A Comprehensive Survey and Comparison on Story Construction Techniques Using Deep Learning for Scene Recognition
Darapu Uma1 , M.Kamala Kumari2
- Department of CSE, Pragati Engineering College, Surampalem, A.P, India.
- Department of CSE, Adikavi Nannaya University, Rajamahendravaram, A.P, India.
Section:Research Paper, Product Type: Journal Paper
Volume-10 ,
Issue-12 , Page no. 14-22, Dec-2022
CrossRef-DOI: https://doi.org/10.26438/ijcse/v10i12.1422
Online published on Dec 31, 2022
Copyright © Darapu Uma, M.Kamala Kumari . This is an open access article distributed under the Creative Commons Attribution License, which permits unrestricted use, distribution, and reproduction in any medium, provided the original work is properly cited.
View this paper at Google Scholar | DPI Digital Library
How to Cite this Paper
- IEEE Citation
- MLA Citation
- APA Citation
- BibTex Citation
- RIS Citation
IEEE Citation
IEEE Style Citation: Darapu Uma, M.Kamala Kumari, “A Comprehensive Survey and Comparison on Story Construction Techniques Using Deep Learning for Scene Recognition,” International Journal of Computer Sciences and Engineering, Vol.10, Issue.12, pp.14-22, 2022.
MLA Citation
MLA Style Citation: Darapu Uma, M.Kamala Kumari "A Comprehensive Survey and Comparison on Story Construction Techniques Using Deep Learning for Scene Recognition." International Journal of Computer Sciences and Engineering 10.12 (2022): 14-22.
APA Citation
APA Style Citation: Darapu Uma, M.Kamala Kumari, (2022). A Comprehensive Survey and Comparison on Story Construction Techniques Using Deep Learning for Scene Recognition. International Journal of Computer Sciences and Engineering, 10(12), 14-22.
BibTex Citation
BibTex Style Citation:
@article{Uma_2022,
author = {Darapu Uma, M.Kamala Kumari},
title = {A Comprehensive Survey and Comparison on Story Construction Techniques Using Deep Learning for Scene Recognition},
journal = {International Journal of Computer Sciences and Engineering},
issue_date = {12 2022},
volume = {10},
Issue = {12},
month = {12},
year = {2022},
issn = {2347-2693},
pages = {14-22},
url = {https://www.ijcseonline.org/full_paper_view.php?paper_id=5534},
doi = {https://doi.org/10.26438/ijcse/v10i12.1422}
publisher = {IJCSE, Indore, INDIA},
}
RIS Citation
RIS Style Citation:
TY - JOUR
DO = {https://doi.org/10.26438/ijcse/v10i12.1422}
UR - https://www.ijcseonline.org/full_paper_view.php?paper_id=5534
TI - A Comprehensive Survey and Comparison on Story Construction Techniques Using Deep Learning for Scene Recognition
T2 - International Journal of Computer Sciences and Engineering
AU - Darapu Uma, M.Kamala Kumari
PY - 2022
DA - 2022/12/31
PB - IJCSE, Indore, INDIA
SP - 14-22
IS - 12
VL - 10
SN - 2347-2693
ER -
![]() |
![]() |
![]() |
337 | 355 downloads | 157 downloads |
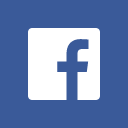
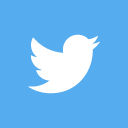
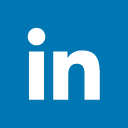
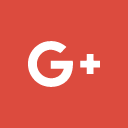
Abstract
Story construction from deep learning is a naive methodology suitable for the digital forensics, smart video surveillance, and intelligent robotics applications. So far deep learning has been utilized for image recognition and classifications. The sequence of those images and classification of them on a temporal basis leads to the development of knowledge on the entire changes in the scenes and finally end up with a story in which the identified scenes are connected with unambiguous changes. This paper retrieves the pros and cons of the research work on recurrent topic transition GAN for Visual Paragraph Generation and relation pair visual paragraph generation. The Existing algorithms proposed for constructing the stories are RTT GAN, RP GAN and BF GAN. These are implemented with respect to different applications human computer interaction, intelligence robotics, digital forensics etc. The survey of the subjected algorithms gives the transparency of their working principles. The current paper presented the visual representation, description, generation of paragraphs with various methodologies along with the comparison.
Key-Words / Index Term
Semantic Region, Attention Module, Discriminator, Scene recognition, Visual features, Generative Adversarial Network
References
[1] X. Liang, Z. Hu, H. Zhang, C. Gan and E. P. Xing, "Recurrent Topic-Transition GAN for Visual Paragraph Generation," IEEE International Conference on Computer Vision (ICCV), pp.3382-3391, 2017.
[2] W. Che, X. Fan, R. Xiong and D. Zhao, "Visual Relationship Embedding Network for Image Paragraph Generation," in IEEE Transactions on Multimedia, Vol.22, no.9, pp.2307-2320, 2020. doi: 10.1109/TMM.2019.2954750.
[3] Z. -J. Zha, D. Liu, H. Zhang, Y. Zhang and F. Wu, "Context-Aware Visual Policy Network for Fine-Grained Image Captioning," in IEEE Transactions on Pattern Analysis and Machine Intelligence, Vol.44, no.2, pp.710-722, 2022. doi: 10.1109/TPAMI.2019.2909864.
[4] D. Liu, J. Fu, Q. Qu and J. Lv, "BFGAN: Backward and Forward Generative Adversarial Networks for Lexically Constrained Sentence Generation," in IEEE/ACM Transactions on Audio, Speech, and Language Processing, Vol 27, no.12, pp.2350-2361, 2019. doi: 10.1109/TASLP.2019.2943018.
[5] K.Kiruba, D. Shiloah Elizabeth, C Sunil Retmin Raj, “Deep Learning for Human Action Recognition – Survey,” International Journal of Computer Sciences and Engineering, Vol.6, Issue.10, pp.323-328, 2018.
[6] B. Prasad, U.K. Devi , “Shape And Texture Based Scene Classification,” International Journal of Computer Sciences and Engineering, Vol.2, Issue.5, pp.79-87, 2014.
[7] Alejandro López-Cifuentes , Marcos Escudero-Viñolo, JesúsBescós, Álvaro García-Martín “Semantic-aware scene recognition” 0031-3203/© 2020 Elsevier.
[8] ” Songhao Zhu *, Yuncai Liu”Automatic scene detection for advanced story retrieval”,Institute of Image Process and Pattern Recognition, Shanghai Jiao Tong University, 800 Dongchuan Road, Shanghai 200240, China.
[9] Ramisa, F. Yan, F. Moreno-Noguer and K. Mikolajczyk, "BreakingNews: Article Annotation by Image and Text Processing," in IEEE Transactions on Pattern Analysis and Machine Intelligence, vol. 40, no. 5, pp. 1072-1085, 2018. doi: 10.1109/TPAMI.2017.2721945
[10] C. P. Chaudhari and S. Devane, "Capturing Semantic Knowledge In Object Localization In Captioning Images," 2021 International Conference on Communication information and Computing Technology (ICCICT), pp.1-4, 2021. doi: 10.1109/ICCICT50803.2021.9510175.
[11] Stanislav Protasov, Adil Mehmood Khan, Konstantin Sozykin & Muhammad AhmadSignal,”Using deep features for video scene detection and annotation”, Image and Video Processing, Vol.12, pp.991–999, 2018.
[12] Y. Choi, S. Kim and J. Lee, "Recurrent Neural Network for Storytelling," 2016 Joint 8th International Conference on Soft Computing and Intelligent Systems (SCIS) and 17th International Symposium on Advanced Intelligent Systems (ISIS), pp. 841-845, 2016. doi: 10.1109/SCIS-ISIS.2016.0182.
[13] P. Haritha, S. Vimala and S. Malathi, "A Systematic Literature Review on Story-Telling for Kids using Image Captioning - Deep Learning," 2020 4th International Conference on Electronics, Communication and Aerospace Technology (ICECA), pp.1588-1593, 2022. doi: 10.1109/ICECA49313.2020.9297457.
[14] H. Zeng, X. Song, G. Chen and S. Jiang, "Learning Scene Attribute for Scene Recognition," in IEEE Transactions on Multimedia, Vol.22, no.6, pp.1519-1530, 2020. doi: 10.1109/TMM.2019.2944241.
[15] H. Seong, J. Hyun and E. Kim, "FOSNet: An End-to-End Trainable Deep Neural Network for Scene Recognition," in IEEE Access, Vol.8, pp.82066-82077, 2020. doi: 10.1109/ACCESS.2020.2989863.
[16] S. Wang, S. Yao, K. Niu, C. Dong, C. Qin and H. Zhuang, "Intelligent Scene Recognition Based on Deep Learning," in IEEE Access, Vol.9, pp.24984-24993, 2021. doi: 10.1109/ACCESS.2021.3057075.
[17] J. Guo, X. Nie and Y. Yin, "Mutual Complementarity: Multi-Modal Enhancement Semantic Learning for Micro-Video Scene Recognition," in IEEE Access, Vol.8, pp.29518-29524, 2020. doi: 10.1109/ACCESS.2020.2973240.
[18] S. Raghunandan, P. Shivakumara, S. Roy, G. H. Kumar, U. Pal and T. Lu, "Multi-Script-Oriented Text Detection and Recognition in Video/Scene/Born Digital Images," in IEEE Transactions on Circuits and Systems for Video Technology, Vol.29, no.4, pp.1145-1162, 2019. doi: 10.1109/TCSVT.2018.2817642.
[19] Chen Wanga,b,? , Guohua Penga , Bernard De Baets “Deep feature fusion through adaptive discriminative metric learning for scene recognition” 1566-2535/© 2020 Elsevier
[20] Lin Xiea,1 , Feifei Leea,1,? , Li Liub , Koji Kotani c , QiuChend, “Scene recognition: A comprehensive survey” , Elsevier Ltd. 0031-3203, 2020.
[21] H. Seong, J. Hyun and E. Kim, "FOSNet: An End-to-End Trainable Deep Neural Network for SceneRecognition," in IEEE Access, Vol.8, pp.82066-82077, 2020. doi: 10.1109/ACCESS.2020.2989863.
[22] A. Jalal, A. Ahmed, A. A. Rafique and K. Kim, "Scene Semantic Recognition Based on Modified Fuzzy C-Mean and Maximum Entropy Using Object-to-Object Relations," in IEEE Access, Vol.9, pp.27758-27772, 2021. doi: 10.1109/ACCESS.2021.3058986.
[23] G. Chen, X. Song, H. Zeng and S. Jiang, "Scene Recognition With Prototype-Agnostic Scene Layout," in IEEE Transactions on Image Processing, Vol.29, pp.5877-5888, 2020. doi: 10.1109/TIP.2020.2986599.
[24] Z. Xiong, Y. Yuan and Q. Wang, "RGB-D Scene Recognition via Spatial-Related Multi-Modal Feature Learning," in IEEE Access, Vol.7, pp.106739-106747, 2019. doi: 10.1109/ACCESS.2019.2932080.
[25] S. Wang, S. Yao, K. Niu, C. Dong, C. Qin and H. Zhuang, "Intelligent Scene Recognition Based on Deep Learning," in IEEE Access, Vol.9, pp.24984-24993, 2021. doi: 10.1109/ACCESS.2021.30570