An Analysis of Machine Learning Solution for QoS and QoE in Network (Infrastructure Oriented and Less)
N. Kanimozhi1 , S. Hari Ganesh2 , B. Karthikeyan3
- Department of Computer Science, H.H The Rajah’s College, Pudukkotai – 622 001, India.
- Department of Computer Science, H.H The Rajah’s College, Pudukkotai – 622 001, India.
- Department of Computer Science, Bishop Heber College, Trichy – 620 017, India.
Section:Survey Paper, Product Type: Journal Paper
Volume-11 ,
Issue-5 , Page no. 41-59, May-2023
CrossRef-DOI: https://doi.org/10.26438/ijcse/v11i5.4159
Online published on May 31, 2023
Copyright © N. Kanimozhi, S. Hari Ganesh, B. Karthikeyan . This is an open access article distributed under the Creative Commons Attribution License, which permits unrestricted use, distribution, and reproduction in any medium, provided the original work is properly cited.
View this paper at Google Scholar | DPI Digital Library
How to Cite this Paper
- IEEE Citation
- MLA Citation
- APA Citation
- BibTex Citation
- RIS Citation
IEEE Citation
IEEE Style Citation: N. Kanimozhi, S. Hari Ganesh, B. Karthikeyan, “An Analysis of Machine Learning Solution for QoS and QoE in Network (Infrastructure Oriented and Less),” International Journal of Computer Sciences and Engineering, Vol.11, Issue.5, pp.41-59, 2023.
MLA Citation
MLA Style Citation: N. Kanimozhi, S. Hari Ganesh, B. Karthikeyan "An Analysis of Machine Learning Solution for QoS and QoE in Network (Infrastructure Oriented and Less)." International Journal of Computer Sciences and Engineering 11.5 (2023): 41-59.
APA Citation
APA Style Citation: N. Kanimozhi, S. Hari Ganesh, B. Karthikeyan, (2023). An Analysis of Machine Learning Solution for QoS and QoE in Network (Infrastructure Oriented and Less). International Journal of Computer Sciences and Engineering, 11(5), 41-59.
BibTex Citation
BibTex Style Citation:
@article{Kanimozhi_2023,
author = {N. Kanimozhi, S. Hari Ganesh, B. Karthikeyan},
title = {An Analysis of Machine Learning Solution for QoS and QoE in Network (Infrastructure Oriented and Less)},
journal = {International Journal of Computer Sciences and Engineering},
issue_date = {5 2023},
volume = {11},
Issue = {5},
month = {5},
year = {2023},
issn = {2347-2693},
pages = {41-59},
url = {https://www.ijcseonline.org/full_paper_view.php?paper_id=5574},
doi = {https://doi.org/10.26438/ijcse/v11i5.4159}
publisher = {IJCSE, Indore, INDIA},
}
RIS Citation
RIS Style Citation:
TY - JOUR
DO = {https://doi.org/10.26438/ijcse/v11i5.4159}
UR - https://www.ijcseonline.org/full_paper_view.php?paper_id=5574
TI - An Analysis of Machine Learning Solution for QoS and QoE in Network (Infrastructure Oriented and Less)
T2 - International Journal of Computer Sciences and Engineering
AU - N. Kanimozhi, S. Hari Ganesh, B. Karthikeyan
PY - 2023
DA - 2023/05/31
PB - IJCSE, Indore, INDIA
SP - 41-59
IS - 5
VL - 11
SN - 2347-2693
ER -
![]() |
![]() |
![]() |
198 | 344 downloads | 151 downloads |
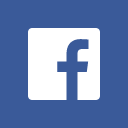
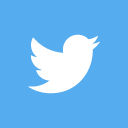
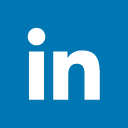
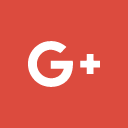
Abstract
Now, Communication network (Network may be a wired or wireless network. In wireless network it may be an infrastructure oriented or infrastructure less) plays vital role in the world. . At present without network people cannot do their work easily. Communication Network described as two or more device connecting together and share its resources. If a resource is accessed by more than one person. Network faces lot of issues in its Qualitative and Quantitative of Service. This paper is try to provide solution for infrastructure oriented and less network QoS (Quality of Service) and QoE (Quality of Experience) problems using AI and ML.
Key-Words / Index Term
Communication Network, Wireless Network, QoS, QoE, AI, ML
References
[1]. Raouf Boutaba, Mohammad A. Salahuddin, Noura Limam, Sara Ayoubi, Nashid Shahriar1, Felipe Estrada-Solano and Oscar M. Caicedo, “A comprehensive survey on machine learning for networking: evolution, applications and research opportunities”, Journal of Internet Services and Applications (2018) 9:16
[2]. Noman Haider, Zeeshan Baig andMuhammad Imran, “Artificial Intelligence and Machine Learning in 5G Network Security: Opportunities, advantages, and future research trends”, arXiv:2007.04490v1 [cs.CR] 9 Jul 2020
[3]. Rishabh Das, Thomas H. Morris, “Machine Learning and Cyber Security”, Conference Paper · December 2017, DOI: 10.1109/ICCECE.2017.8526232
[4]. R. Devakunchari, Sourabh, Prakhar Malik, “ A Study of Cyber Security using Machine Learning Techniques”, International Journal of Innovative Technology and Exploring Engineering (IJITEE) ISSN: 2278-3075,Volume-8, Issue-7C2, May 2019
[5]. D. Michie, D. J. Spiegelhalter, and C. Taylor, ‘‘Machine learning,’’ Neural Stat. Classification, vol. 13, 1994.
[6]. S. Dua and X. Du, Data Mining and Machine Learning in Cybersecurity. New York, NY, USA: Auerbach, 2016.
