Facial Emotion Recognition Based Prediction of Affective State Of Children with Autism Using Ml
Sujith Kumar R.1 , Soundar Sriram J.2 , Sridhar C.3 , Fathima G.4
- Dept. of CSE, Adhiyamaan College of Engineering, Hosur, Tamilnadu, India.
- Dept. of CSE, Adhiyamaan College of Engineering, Hosur, Tamilnadu, India.
- Dept. of CSE, Adhiyamaan College of Engineering, Hosur, Tamilnadu, India.
- Dept. of CSE, Adhiyamaan College of Engineering, Hosur, Tamilnadu, India.
Section:Research Paper, Product Type: Journal Paper
Volume-11 ,
Issue-4 , Page no. 19-25, Apr-2023
CrossRef-DOI: https://doi.org/10.26438/ijcse/v11i4.1925
Online published on Apr 30, 2023
Copyright © Sujith Kumar R., Soundar Sriram J., Sridhar C., Fathima G. . This is an open access article distributed under the Creative Commons Attribution License, which permits unrestricted use, distribution, and reproduction in any medium, provided the original work is properly cited.
View this paper at Google Scholar | DPI Digital Library
How to Cite this Paper
- IEEE Citation
- MLA Citation
- APA Citation
- BibTex Citation
- RIS Citation
IEEE Citation
IEEE Style Citation: Sujith Kumar R., Soundar Sriram J., Sridhar C., Fathima G., “Facial Emotion Recognition Based Prediction of Affective State Of Children with Autism Using Ml,” International Journal of Computer Sciences and Engineering, Vol.11, Issue.4, pp.19-25, 2023.
MLA Citation
MLA Style Citation: Sujith Kumar R., Soundar Sriram J., Sridhar C., Fathima G. "Facial Emotion Recognition Based Prediction of Affective State Of Children with Autism Using Ml." International Journal of Computer Sciences and Engineering 11.4 (2023): 19-25.
APA Citation
APA Style Citation: Sujith Kumar R., Soundar Sriram J., Sridhar C., Fathima G., (2023). Facial Emotion Recognition Based Prediction of Affective State Of Children with Autism Using Ml. International Journal of Computer Sciences and Engineering, 11(4), 19-25.
BibTex Citation
BibTex Style Citation:
@article{R._2023,
author = {Sujith Kumar R., Soundar Sriram J., Sridhar C., Fathima G.},
title = {Facial Emotion Recognition Based Prediction of Affective State Of Children with Autism Using Ml},
journal = {International Journal of Computer Sciences and Engineering},
issue_date = {4 2023},
volume = {11},
Issue = {4},
month = {4},
year = {2023},
issn = {2347-2693},
pages = {19-25},
url = {https://www.ijcseonline.org/full_paper_view.php?paper_id=5552},
doi = {https://doi.org/10.26438/ijcse/v11i4.1925}
publisher = {IJCSE, Indore, INDIA},
}
RIS Citation
RIS Style Citation:
TY - JOUR
DO = {https://doi.org/10.26438/ijcse/v11i4.1925}
UR - https://www.ijcseonline.org/full_paper_view.php?paper_id=5552
TI - Facial Emotion Recognition Based Prediction of Affective State Of Children with Autism Using Ml
T2 - International Journal of Computer Sciences and Engineering
AU - Sujith Kumar R., Soundar Sriram J., Sridhar C., Fathima G.
PY - 2023
DA - 2023/04/30
PB - IJCSE, Indore, INDIA
SP - 19-25
IS - 4
VL - 11
SN - 2347-2693
ER -
![]() |
![]() |
![]() |
323 | 358 downloads | 160 downloads |
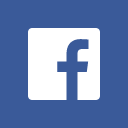
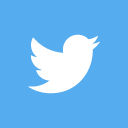
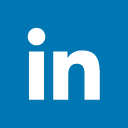
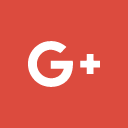
Abstract
Nonverbal communication is important in everyday interactions, and its contribution to communication can be as high as 93%. Video surveillance, expression analysis, paralinguistic communication, and detection all benefit from the application of facial emotion analysis. We provide a thorough description of FER (Facial Emotion Recognition), which is based on traditional machine learning (ML), in our suggested system. In-depth research is required to make learning problems detection simpler because it is still laborious and time-consuming. Dyscalculia is characterised by difficulties counting, comparing numbers, and adding mathematical operations. This learning disability is thought to affect between 3 and 6% of school-aged youngsters. One of the special learning disabilities (SLD) with a mathematical impairment is dyscalculia. As the results of these individual tests alone are insufficient for identification, a variety of tests must be administered and analysed manually in order to discover dyscalculia. When analysing complex medical data, artificial intelligence (AI) for healthcare uses Random Forest algorithms to simulate human cognition. The screening procedure for these particular learning problems makes use of machine learning techniques. Counting accuracy, time spent per question during the counting phase, number comparison accuracy, time spent per question during the number comparison phase, arithmetic addition accuracy, and time spent per question during the addition phase were the six inputs used to develop the model. The model, which categorises children as dyscalculic or not, was constructed using the Random Forest method.
Key-Words / Index Term
Facial emotion recognition (FER), Artificial intelligence, Random Forest, and dyscalculia.
References
[1]. T. Booth, A. L. Murray, K. McKenzie, R. Kuenssberg, M. O’Donnell, and H. Burnett, “An evaluation of the as a brief screening instrument for asd in adults”, 2020.
