrointestinal diseases in wireless capsule endoscopy." arXiv preprint arXiv:2009.01179, 2020.
[12] Benkessirat, Amina & Benblidia, Nadjia & Beghdadi, Azeddine. (2021). Gastrointestinal image classification based on VGG16 and transfer learning. 1-5. 10.1109/ICISAT54145.2021.9678481.
[13] Ahmed, A. (2022). Classification of Gastrointestinal Images Based on Transfer Learning and Denoising Convolutional Neural Networks. In: Saraswat, M., Roy, S., Chowdhury, C., Gandomi, A.H. (eds) Proceedings of International Conference on Data Science and Applications. Lecture Notes in Networks and Systems, Springer, Singapore. Vol 288, 2022.
[14] Ahmad, J., Muhammad, K., Lee, M.Y. et al. Endoscopic Image Classification and Retrieval using Clustered Convolutional Features. J Med Syst 41, 196, 2017.
[15] H. L. Gjestang, S. A. Hicks, V. Thambawita, P. Halvorsen and M. A. Riegler, "A self-learning teacher-student framework for gastrointestinal image classification," 2021 IEEE 34th International Symposium on Computer-Based Medical Systems (CBMS), pp. 539-544, 2021.
[16] Timothy Cogan, Maribeth Cogan, Lakshman Tamil, MAPGI: Accurate identification of anatomical landmarks and diseased tissue in the gastrointestinal tract using deep learning, Computers in Biology and Medicine, Volume 111,2019,103351, ISSN 0010-4825.
[17] Trung-Hieu Hoang, Hai-Dang Nguyen, Viet-Anh Nguyen, Thanh-An Nguyen, Vinh-Tiep Nguyen, and Minh-Triet Tran. 2019. Enhancing Endoscopic Image Classification with Symptom Localization and Data Augmentation. In Proceedings of the 27th ACM International Conference on Multimedia (MM `19). Association for Computing Machinery, New York, NY, USA, 2578–2582, 2019.
[18] Huang, C. H., Wu, H. Y., & Lin, Y. L. (2021). Hardnet-mseg: A simple encoder-decoder polyp segmentation neural network that achieves over 0.9 mean dice and 86 fps. arXiv preprint arXiv:2101.07172.
[19] S. Poudel, Y. J. Kim, D. M. Vo and S. -W. Lee, "Colorectal Disease Classification Using Efficiently Scaled Dilation in Convolutional Neural Network," in IEEE Access, vol. 8, pp. 99227-99238, 2020.
[20] Li, Qiang & Yang, Yingjian & Guo, Yingwei & Li, Wei & Liu, Yang & Liu, Han & Kang, Yan. (2021). Performance Evaluation of Deep Learning Classification Network for Image Features. IEEE Access. PP. 1-1. 10.1109/ACCESS.2020.3048956.
[21] Sarker, I.H. Deep Learning: A Comprehensive Overview on Techniques, Taxonomy, Applications and Research Directions. SN COMPUT. SCI. 2, 420, 2021.