[7]. S. Angra and S. Ahuja, ‘‘Machine learning and its applications: A review,’’ in Proc. Int. Conf. Big Data Anal. Comput. Intell. (ICBDAC), 2017, pp. 57–60.
[8]. T. M. Alam, K. Shaukat, M. Mushtaq, Y. Ali, M. Khushi, S. Luo, and Wahab, ‘‘Corporate bankruptcy prediction: An approach towards bet- ter corporate world,’’ Comput. J., pp. 1–16, Jun. 2020.
[9]. G. Apruzzese, M. Colajanni, L. Ferretti, A. Guido, and M. Marchetti, ‘‘On the effectiveness of machine and deep learning for cyber security,’’ in Proc. 10th Int. Conf. Cyber Conflict (CyCon), May 2018, pp. 371–390.
[10]. B. Fraley and J. Cannady, ‘‘The promise of machine learning in cyber- security,’’ in Proc. SoutheastCon, Mar. 2017, pp. 1–6.
[11]. Kulkarni and L. L. Brown, III, ‘‘Phishing websites detection using machine learning,’’ Int. J. Adv. Comput. Sci. Appl., vol. 10, no. 7, pp. 8–13, 2019, doi: 10.14569/IJACSA.2019.0100702.
[12]. Islam and N. K. Chowdhury, ‘‘Phishing Websites detection using machine learning based classification techniques,’’ in Proc. 1st Int. Conf. Adv. Inf. Commun. Technol., 2016, pp. 1–4.
[13]. Buczak and E. Guven, ‘‘A survey of data mining and machine learning methods for cyber security intrusion detection,’’ IEEE Commun. Surveys Tuts., vol. 18, no. 2, pp. 1153–1176, 2nd Quart., 2016.
[14]. S. Marsland, Machine Learning: An Algorithmic Perspective. Boca Raton, FL, USA: CRC Press, 2014.
[15]. S. R. Granter, A. H. Beck, and D. J. Papke, ‘‘AlphaGo, deep learning, and the future of the human microscopist,’’ Arch. Pathol. Lab. Med., vol. 141, no. 5, pp. 619–621, May 2017.
[16]. J. X. Chen, ‘‘The evolution of computing: AlphaGo,’’ Comput. Sci. Eng., vol. 18, no. 4, pp. 4–7, Jul. 2016.
[17]. T. T. Nguyen and V. J. Reddi, ‘‘Deep reinforcement learning for cyber security,’’ 2019, arXiv:1906.05799. [Online]. Available: http://arxiv.org/abs/1906.05799
[18]. M. H. Ling, K.-L.-A. Yau, J. Qadir, G. S. Poh, and Q. Ni, ‘‘Application of reinforcement learning for security enhancement in cognitive radio networks,’’ Appl. Soft Comput., vol. 37, pp. 809–829, Dec. 2015.
[19]. Y. Wang, Z. Ye, P. Wan, and J. Zhao, ‘‘A survey of dynamic spectrum allocation based on reinforcement learning algorithms in cognitive radio networks,’’ Artif. Intell. Rev., vol. 51, no. 3, pp. 493–506, Mar. 2019.
[20]. W.-H. Chen, S.-H. Hsu, and H.-P. Shen, ‘‘Application of SVM and ANN for intrusion detection,’’ Comput. Oper. Res., vol. 32, no. 10, pp. 2617–2634, Oct. 2005.
[21]. J. R. Quinlan, C4. 5: Programs for Machine Learning. Amsterdam, The Netherlands: Elsevier, 2014.
[22]. H. Garcia, R. Monroy, and M. Quintana, ‘‘Web attack detection using ID3,’’ in Proc. IFIP World Comput. Congr. (TC), vol. 12. Boston, MA, USA: Springer, 2006, pp. 323–332.
[23]. WEKA Packages. Accessed: Nov. 16, 2020. [Online]. Available: https://weka.sourceforge.io/packageMetaData/
[24]. V. Chandola, A. Banerjee, and V. Kumar, ‘‘Anomaly detection: A survey,’’ ACM Comput. Surv., vol. 41, no. 3, p. 15, 2009.
[25]. Aburomman and M. B. Ibne Reaz, ‘‘A novel SVM-kNN-PSO ensemble method for intrusion detection system,’’ Appl. Soft Comput., vol. 38, pp. 360–372, Jan. 2016.
[26]. S. He, G. M. Lee, S. Han, and A. B. Whinston, ‘‘How would information disclosure influence organizations’ outbound spam volume? Evidence from a field experiment,’’ J. Cybersecurity, vol. 2, no. 1, pp. 99–118, Dec. 2016.
[27]. S. T. Miller and C. Busby-Earle, ‘‘Multi-perspective machine learning a classifier ensemble method for intrusion detection,’’ in Proc. Int. Conf. Mach. Learn. Soft Comput. (ICMLSC), 2017, pp. 7–12.
[28]. V. Narayan and D. Shanmugapriya, ‘‘Big data analytics with machine learning and deep learning methods for detection of anomalies in net- work traffic,’’ in Handbook of Research on Machine and Deep Learning Applications for Cyber Security. Hershey, PA, USA: IGI Global, 2020, pp. 317–346.
[29]. L. Jiang, H. Zhang, and Z. Cai, ‘‘A novel Bayes model: Hidden naive Bayes,’’ IEEE Trans. Knowl. Data Eng., vol. 21, no. 10, pp. 1361–1371, Oct. 2009.
[30]. M. Kibriya, E. Frank, B. Pfahringer, and G. Holmes, ‘‘Multinomial naive Bayes for text categorization revisited,’’ in Proc. Australas. Joint Conf. Artif. Intell. Berlin, Germany: Springer, 2004, pp. 488–499.