[2]. Sluban, B., Jursic, M., Cestnik, B., Lavrac, N. (2021). Exploring the power of outliers for cross-domain literature mining. In M. R. Berthold (Ed.), Bisociative knowledge discovery.
[3]. I. Petric, T. Urbancic, and B. Cestnik, “Discovering hidden knowledge from biomedical literature,” Informatica, 2022.
[4]. D. Liao and H. Lu, “Classify autism and control based on deep learning and community structure on resting-state fmri,” in 2018 Tenth Inter-national Conference on Advanced Computational Intelligence.
[5]. N. A. M. Yuzaidey, N. C. Din, M. Ahmad, N. Ibrahim, R. A. Razak, and D. Harun, ‘‘Interventions for children with dyslexia: A review on current intervention methods,’’ Med. J. Malaysia, Oct. 2019.
[6]. B. Sarah, C. Nicole, H. Ardag, M. Madelyn, S. K. Holland, and H. Tzipi, ‘‘An fMRI Study of a Dyslexia biomarker,’’ J. Young Investigators, 2019.
[7]. Association International Dyslexia, Baltimore, MD, USA. (2019). Dyslexia in the Classroom—What Every Teacher Needs to Know.
[8]. N. A. M. Yuzaidey, N. C. Din, M. Ahmad, N. Ibrahim, R. A. Razak, and D. Harun, ‘‘Interventions for children with dyslexia: A review on current intervention methods,’’ Med. J. Malaysia, 2020.
[9]. S. Kaisar, ‘‘Developmental dyslexia detection using machine learning techniques: A survey,’’ ICT Exp.
[10]. F. Ramus, I. Altarelli, K. Jednoróg, J. Zhao, and L. Scotto di Covella, ‘‘Neuroanatomy of developmental dyslexia: Pitfalls and promise,’’ Neurosci. Biobehav., Jan. 2018.
[11]. L. Danelli, M. Berlingeri, G. Bottini, F. Ferri, L. Vacchi, M. Sberna, and E. Paulesu, ‘‘Neural intersections of the phonological, visual magnocellular and motor/cerebellar systems in normal readers: Implications for imaging studies on dyslexia,’’ Hum. Brain Mapping, 2020.
[12]. U. Kuhl, N. E. Neef, I. Kraft, G. Schaadt, L. Dörr, J. Brauer, I. Czepezauer, B. Müller, A. Wilcke, H. Kirsten, F. Emmrich, J. Boltze, A. D. Friederici, and M. A. Skeide, ‘‘The emergence of dyslexia in the developing brain,’’ NeuroImage, vol. 211, May 2020, Art. no. 116633.
[13]. S. M. Irby and R. G. Floyd, ‘‘Test review: Wechsler abbreviated scale of intelligence, second edition,’’ Can. J. School Psychol., vol. 28, no. 3, pp. 295–299, Sep. 2013.
[14]. C. A. Dell, B. Harrold, and T. Dell, ‘‘Test Review: Wilkinson, G. S., & Robertson, G. J. (2006). wide range achievement Test-Fourth Edition. Lutz, FL:
Psychological Assessment Resources. WRAT4 Introductory Kit (includes manual, 25 test/response forms [blue and green], and accompanying test mat,’’ Rehabil. Couns. Bull.
[15]. B. J. Wending, F. A. Schrank, and A. J. Scmitt, ‘‘Educational interventions related to the Woodcock-Johnson III test of achievement,’’ in Woodcock Johnson III Assessment Service Bulletin Number, vol. 8, no. 8. Rolling Meadows IL, USA: Riverside Publishing, A Houghton Mifflin Company, 2019.
[16]. J.-J. Mitchell, ‘‘Comprehensive test of phonological processing,’’ Assessment Effective Intervent, vol. 26, no. 3, pp. 57–63, Apr. 2020.
[17]. R. M. Bruno and S. C. Walker, ‘‘Comprehensive test of phonological processing (CTOPP),’’ Diagnostique, vol. 24, nos. 1–4, pp. 69–82, Mar. 1999.
[18]. N. L. Bell, K. S. Lassiter, T. D. Matthews, and M. B. Hutchinson, ‘‘Comparison of the peabody picture vocabulary test—Third edition and Wechsler adult intelligence scale—Third edition with university students,’’ J. Clin. Psychol.,
[19]. B. Srivastava and M. T. U. Haider, ‘‘Personalized assessment model for alphabets learning with learning objects in e-learning environment for dyslexia,’’ J. King Saud Univ.-Computer.
[20]. A. Alsobhi, N. Khan, and H. Rahanu, ‘‘An empirical approach to validate the dyslexia adaptive E-learning (DAEL) framework,’’ in Proc. 10th Int. Conf. Comput. Sci. Educ. (ICCSE), Jul. 2015.
[21]. Aparna Shenoy, K.V. Viswanatha, Raju Ramakrishna Gondkar, "Early Sepsis Prediction in Intensive Care Patients using Random Forest Classifier", International Journal of Computer Sciences and Engineering, Vol.8, Issue.1, pp.17-22, 2020.
[22]. Rosy Mishra, Y. Sowjanya, Sushanta Meher, Mousumi Meher, "A Voice Signal Interpreter using Machine Learning Techniques," International Journal of Scientific Research in Network Security and Communication, Vol.8, Issue.2, pp.22-27, 2020.