Blood Glucose Monitoring Using Non-Invasive Method Based On IOT
V. Gunavardini1 , B. Vinodhini2 , K. Sangeetha3
- Department of Computer Science and Engineering, Anna University, Chennai, India.
- Department of Computer Science and Engineering, SNS College of Technology, Coimbatore, India.
- Department of Computer Science and Engineering, SNS College of Technology, Coimbatore, India.
Section:Research Paper, Product Type: Journal Paper
Volume-11 ,
Issue-5 , Page no. 34-40, May-2023
CrossRef-DOI: https://doi.org/10.26438/ijcse/v11i5.3440
Online published on May 31, 2023
Copyright © V. Gunavardini, B. Vinodhini, K. Sangeetha . This is an open access article distributed under the Creative Commons Attribution License, which permits unrestricted use, distribution, and reproduction in any medium, provided the original work is properly cited.
View this paper at Google Scholar | DPI Digital Library
How to Cite this Paper
- IEEE Citation
- MLA Citation
- APA Citation
- BibTex Citation
- RIS Citation
IEEE Style Citation: V. Gunavardini, B. Vinodhini, K. Sangeetha, “Blood Glucose Monitoring Using Non-Invasive Method Based On IOT,” International Journal of Computer Sciences and Engineering, Vol.11, Issue.5, pp.34-40, 2023.
MLA Style Citation: V. Gunavardini, B. Vinodhini, K. Sangeetha "Blood Glucose Monitoring Using Non-Invasive Method Based On IOT." International Journal of Computer Sciences and Engineering 11.5 (2023): 34-40.
APA Style Citation: V. Gunavardini, B. Vinodhini, K. Sangeetha, (2023). Blood Glucose Monitoring Using Non-Invasive Method Based On IOT. International Journal of Computer Sciences and Engineering, 11(5), 34-40.
BibTex Style Citation:
@article{Gunavardini_2023,
author = {V. Gunavardini, B. Vinodhini, K. Sangeetha},
title = {Blood Glucose Monitoring Using Non-Invasive Method Based On IOT},
journal = {International Journal of Computer Sciences and Engineering},
issue_date = {5 2023},
volume = {11},
Issue = {5},
month = {5},
year = {2023},
issn = {2347-2693},
pages = {34-40},
url = {https://www.ijcseonline.org/full_paper_view.php?paper_id=5573},
doi = {https://doi.org/10.26438/ijcse/v11i5.3440}
publisher = {IJCSE, Indore, INDIA},
}
RIS Style Citation:
TY - JOUR
DO = {https://doi.org/10.26438/ijcse/v11i5.3440}
UR - https://www.ijcseonline.org/full_paper_view.php?paper_id=5573
TI - Blood Glucose Monitoring Using Non-Invasive Method Based On IOT
T2 - International Journal of Computer Sciences and Engineering
AU - V. Gunavardini, B. Vinodhini, K. Sangeetha
PY - 2023
DA - 2023/05/31
PB - IJCSE, Indore, INDIA
SP - 34-40
IS - 5
VL - 11
SN - 2347-2693
ER -
![]() |
![]() |
![]() |
135 | 192 downloads | 104 downloads |
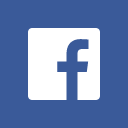
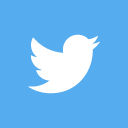
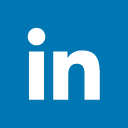
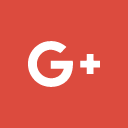
Abstract
The current standard of diabetes management depends upon invasive blood pricking techniques. In recent times, continuous glucose monitoring devices have made some improvements in the life of diabetic patients. These proposed techniques have the potential to evolve into a wearable device for non-invasive diabetes management. The conventional Blood Glucose Monitoring (BGM) techniques currently used for the collection of blood samples through finger pricks make the process painful with the risk of infection. In recent years, researchers focus more on making BGM non-invasive under near infrared (NIR) rays. In Intensive Care Unit (ICU) the patients who are critically ill are admitted for the treatment and the Doctors need an all-time update patient’s health related parameters like heart pulse and temperature. Manually doing this is a tedious task for the multiple patients. For this, IOT based system can bring about an automation that keep the Doctors updated all time over the internet. IOT Based ICU Patient Monitoring System is arduino based system which have collects patient’s information with the help of few sensors and uses Wifi module to communicate the information to the internet where the heart beat pulse sensor and heart beat monitor module are electrically connected to the system and physically to be worn by the user. Thus, the doctor can get access to these vital parameters of the patient’s health over the IOT Gecko web interface from anywhere in the world.
Key-Words / Index Term
ICU- Intensive Care Unit , BGM-Blood Glucose Monitoring, BGL-Blood Glucose Level, CGM-Continuous Glucose Monitoring, mg/dl-Milligrams per deciliter.
References
[1] H. Esmaily, M. Tayefi, H. Doosti, H. Nezami and A. Amirabadizadeh, "A Comparison between Decision Tree and Random Forest in Determining the Risk Factors Associated with Type 2 Diabetes", J. Res. Health Sci, vol. 18, no. 2, 2018.
[2] Russell O Potts, Janet A. Tamada, and Michael J. Tierney. 2002. Glucose monitoring by reverse iontophoresis. Diabetes/metabolism research and reviews18, S1(2002), S49–S53.
[3]. Georgeanne Purvinis, Brent D Cameron, and Douglas M Altrogge. 2011. Non-invasive polarimetric-based glucose monitoring: an in vivo study. Journal of diabetes science and technology 5, 2 (2011), 380–387.
[4]. Yun Qian, Yanchun Liang, Mu Li, Guoxiang Feng, and Xiaohu Shi. 2014. A resampling ensemble algorithm for classification of imbalance problems. Neurocomputing 143 (2014), 57–67.
[5]. Sanjiv Sharma, Zhenyi Huang, Michelle Rogers, Martyn Boutelle, and AnthonyEG Cass. 2016. Evaluation of a minimally invasive glucose biosensor for continuous tissue monitoring. Analytical and bio analytical chemistry 408, 29 (2016), 8427– 8435.
[6]. MJ Tierney, HL Kim,MDBurns, JA Tamada, and RO Potts. 2000. Electro analysis of glucose in transcutaneously extracted samples. Electro analysis: An International Journal Devoted to Fundamental and Practical Aspects of Electro analysis 12, 9 (2000), 666–671.
[7]. GT Tucker and MS Lennard. 1990. Enantiomer specific pharmacokinetics. Pharmacology & therapeutics 45, 3 (1990), 309–329. 60
[8]. M. Alsalamah, S. Amin and V. Palade, "Clinical Practice for Diagnostic Causes for Obstructive Sleep Apnea Using Atificial Intelligent Neural Networks", International Conference for Emerging Technologies in Computing, pp. 259-272, 2018.
[9]. Sandeep Kumar Vashist. 2012. Non-invasive glucose monitoring technology in diabetes management: A review. Analytica chimica acta 750 (2012), 16–27.
[10]. Sandeep Kumar Vashist, Dan Zheng, Khalid Al-Rubeaan, John HT Luong, and Fwu-Shan Sheu. 2011. Advances in carbon nanotube based electrochemical sensors for bioanalytical applications. Biotechnology advances 29, 2 (2011)
[11]. Jeanette M Waller and Howard I Maibach. 2005. Age and skin structure and function, a quantitative approach (I): blood flow, pH, thickness, and ultrasound echogenicity. Skin research and technology 11, 4 (2005), 221–235.
[12]. Stuart AlanWeinzimer. 2004. Analysis: PENDRA: The Once and Future Non-invasive Continuous Glucose Monitoring Device? Diabetes technology & therapeutics 6, 4 (2004), 442–444.
[13]. W. Chen, S. Chen, H. Zhang and T. Wu, "A hybrid prediction model for type 2 diabetes using K-means and decision tree", Software Engineering and Service Science (ICSESS) 2017 8th IEEE International Conference on, pp. 386-390, 2017.
[14]. Michael FGWood, Nirmalya Ghosh, Xinxin Guo, and I Alex Vitkin. 2008. Towards non-invasive glucose sensing using polarization analysis of multiply scattered light. Handbook of optical sensing of glucose in biological fluids and tissues 12(2008).
[15]. International Diabetes Federation Guideline Development Group Global guideline for type 2 diabetes. Diabetes Res. Clin. Pract. 2014;104:1–52. doi: 10.1016/j.diabres.2012.10.001.
[16]. Alarcón-Paredes A, Francisco-García V, Guzmán-Guzmán IP, Cantillo-Negrete J, Cuevas-Valencia RE, Alonso-Silverio GA. An IoT-based non-invasive glucose level monitoring system using Raspberry Pi. Applied Sciences 2019 Jul 28;9(15):3046.
[17]. J. F. Kaiser, "Some useful properties of Teager`s energy operators," IEEE International Conference on Acoustics, Speech, and Signal Processing, pp. 149- 152 vol.3, 1993. 61
[18]. Lekha S, M S. Real-time non-invasive detection and classification of diabetes using modified convolution neural network. IEEE J Biomed Health Inform 2018 Sep;22(5):1630-1636.
[19]. Y. Chen, D. Li, Y. Li, X. Ma, and J. Wei, “Use moving average filter to reduce noises in wearable PPG during continuous monitoring,” IneHealth 360° Springer, pp. 193-203, 2017.
[20]. P. Fan, H. Peiyu, L. Shangwen, and D. Wenfeng, “Feature extraction of photoplethysmography signal using Wavelet Approach,” IEEE International Conference on Digital Signal Processing (DSP), July. 2015, pp. 283-286.L. Atzori, A. Iera, and G. Morabito, “The internet of things: A survey,” Comput. Netw., vol. 54, no. 15, pp. 2787–2805, 2010.
[21]. X.-H. Meng, Y.-X. Huang, D.-P. Rao, Q. Zhang and Q. Liu, "Comparison of three data mining models for predicting diabetes or prediabetes by risk factors", Kaohsiung J. Med. Sci., vol. 29, no. 2, pp. 93-99, 2013.
[22]. Amir O, Weinstein D, Zilberman S, Less M, Perl-Treves D, Primack H, et al. Continuous noninvasive glucose monitoring technology based on `occlusion spectroscopy`. J Diabetes Sci Technol 2007 Jul;1(4):463-469.