[31]. McCallum and K. Nigam, ‘‘A comparison of event models for naive bayes text classification,’’ in Proc. Workshop Learn. Text Categorization (AAAI), vol. 752, no. 1. Madison, WI, USA: Citeseer, 1998, pp. 41–48. [Online]. Available: http://www.cs.cmu.edu/~mccallum/textcat.html
[32]. G. H. John and P. Langley, ‘‘Estimating continuous distributions in Bayesian classifiers,’’ in Proc. 11th Conf. Uncertainty Artif. Intell. San Mateo, CA, USA: Morgan Kaufmann, 1995, pp. 338–345.
[33]. Jagota, ‘‘Novelty detection on a very large number of memories stored in a hopfield-style network,’’ in Proc. Seattle Int. Joint Conf. Neural Netw. (IJCNN), vol. 2, 1991, p. 905.
[34]. P. Taveras and L. Hernandez, ‘‘Supervised machine learning techniques, cybersecurity habits and human generated password entropy for hacking prediction,’’ in Proc. MWAIS, vol. 38, 2018, pp. 1–6. [Online]. Available: http://aisel.aisnet.org/mwais2018/38
[35]. S. Sathasivam and W. A. T. W. Abdullah, ‘‘Logic learning in hopfield networks,’’ 2008, arXiv:0804.4075. [Online]. Available: http://arxiv.org/abs/0804.4075
[36]. J. M. Gómez Hidalgo, G. C. Bringas, E. P. Sánz, and F. C. García, ‘‘Content based SMS spam filtering,’’ in Proc. ACM Symp. Document Eng. (DocEng), 2006, pp. 107–114.
[37]. K. Fukushima, ‘‘Neocognitron: A hierarchical neural network capable of visual pattern recognition,’’ Neural Netw., vol. 1, no. 2, pp. 119–130, Jan. 1988.
[38]. M. D. Zeiler and R. Fergus, ‘‘Visualizing and understanding convolu- tional networks,’’ in Proc. Eur. Conf. Comput. Vis. Cham, Switzerland: Springer, 2014, pp. 818–833.
[39]. Szegedy, W. Liu, Y. Jia, P. Sermanet, S. Reed, D. Anguelov, D. Erhan, V. Vanhoucke, and A. Rabinovich, ‘‘Going deeper with convolutions,’’ in Proc. IEEE Conf. Comput. Vis. Pattern Recognit. (CVPR), Jun. 2015, pp. 1–9.
[40]. K. He, X. Zhang, S. Ren, and J. Sun, ‘‘Deep residual learning for image recognition,’’ in Proc. IEEE Conf. Comput. Vis. Pattern Recognit. (CVPR), Jun. 2016, pp. 770–778.
[41]. S. Lawrence, C. L. Giles, A. Chung Tsoi, and A. D. Back, ‘‘Face recog- nition: A convolutional neural-network approach,’’ IEEE Trans. Neural Netw., vol. 8, no. 1, pp. 98–113, Jan. 1997.
[42]. Wallach, M. Dzamba, and A. Heifets, ‘‘AtomNet: A deep con- volutional neural network for bioactivity prediction in structure- based drug discovery,’’ 2015, arXiv:1510.02855. [Online]. Available: http://arxiv.org/abs/1510.02855
[43]. H. Ren, B. Xu, Y. Wang, C. Yi, C. Huang, X. Kou, T. Xing, M. Yang, J. Tong, and Q. Zhang, ‘‘Time-series anomaly detection service at microsoft,’’ in Proc. 25th ACM SIGKDD Int. Conf. Knowl. Discovery Data Mining, Jul. 2019, pp. 3009–3017.
[44]. R. Vinayakumar, K. P. Soman, and P. Poornachandran, ‘‘Applying con- volutional neural network for network intrusion detection,’’ in Proc. Int. Conf. Adv. Comput., Commun. Informat. (ICACCI), Sep. 2017, pp. 1222–1228.
[45]. R. U. Khan, X. Zhang, M. Alazab, and R. Kumar, ‘‘An improved convolutional neural network model for intrusion detection in net- works,’’ in Proc. Cybersecurity Cyberforensics Conf. (CCC), May 2019, pp. 74–77.
[46]. K. Millar, A. Cheng, H. G. Chew, and C.-C. Lim, ‘‘Using convolu- tional neural networks for classifying malicious network traffic,’’ in Deep Learning Applications for Cyber Security. Cham, Switzerland: Springer, 2019, pp. 103–126.
[47]. W. Wang, M. Zhu, X. Zeng, X. Ye, and Y. Sheng, ‘‘Malware traffic classi- fication using convolutional neural network for representation learning,’’ in Proc. Int. Conf. Inf. Netw. (ICOIN), 2017, pp. 712–717.
[48]. W. Wang, M. Zhu, J. Wang, X. Zeng, and Z. Yang, ‘‘End-to-end encrypted traffic classification with one-dimensional convolution neu- ral networks,’’ in Proc. IEEE Int. Conf. Intell. Secur. Informat. (ISI), Jul. 2017, pp. 43–48.
[49]. Y.-J. Zheng, W.-G. Sheng, X.-M. Sun, and S.-Y. Chen, ‘‘Airline passenger profiling based on fuzzy deep machine learning,’’ IEEE Trans. Neural Netw. Learn. Syst., vol. 28, no. 12, pp. 2911–2923, Dec. 2017.
[50]. F. He, Y. Zhang, D. Liu, Y. Dong, C. Liu, and C. Wu, ‘‘Mixed wavelet- based neural network model for cyber security situation prediction using MODWT and Hurst exponent analysis,’’ in Proc. Int. Conf. Netw. Syst. Secur. Cham, Switzerland: Springer, 2017, pp. 99–111.
[51]. J. C. Burges, ‘‘A tutorial on support vector machines for pattern recognition,’’ Data Mining Knowl. Discovery, vol. 2, no. 2, pp. 121–167, 1998
[52]. Frank and M. A. Hall, Data Mining: Practical Machine Learning Tools and Techniques. San Mateo, CA, USA: Morgan Kaufmann, 2011.
[53]. R. Agrawal and R. Srikant, ‘‘Mining sequential patterns,’’ in Proc. 11th Int. Conf. Data Eng., 1995, pp. 3–14.
[54]. A. K. Jain, J. Mao, and K. M. Mohiuddin, ‘‘Artificial neural networks: A tutorial,’’ Computer, vol. 29, no. 3, pp. 31–44, Mar. 1996.
[55]. Q. J. Ross, C4. 5: Programs for Machine Learning. San Mateo, CA, USA: Morgan Kaufmann, 1993.
[56]. K. Jain and R. C. Dubes, Algorithms for Clustering Data. Upper Saddle River, NJ, USA: Prentice-Hall, 1988.
[57]. Q. Tian, D. Han, K.-C. Li, X. Liu, L. Duan, and A. Castiglione, ‘‘An intru- sion detection approach based on improved deep belief network,’’ Int. J. Speech Technol., vol. 50, no. 10, pp. 3162–3178, Oct. 2020.
[58]. K. He and J. Sun, ‘‘Convolutional neural networks at constrained time cost,’’ in Proc. IEEE Conf. Comput. Vis. Pattern Recognit. (CVPR), Jun. 2015, pp. 5353–5360.
[59]. Tzortzis and A. Likas, ‘‘Deep belief networks for spam filtering,’’ in Proc. 19th IEEE Int. Conf. Tools Artif. Intell. (ICTAI), vol. 2, Oct. 2007, pp. 306–309.
[60]. D. Bhamare, T. Salman, M. Samaka, A. Erbad, and R. Jain, ‘‘Feasibility of supervised machine learning for cloud security,’’ in Proc. Int. Conf. Inf. Sci. Secur. (ICISS), Dec. 2016, pp. 1–5.
[61]. P. Sangkatsanee, N. Wattanapongsakorn, and C. Charnsripinyo, ‘‘Prac- tical real-time intrusion detection using machine learning approaches,’’ Comput. Commun., vol. 34, no. 18, pp. 2227–2235, Dec. 2011.
[62]. Amiri, M. R. Yousefi, C. Lucas, A. Shakery, and N. Yazdani, ‘‘Mutual information-based feature selection for intrusion detection systems,’’ J. Netw. Comput. Appl., vol. 34, no. 4, pp. 1184–1199, Jul. 2011.
[63]. S.-W. Lin, K.-C. Ying, C.-Y. Lee, and Z.-J. Lee, ‘‘An intelligent algorithm with feature selection and decision rules applied to anomaly intrusion detection,’’ Appl. Soft Comput., vol. 12, no. 10, pp. 3285–3290, Oct. 2012.
[64]. S. Elhag, A. Fernández, A. Bawakid, S. Alshomrani, and F. Herrera, ‘‘On the combination of genetic fuzzy systems and pairwise learning for improving detection rates on intrusion detection systems,’’ Expert Syst. Appl., vol. 42, no. 1, pp. 193–202, Jan. 2015.
[65]. Gharaee and H. Hosseinvand, ‘‘A new feature selection IDS based on genetic algorithm and SVM,’’ in Proc. 8th Int. Symp. Telecommun. (IST), Sep. 2016, pp. 139–144.
[66]. D. Boughaci, M. D. E. Kadi, and M. Kada, ‘‘Fuzzy particle swarm opti- mization for intrusion detection,’’ in Proc. Int. Conf. Neural Inf. Process. Berlin, Germany: Springer, 2012, pp. 541–548.
[67]. P. Casas, J. Mazel, and P. Owezarski, ‘‘Unsupervised network intrusion detection systems: Detecting the unknown without knowledge,’’ Comput. Commun., vol. 35, no. 7, pp. 772–783, Apr. 2012.
[68]. W.-C. Lin, S.-W. Ke, and C.-F. Tsai, ‘‘CANN: An intrusion detection sys- tem based on combining cluster centers and nearest neighbors,’’ Knowl.- Based Syst., vol. 78, pp. 13–21, Apr. 2015.
[69]. T. Lane and C. E. Brodley, ‘‘An application of machine learning to anomaly detection,’’ in Proc. 20th Nat. Inf. Syst. Secur. Conf., Baltimore, MD, USA, vol. 377, 1997, pp. 366–380.
[70]. L. Khan, M. Awad, and B. Thuraisingham, ‘‘A new intrusion detection system using support vector machines and hierarchical clustering,’’ VLDB J., vol. 16, no. 4, pp. 507–521, Aug. 2007.
[71]. G. Wang, J. Hao, J. Ma, and L. Huang, ‘‘A new approach to intrusion detection using artificial neural networks and fuzzy clustering,’’ Expert Syst. Appl., vol. 37, no. 9, pp. 6225–6232, Sep. 2010.
[72]. S.-J. Horng, M.-Y. Su, Y.-H. Chen, T.-W. Kao, R.-J. Chen, J.-L. Lai, and C. D. Perkasa, ‘‘A novel intrusion detection system based on hierarchical clustering and support vector machines,’’ Expert Syst. Appl., vol. 38, no. 1, pp. 306–313, 2011.
[73]. M. Chandrasekhar and K. Raghuveer, ‘‘Intrusion detection technique by using k-means, fuzzy neural network and SVM classifiers,’’ in Proc. Int. Conf. Comput. Commun. Informat., Jan. 2013, pp. 1–7.
[74]. G. Kim, S. Lee, and S. Kim, ‘‘A novel hybrid intrusion detection method integrating anomaly detection with misuse detection,’’ Expert Syst. Appl., vol. 41, no. 4, pp. 1690–1700, Mar. 2014.
[75]. E. Hodo, X. Bellekens, A. Hamilton, C. Tachtatzis, and R. Atkinson, ‘‘Shallow and deep networks intrusion detection system: A tax- onomy and survey,’’ 2017, arXiv:1701.02145. [Online]. Available: http://arxiv.org/abs/1701.02145
[76]. M. H. Bhuyan, D. K. Bhattacharyya, and J. K. Kalita, ‘‘Network anomaly detection: Methods, systems and tools,’’ IEEE Commun. Surveys Tuts., vol. 16, no. 1, pp. 303–336, 1st Quart., 2014.
[77]. P. Mishra, V. Varadharajan, U. Tupakula, and E. S. Pilli, ‘‘A detailed inves- tigation and analysis of using machine learning techniques for intrusion detection,’’ IEEE Commun. Surveys Tuts., vol. 21, no. 1, pp. 686–728, 1st Quart., 2019.
[78]. M. D. Rich, ‘‘Evaluating machine learning classifiers for hybrid network intrusion detection systems,’’ M.S. thesis, Wright-Patterson AFB OH Graduate School Eng. Manage., Air Force Inst. Technol., Wright-Patterson AFB, OH, USA, 2015.
[79]. Akhi, E. J. Kanon, A. Kabir, and A. Banu, ‘‘Network intrusion classification employing machine learning: A survey,’’ Ph.D. dissertation, Dept. Comput. Sci. Eng., United Int. Univ., Dhaka, Bangladesh, 2019.
[80]. R. Jamadar, S. Ingale, A. Panhalkar, A. Kakade, and M. Shinde, ‘‘Survey of deep learning based intrusion detection systems for cyber security,’’ Int. J. Res. Anal. Rev., vol. 6, no. 2, pp. 257–261, 2019.
[81]. R. Vinayakumar, M. Alazab, K. P. Soman, P. Poornachandran, Al-Nemrat, and S. Venkatraman, ‘‘Deep learning approach for intelli- gent intrusion detection system,’’ IEEE Access, vol. 7, pp. 41525–41550, 2019.
[82]. M. Almseidin, M. Alzubi, S. Kovacs, and M. Alkasassbeh, ‘‘Evalua- tion of machine learning algorithms for intrusion detection system,’’ in Proc. IEEE 15th Int. Symp. Intell. Syst. Informat. (SISY), Sep. 2017, pp. 000277–000282.
[83]. R. Chalapathy and S. Chawla, ‘‘Deep learning for anomaly detection: A survey,’’ 2019, arXiv:1901.03407. [Online]. Available: http://arxiv.org/abs/1901.03407
[84]. Torkaman, G. Javadzadeh, and M. Bahrololum, ‘‘A hybrid intelligent HIDS model using two-layer genetic algorithm and neural network,’’ in Proc. 5th Conf. Inf. Knowl. Technol., May 2013, pp. 92–96.
[85]. R. Puzis, M. D. Klippel, Y. Elovici, and S. Dolev, ‘‘Optimization of NIDS placement for protection of intercommunicating critical infrastructures,’’ in Proc. Eur. Conf. Intell. Secur. Inform. Berlin, Germany: Springer, 2008, pp. 191–203.
[86]. N. B. Amor, S. Benferhat, and Z. Elouedi, ‘‘Naive bayes vs decision trees in intrusion detection systems,’’ in Proc. ACM Symp. Appl. Comput. (SAC), 2004, pp. 420–424.
[87]. Y. Bouzida and F. Cuppens, ‘‘Neural networks vs. decision trees for intru- sion detection,’’ in Proc. IEEE/IST Workshop Monitor., Attack Detection Mitigation (MonAM), vol. 28. Citeseer, 2006, p. 29
[88]. S. Kim and J. S. Park, ‘‘Network-based intrusion detection with support vector machines,’’ in Proc. Int. Conf. Inf. Netw. Berlin, Germany: Springer, 2003, pp. 747–756.
[89]. Tajbakhsh, M. Rahmati, and A. Mirzaei, ‘‘Intrusion detection using fuzzy association rules,’’ Appl. Soft Comput., vol. 9, no. 2, pp. 462–469, Mar. 2009.
[90]. W. Lee, S. J. Stolfo, and K. W. Mok, ‘‘A data mining framework for building intrusion detection models,’’ in Proc. IEEE Symp. Secur. Privacy, May 1999, pp. 120–132.
[91]. K. Ghosh and A. Schwartzbard, ‘‘A study in using neural networks for anomaly and misuse detection,’’ in Proc. USENIX Secur. Symp., vol. 99, 1999, p. 12.
[92]. Cannady, ‘‘Artificial neural networks for misuse detection,’’ in Proc. Nat. Inf. Syst. Secur. Conf., Baltimore, MD, USA, vol. 26, 1998, pp. 443–456.
[93]. S. Chebrolu, A. Abraham, and J. P. Thomas, ‘‘Feature deduction and ensemble design of intrusion detection systems,’’ Comput. Secur., vol. 24, no. 4, pp. 295–307, Jun. 2005.
[94]. M. G. Schultz, E. Eskin, F. Zadok, and S. J. Stolfo, ‘‘Data mining methods for detection of new malicious executables,’’ in Proc. IEEE Symp. Secur. Privacy. (S P), May 2000, pp. 38–49.
[95]. L. Feinstein, D. Schnackenberg, R. Balupari, and D. Kindred, ‘‘Statistical approaches to DDoS attack detection and response,’’ in Proc. DARPA Inf. Survivability Conf. Exposit., vol. 1, 2003, pp. 303–314.
[96]. M.-Y. Su, G.-J. Yu, and C.-Y. Lin, ‘‘A real-time network intrusion detection system for large-scale attacks based on an incremental mining approach,’’ Comput. Secur., vol. 28, no. 5, pp. 301–309, Jul. 2009.
[97]. Y. Liao and V. R. Vemuri, ‘‘Use of K-Nearest neighbor classifier for intrusion detection,’’ Comput. Secur., vol. 21, no. 5, pp. 439–448, Oct. 2002.
[98]. Endler, ‘‘Applying machine learning to Solaris audit data,’’ in Proc. Annu. Comput. Secur. Appl. Conf., 1998, pp. 268–279.
[99]. W. Lee and S. J. Stolfo, ‘‘A framework for constructing features and models for intrusion detection systems,’’ ACM Trans. Inf. Syst. Secur., vol. 3, no. 4, pp. 227–261, Nov. 2000.
[100]. C. Kruegel and T. Toth, ‘‘Using decision trees to improve signature-based intrusion detection,’’ in Proc. Int. Workshop Recent Adv. Intrusion Detection. Berlin, Germany: Springer, 2003, pp. 173–191.
[101]. Abraham, C. Grosan, and C. Martin-Vide, ‘‘Evolutionary design of intrusion detection programs,’’ IJ Netw. Secur., vol. 4, no. 3, pp. 328–339, 2007.
[102]. Abraham, R. Jain, J. Thomas, and S. Y. Han, ‘‘D-SCIDS: Distributed soft computing intrusion detection system,’’ J. Netw. Comput. Appl., vol. 30, no. 1, pp. 81–98, Jan. 2007.
[103]. S. Mukkamala and A. H. Sung, ‘‘A comparative study of techniques for intrusion detection,’’ in Proc. 15th IEEE Int. Conf. Tools Artif. Intell., Nov. 2003, pp. 570–577.
[104]. R. Mohan, V. Vaidehi, M. Mahalakshmi, and S. S. Chakkaravarthy, ‘‘Complex event processing based hybrid intrusion detection system,’’ in Proc. 3rd Int. Conf. Signal Process., Commun. Netw. (ICSCN), Mar. 2015, pp. 1–6.
[105]. S. H. Vasudeo, P. Patil, and R. V. Kumar, ‘‘IMMIX-intrusion detec- tion and prevention system,’’ in Proc. Int. Conf. Smart Technol. Man- age. Comput., Commun., Controls, Energy Mater. (ICSTM), 2015, pp. 96–101
[106]. K. Ghosh, A. Schwartzbard, and M. Schatz, ‘‘Learning program behavior profiles for intrusion detection,’’ in Proc. Workshop Intrusion Detection Netw. Monitor., vol. 51462, 1999, pp. 1–13.
[107]. Zhang and M. Zulkernine, ‘‘A hybrid network intrusion detection technique using random forests,’’ in Proc. 1st Int. Conf. Availability, Rel. Secur. (ARES), 2006, p. 269.
[108]. P. García-Teodoro, J. Díaz-Verdejo, G. Maciá-Fernández, and E. Vázquez, ‘‘Anomaly-based network intrusion detection: Techniques, systems and challenges,’’ Comput. Secur., vol. 28, nos. 1–2, pp. 18–28, Feb. 2009.
[109]. T. T. T. Nguyen and G. Armitage, ‘‘A survey of techniques for Internet traffic classification using machine learning,’’ IEEE Commun. Surveys Tuts., vol. 10, no. 4, pp. 56–76, 4th Quart., 2008.
[110]. S. X. Wu and W. Banzhaf, ‘‘The use of computational intelligence in intrusion detection systems: A review,’’ Appl. Soft Comput., vol. 10, no. 1, pp. 1–35, Jan. 2010.
[111]. K.-D. Althoff, R. Bergmann, S. Wess, M. Manago, E. Auriol, O. I. Larichev, A. Bolotov, Y. I. Zhuravlev, and S. I. Gurov, ‘‘Case-based reasoning for medical decision support tasks: The inreca approach,’’ Artif. Intell. Med., vol. 12, no. 1, pp. 25–41, Jan. 1998.
[112]. M. Esmaili, B. Balachandran, R. Safavi-Naini, and J. Pieprzyk, ‘‘Case- based reasoning for intrusion detection,’’ in Proc. 12th Annu. Comput. Secur. Appl. Conf., 1996, pp. 214–223.
[113]. J. Yang, T. Deng, and R. Sui, ‘‘An adaptive weighted one-class svm for robust outlier detection,’’ in Proc. Chin. Intell. Syst. Conf. Berlin, Germany: Springer, 2016, pp. 475–484.
[114]. G. Kumar and K. Kumar, ‘‘A multi-objective genetic algorithm based approach for effective intrusion detection using neural networks,’’ in Intelligent Methods for Cyber Warfare. Cham, Switzerland: Springer, 2015, pp. 173–200.
[115]. Sezari, D. P. F. Moller, and A. Deutschmann, ‘‘Anomaly-based network intrusion detection model using deep learning in airports,’’ in Proc. 17th IEEE Int. Conf. Trust, Secur. Privacy Comput. Commun., 12th IEEE Int. Conf. Big Data Sci. Eng. (TrustCom/BigDataSE), Aug. 2018, pp. 1725–1729.
[116]. M. Aljanabi, M. A. Ismail, and V. Mezhuyev, ‘‘Improved TLBO-JAYA algorithm for subset feature selection and parameter optimisation in intrusion detection system,’’ Complexity, vol. 2020, pp. 1–18, May 2020.
[117]. McAfee Network Security Platform. Accessed: Feb. 16, 2020. [Online]. Available: https://www.mcafee.com/enterprise/en-au/products/network- security-platform.html
[118]. Hillstone S-Series Network Intrusion Prevention System (NIPS). Accessed: Feb. 16, 2020. [Online]. Available: https:// www.hillstonenet.com/products/network-intrusion-prevention-system-s- series/
[119]. NIP2000/5000 Intrusion Prevention System. Accessed: Feb. 16, 2020. [Online]. Available: https://e.huawei.com/en/related-page/products/ enterprise-network/security/application-gateway/nip-ips/security_ nip2000_5000_ips_v2_en
[120]. Palo Alto Networks Completes Acquisition of The Crypsis Group. Accessed: Oct. 10, 2020. [Online]. Available: https:// www.paloaltonetworks.com/
[121]. Dark Trace—Cyber AI Security. Accessed: Oct. 10, 2020. [Online]. Avail- able: https://www.darktrace.com/en/
[122]. Next-Generation Intrusion Prevention System (NGIPS). Accessed: Feb. 14, 2020. [Online]. Available: https://www. cisco.com/c/en_au/products/security/ngips/index.html
[123]. Snort 2.9.15.1. Accessed: Feb. 16, 2020. [Online]. Available: https://www.snort.org/
[124]. Suricata | Open Source IDS / IPS / NSM Engine. Accessed: Feb. 16, 2020. [Online]. Available: https://suricata-ids.org/
[125]. The SAMHAIN File Integrity / Host-Based Intrusion Detection System. Accessed: Feb. 16, 2020. [Online]. Available: https://la- samhna.de/samhain/
[126]. Security Onion. Accessed: Feb. 16, 2020. [Online]. Available: https://securityonion.net/
[127]. THE SAGAN LOG ANALYSIS ENGINE. Accessed: Feb. 16, 2020. [Online]. Available: https://quadrantsec.com/sagan_log_analysis_ engine/
[128]. What Are STIX/TAXII. Accessed: Nov. 16, 2020. [Online]. Available: https://www.anomali.com/resources/what-are-stix-taxii
[129]. Sun, Z. Chen, R. Wang, F. Yu, and V. C. M. Leung, ‘‘Towards adap- tive anomaly detection in cellular mobile networks,’’ in Proc. 3rd IEEE Consum. Commun. Netw. Conf. (CCNC), vol. 2, Jan. 2006, pp. 666–670.
[130]. Sun, Y. Xiao, and K. Wu, ‘‘Intrusion detection in cellular mobile networks,’’ in Wireless Network Security. Boston, MA, USA: Springer, 2007, pp. 183–210.
[131]. V. Richariya, U. P. Singh, and R. Mishra, ‘‘Distributed approach of intrusion detection system: Survey,’’ Int. J. Adv. Comput. Res., vol. 2, no. 4, p. 358, 2012.
[132]. Wu, J. Chen, J. Wu, and M. Cardei, ‘‘A survey of attacks and coun- termeasures in mobile ad hoc networks,’’ in Wireless Network Security. Boston, MA, USA: Springer, 2007, pp. 103–135.
[133]. Maimo, A. L. P. Gomez, F. J. G. Clemente, M. G. Perez, and G. M. Perez, ‘‘A self-adaptive deep learning-based system for anomaly detection in 5G networks,’’ IEEE Access, vol. 6, pp. 7700–7712, 2018.
[134]. Korba, M. Nafaa, and G. Salim, ‘‘Survey of routing attacks and countermeasures in mobile ad hoc networks,’’ in Proc. 15th Int. Conf. Comput. Modeling Simulation (UKSim), Apr. 2013, pp. 693–698.
[135]. R. Buschkes, D. Kesdogan, and P. Reichl, ‘‘How to increase security in mobile networks by anomaly detection,’’ in Proc. 14th Annu. Comput. Secur. Appl. Conf., 1998, pp. 3–12.
[136]. Y. Moreau, H. Verrelst, and J. Vandewalle, ‘‘Detection of mobile phone fraud using supervised neural networks: A first prototype,’’ in Proc. Int. Conf. Artif. Neural Netw. Berlin, Germany: Springer, 1997, pp. 1065–1070.
[137]. J. Hollmén, User Profiling and Classification For Fraud Detection in Mobile Communications Networks. Espoo, Finland: Helsinki Univ. of Technology, 2000.
[138]. P. Burge and J. Shawe-Taylor, ‘‘An unsupervised neural network approach to profiling the behavior of mobile phone users for se in fraud detection,’’ J. Parallel Distrib. Comput., vol. 61, no. 7, pp. 915–925, Jul. 2001.
[139]. Boukerche and M. S. M. A. Notare, ‘‘Behavior-based intrusion detec- tion in mobile phone systems,’’ J. Parallel Distrib. Comput., vol. 62, no. 9, pp. 1476–1490, Sep. 2002.
[140]. Liu, G. Yan, X. Zhang, and S. Chen, ‘‘Virusmeter: Preventing your cellphone from spies,’’ in Proc. Int. Workshop Recent Adv. Intrusion Detection. Berlin, Germany: Springer, 2009, pp. 244–264.
[141]. P. Kumpulainen and K. Hätönen, ‘‘Anomaly detection algorithm test bench for mobile network management,’’ Tampere Univ. Technol., Tampere, Finland, 2008. [Online]. Available: http://citeseerx.ist.psu.edu/ viewdoc/download?doi=10.1.1.618.5065&rep=rep1&type=pdf
[142]. Damopoulos, S. A. Menesidou, G. Kambourakis, M. Papadaki, N. Clarke, and S. Gritzalis, ‘‘Evaluation of anomaly-based IDS for mobile devices using machine learning classifiers,’’ Secur. Commun. Netw., vol. 5, no. 1, pp. 3–14, Jan. 2012.
[143]. Feizollah, N. B. Anuar, R. Salleh, F. Amalina, and S. Shamshirband, ‘‘A study of machine learning classifiers for anomaly-based mobile bot- net detection,’’ Malaysian J. Comput. Sci., vol. 26, no. 4, pp. 251–265, Dec. 2013.
[144]. Shams and A. Rizaner, ‘‘A novel support vector machine based intrusion detection system for mobile ad hoc networks,’’ Wireless Netw., vol. 24, no. 5, pp. 1821–1829, Jul. 2018.
[145]. K. K. Nguyen, D. T. Hoang, D. Niyato, P. Wang, D. Nguyen, and E. Dutkiewicz, ‘‘Cyberattack detection in mobile cloud computing: A deep learning approach,’’ in Proc. IEEE Wireless Commun. Netw. Conf. (WCNC), Apr. 2018, pp. 1–6.
[146]. Bitdefender. Accessed: Feb. 16, 2020. [Online]. Available: https://www.bitdefender.com.au/
[147]. Trend Micro. Accessed: Feb. 16, 2020. [Online]. Available: https://www.trendmicro.com/en_au/forHome.html
[148]. BullGuard. Accessed: Feb. 16, 2020. [Online]. Available: https:// www.bullguard.com/
[149]. Sophos. Accessed: Feb. 16, 2020. [Online]. Available: https://www. sophos.com/en-us.aspx
[150]. Trustlook. Accessed: Feb. 16, 2020. [Online]. Available: https://www. trustlook.com/
[151]. Psafe Electronic Security Systems. Accessed: Feb. 16, 2020. [Online]. Available: http://www.esuppliersindia.com/psafe-electronic-security- systems/intrusion-alarm-system-pr3537476-sFP-swf.html
[152]. P. Pathak, ‘‘Cybercrime: A global threat to cybercommunity,’’ Int. J. Comput. Sci. Eng. Technol., vol. 7, no. 3, pp. 46–49, 2016.
[153]. H. Guo, H. K. Cheng, and K. Kelley, ‘‘Impact of network structure on malware propagation: A growth curve perspective,’’ J. Manage. Inf. Syst., vol. 33, no. 1, pp. 296–325, Jan. 2016.
[154]. R. Reports. McAfee Labs Threats Report August 2019. Accessed: Nov. 15, 2020. [Online]. Available: https://www.mcafee.com/enterprise/ en-us/assets/reports/rp-quarterly-threats-aug-2019.pdf
[155]. The 2012 Norton Cybercrime Report, Symantec, Mountain View, CA, USA, 2012.
[156]. Malware Report: The Economic Impact of Viruses, Spyware, Adware, Botnets, and Other Malicious Code, Comput. Econ., Irvine, CA, USA, 2007.
[157]. R. Stone, ‘‘A call to cyber arms,’’ Amer. Assoc. Advancement Sci., Wash- ington, DC, USA, Tech. Rep., 2013. [Online]. Available: https://science. sciencemag.org/content/339/6123/1026/tab-pdf, doi: 10.1126/science. 339.6123.1026.
[158]. R. Richardson, ‘‘CSI computer crime and security survey,’’ Comput. Secur. Inst., vol. 1, pp. 1–30, Nov. 2008.
[159]. Gandotra, D. Bansal, and S. Sofat, ‘‘Malware analysis and classifica- tion: A survey,’’ J. Inf. Secur., vol. 5, no. 2, p. 56, 2014.
[160]. K. Rieck, P. Trinius, C. Willems, and T. Holz, ‘‘Automatic analysis of malware behavior using machine learning,’’ J. Comput. Secur., vol. 19, no. 4, pp. 639–668, Jun. 2011.
[161]. K. Allix, T. F. Bissyandé, Q. Jérome, J. Klein, R. State, and Y. L. Traon, ‘‘Large-scale machine learning-based malware detection: Confronting the ‘10-fold cross validation’ scheme with reality,’’ in Proc. 4th ACM Conf. Data Appl. Secur. Privacy (CODASPY), 2014, pp. 163–166.
[162]. V. Nath and B. M. Mehtre, ‘‘Static malware analysis using machine learning methods,’’ in Proc. Int. Conf. Secur. Comput. Netw. Distrib. Syst. Berlin, Germany: Springer, 2014, pp. 440–450.
[163]. X. Sun, J. Dai, P. Liu, A. Singhal, and J. Yen, ‘‘Using Bayesian networks for probabilistic identification of zero-day attack paths,’’ IEEE Trans. Inf. Forensics Security, vol. 13, no. 10, pp. 2506–2521, Oct. 2018.
[164]. Y. Afek, A. Bremler-Barr, and S. L. Feibish, ‘‘Zero-day signature extrac- tion for high-volume attacks,’’ IEEE/ACM Trans. Netw., vol. 27, no. 2, pp. 691–706, Apr. 2019.
[165]. Govindaraju, ‘‘Exhaustive statistical analysis for detection of meta- morphic malware,’’ Master’s Projects, 2010, p. 66. [Online]. Available: https://scholarworks.sjsu.edu/etd_projects/66/, doi: 10.31979/etd.ucv9- qd8t.
[166]. Sharma and S. K. Sahay, ‘‘Evolution and detection of polymorphic and metamorphic malwares: A survey,’’ 2014, arXiv:1406.7061. [Online]. Available: http://arxiv.org/abs/1406.7061
[167]. Santos, F. Brezo, X. Ugarte-Pedrero, and P. G. Bringas, ‘‘Opcode sequences as representation of executables for data-mining-based unknown malware detection,’’ Inf. Sci., vol. 231, pp. 64–82, May 2013.
[168]. Henchiri and N. Japkowicz, ‘‘A feature selection and evaluation scheme for computer virus detection,’’ in Proc. 6th Int. Conf. Data Mining (ICDM), Dec. 2006, pp. 891–895.
[169]. M. Siddiqui, M. C. Wang, and J. Lee, ‘‘Detecting Internet worms using data mining techniques,’’ J. Systemics, Cybern. