A Review on Machine Learning Intrusion Detection Systems (MLIDS) in Encrypted Traffic
K.R. Harinath1 , G. Kishore Kumar2
- Dept. of Computer Science and Engineering, JNTUA, Andhra Pradesh, India.
- Dept. of Computer Science and Engineering, RGM College of Engineering and Technology, Andhra Pradesh, India.
Section:Review Paper, Product Type: Journal Paper
Volume-11 ,
Issue-1 , Page no. 1-10, Jan-2023
CrossRef-DOI: https://doi.org/10.26438/ijcse/v11i1.110
Online published on Jan 31, 2023
Copyright © K.R. Harinath, G. Kishore Kumar . This is an open access article distributed under the Creative Commons Attribution License, which permits unrestricted use, distribution, and reproduction in any medium, provided the original work is properly cited.
View this paper at Google Scholar | DPI Digital Library
How to Cite this Paper
- IEEE Citation
- MLA Citation
- APA Citation
- BibTex Citation
- RIS Citation
IEEE Style Citation: K.R. Harinath, G. Kishore Kumar, “A Review on Machine Learning Intrusion Detection Systems (MLIDS) in Encrypted Traffic,” International Journal of Computer Sciences and Engineering, Vol.11, Issue.1, pp.1-10, 2023.
MLA Style Citation: K.R. Harinath, G. Kishore Kumar "A Review on Machine Learning Intrusion Detection Systems (MLIDS) in Encrypted Traffic." International Journal of Computer Sciences and Engineering 11.1 (2023): 1-10.
APA Style Citation: K.R. Harinath, G. Kishore Kumar, (2023). A Review on Machine Learning Intrusion Detection Systems (MLIDS) in Encrypted Traffic. International Journal of Computer Sciences and Engineering, 11(1), 1-10.
BibTex Style Citation:
@article{Harinath_2023,
author = {K.R. Harinath, G. Kishore Kumar},
title = {A Review on Machine Learning Intrusion Detection Systems (MLIDS) in Encrypted Traffic},
journal = {International Journal of Computer Sciences and Engineering},
issue_date = {1 2023},
volume = {11},
Issue = {1},
month = {1},
year = {2023},
issn = {2347-2693},
pages = {1-10},
url = {https://www.ijcseonline.org/full_paper_view.php?paper_id=5538},
doi = {https://doi.org/10.26438/ijcse/v11i1.110}
publisher = {IJCSE, Indore, INDIA},
}
RIS Style Citation:
TY - JOUR
DO = {https://doi.org/10.26438/ijcse/v11i1.110}
UR - https://www.ijcseonline.org/full_paper_view.php?paper_id=5538
TI - A Review on Machine Learning Intrusion Detection Systems (MLIDS) in Encrypted Traffic
T2 - International Journal of Computer Sciences and Engineering
AU - K.R. Harinath, G. Kishore Kumar
PY - 2023
DA - 2023/01/31
PB - IJCSE, Indore, INDIA
SP - 1-10
IS - 1
VL - 11
SN - 2347-2693
ER -
![]() |
![]() |
![]() |
229 | 326 downloads | 98 downloads |
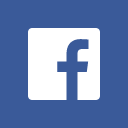
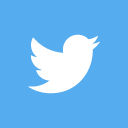
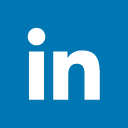
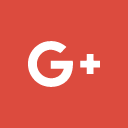
Abstract
Global connection depends on the internet and protecting it is a top priority for organizations and governments. As technology advances, so does the number of different types of network attacks. These attacks can be considered as intrusions. Due to deficiency of protection the information protection becomes onerous. To detect intrusions, a well defined intrusion detection system was utilized. It is one among the tools towards building secure system. To combat with advanced attacks and to protect the data and network, MLIDS (Machine Learning Based Intrusion Detection systems) is an advanced technology among best solutions. When accesses are encrypted, however, IDS is ineffective. Although encryption increases sender and receiver privacy, it causes an issue with inaccurate traffic categorization. There are Several ID approaches to analyse encrypted traffic interchange using data range, data similarity and data time without decryption. In this survey, paper presents a different techniques, datasets and challenges of detection over cipher text and comparative survey on machine learning algorithms from recent work.
Key-Words / Index Term
IDS, Encryption, Encrypted traffic, datasets, intrusions
References
[1]. Khraisat, A., Gondal, I., Vamplew, P., &Kamruzzaman, J. Survey of intrusion detection systems: techniques, datasets and challenges. Cybersecurity, Vol.2, Issue.1, pp.1-22, 2019.
[2]. Gulla, K. K., Viswanath, P., Veluru, S. B., & Kumar, R. R. (2020). Machine learning based intrusion detection techniques. In Handbook of computer networks and cyber security. Springer, Cham, pp.873-888, 2020.
[3]. Kumar, G. K., Viswanath, P., & Rao, A. A. (2011). Intrusion Detection Using an Ensemble of Decision Trees. In IICAI, pp.382-392, 2011.
[4]. Van Engelen, J. E., &Hoos, H. H. (2020). A survey on semi-supervised learning. Machine Learning, Vol.109, Issue.2, pp.373-440, 2020.
[5]. Garcia-Teodoro, P., Diaz-Verdejo, J., Maciá-Fernández, G., &Vázquez, E. (2009). Anomaly-based network intrusion detection: Techniques, systems and challenges. computers & security, Vol.28, Issue.1-2, pp.18-28, 2009.
[6]. Chiba, Z., Abghour, N., Moussaid, K., &Rida, M. (2019). Intelligent approach to build a Deep Neural Network based IDS for cloud environment using combination of machine learning algorithms. Computers & Security, 86, pp.291-317, 2019.
[7]. Siddique, K., Akhtar, Z., Khan, F. A., & Kim, Y. (2019). KDD Cup 99 data sets: a perspective on the role of data sets in network intrusion detection research. Computer, Vol.52, Issue.2, pp.41-51, 2019.
[8]. Kumar, V., Srivastava, J., &Lazarevic, A. (Eds.). (2006). Managing cyber threats: issues, approaches, and challenges. Springer Science & Business Media, Vol.5, 2006.
[9]. Kim, H., Kim, J., Kim, Y., Kim, I., & Kim, K. J. (2019). Design of network threat detection and classification based on machine learning on cloud computing. Cluster Computing, Vol.22, Issue.1, pp.2341-2350, 2019.
[10]. Hatef, M. A., Shaker, V., Jabbarpour, M. R., Jung, J., &Zarrabi, H. (2018). HIDCC: A hybrid intrusion detection approach in cloud computing. Concurrency and Computation: Practice and Experience, Vol.30, Issue.3, e4171, 2018.
[11]. Sultana, N., Chilamkurti, N., Peng, W., &Alhadad, R. (2019). Survey on SDN based network intrusion detection system using machine learning approaches. Peer-to-Peer Networking and Applications, Vol.12, Issue.2, pp.493-501, 2019.
[12]. Hu, J. (2010). Host-based anomaly intrusion detection. In Handbook of information and communication security. Springer, Berlin, Heidelberg, pp.235-255, 2010.
[13]. Liu, M., Xue, Z., Xu, X., Zhong, C., & Chen, J. (2018). Host-based intrusion detection system with system calls: Review and future trends. ACM Computing Surveys (CSUR), Vol.51, Issue.5, 1-36, 2018.
[14]. Ahmed, M., Pal, R., Hossain, M. M., Bikas, M. A. N., &Hasan, M. K. (2009, April). NIDS: A network based approach to intrusion detection and prevention. In 2009 International Association of Computer Science and Information Technology-Spring Conference. IEEE, pp.141-144, 2009.
[15]. Singh, R., Kalra, M., & Solanki, S. (2020). A hybrid approach for intrusion detection based on machine learning. International Journal of Security and Networks, Vol.15, Issue.4, 233-242, 2020.
[16]. Çavu?o?lu, Ü. (2019). A new hybrid approach for intrusion detection using machine learning methods. Applied Intelligence, Vol.49, Issue.7, pp.2735-2761, 2019.
[17]. Ray, S. (2019, February). A quick review of machine learning algorithms. In 2019 International conference on machine learning, big data, cloud and parallel computing (COMITCon). IEEE, pp.35-39, 2019.
[18]. Chaudhari, R. R., &Patil, S. P. (2017). Intrusion detection system: classification, techniques and datasets to implement. Int. Res. J. Eng. Technol.(IRJET), Vol.4, Issue.2, pp.1860-1866, 2017.
[19]. Aljamal, I., Tekeo?lu, A., Bekiroglu, K., &Sengupta, S. (2019, May). Hybrid intrusion detection system using machine learning techniques in cloud computing environments. In 2019 IEEE 17th international conference on software engineering research, management and applications (SERA). IEEE, pp.84-89, 2019.
[20]. Wahyudi, B., Ramli, K., &Murfi, H. (2018). Implementation and analysis of combined machine learning method for intrusion detection system. International Journal of Communication Networks and Information Security, Vol.10, Issue.2, pp.295-304, 2018.
[21]. Yamada, A., Miyake, Y., Takemori, K., Studer, A., &Perrig, A. (2007, May). Intrusion detection for encrypted web accesses. In 21st International Conference on Advanced Information Networking and Applications Workshops (AINAW`07). IEEE, Vol.1, pp.569-576, 2007.
[22]. Li, J., Qu, Y., Chao, F., Shum, H. P., Ho, E. S., & Yang, L. (2019). Machine learning algorithms for network intrusion detection. AI in Cybersecurity, pp.151-179, 2019.
[23]. Mahesh, B. (2020). Machine learning algorithms-a review. International Journal of Science and Research (IJSR).[Internet], 9, pp.381-386, 2020.
[24]. Thaseen, I. S., Poorva, B., &Ushasree, P. S. (2020, February). Network intrusion detection using machine learning techniques. In 2020 International conference on emerging trends in information technology and engineering (IC-ETITE). IEEE, pp.1-7, 2020.
[25]. Kovanen, T., David, G., &Hämäläinen, T. (2016). Survey: Intrusion detection systems in encrypted traffic. In Internet of Things, Smart Spaces, and Next Generation Networks and Systems. Springer, Cham, pp.281-293, 2016.
[26]. Koch, R., &Rodosek, G. D. (2010, September). Command evaluation in encrypted remote sessions. In 2010 Fourth International Conference on Network and System Security. IEEE, pp.299-305, 2010.
[27]. Sharafaldin, I., Lashkari, A. H., &Ghorbani, A. A. (2018). Toward generating a new intrusion detection dataset and intrusion traffic characterization. ICISSp, 1, pp.108-116, 2018.
[28]. Creech, G. (2014). Developing a high-accuracy cross platform Host-Based Intrusion Detection System capable of reliably detecting zero-day attacks (Doctoral dissertation, University of New South Wales, Canberra, Australia). 2014.
[29]. Shiravi, A., Shiravi, H., Tavallaee, M., &Ghorbani, A. A. (2012). Toward developing a systematic approach to generate benchmark datasets for intrusion detection. computers& security, Vol.31, Issue.3, pp.357-374, 2012.
[30]. Tavallaee, M., Bagheri, E., Lu, W., &Ghorbani, A. A. (2009, July). A detailed analysis of the KDD CUP 99 data set. In 2009 IEEE symposium on computational intelligence for security and defense applications. Ieee, pp.1-6, 2009.
[31]. Hendry, G. R., & Yang, S. J. (2008, March). Intrusion signature creation via clustering anomalies. In Data Mining, Intrusion Detection, Information Assurance, and Data Networks Security 2008. International Society for Optics and Photonics. Vol.6973, p.69730C, 2008.
[32]. Butun, I., Morgera, S. D., &Sankar, R. (2013). A survey of intrusion detection systems in wireless sensor networks. IEEE communications surveys & tutorials, Vol.16, Issue.1, pp.266-282, 2013.
[33]. Ye, N., Emran, S. M., Chen, Q., &Vilbert, S. (2002). Multivariate statistical analysis of audit trails for host-based intrusion detection. IEEE Transactions on computers, Vol.51, Issue.7, pp.810-820, 2002.
[34]. Walkinshaw, N., Taylor, R., & Derrick, J. (2016). Inferring extended finite state machine models from software executions. Empirical Software Engineering, Vol.21, Issue.3, pp.811-853, 2016.
[35]. Dua, S., & Du, X. (2016). Data mining and machine learning in cybersecurity. CRC press. 2016.
[36]. Celebi, M. E., & Aydin, K. (Eds.). (2016). Unsupervised learning algorithms. Berlin: Springer International Publishing. 2016.
[37]. Sinaga, K. P., & Yang, M. S. (2020). Unsupervised K-means clustering algorithm. IEEE access, 8, pp.80716-80727, 2020.
[38]. SeyedTabatabaei, T., Adel, M., Karray, F., &Kamel, M. (2012, July). Machine learning-based classification of encrypted internet traffic. In International Workshop on Machine Learning and Data Mining in Pattern Recognition. Springer, Berlin, Heidelberg. pp.578-592, 2012.
[39]. Koch, R., Golling, M., &Rodosek, G. D. (2014). Behavior-based intrusion detection in encrypted environments. IEEE Communications Magazine, Vol.52, Issue.7, pp.124-131, 2014.
[40]. Cha, S., & Kim, H. (2016, August). Detecting encrypted traffic: a machine learning approach. In International Workshop on Information Security Applications. Springer, Cham. pp.54-65, 2016.
[41]. Wang, P., Chen, X., Ye, F., & Sun, Z. (2019). A survey of techniques for mobile service encrypted traffic classification using deep learning. IEEE Access, 7, pp.54024-54033, 2019.
[42]. Rezaei, S., & Liu, X. (2019). Deep learning for encrypted traffic classification: An overview. IEEE communications magazine, Vol.57, Issue.5, pp.76-81, 2019.
[43]. Karaçay, L., Sava?, E., &Alptekin, H. (2020). Intrusion detection over encrypted network data. The Computer Journal. Papadogiannaki, E., & Ioannidis, S. (2021). Vol.63, Issue.4, pp.604-619, 2020.
[44]. Acceleration of intrusion detection in encrypted network traffic using heterogeneous hardware. Sensors, 21(4), 1140.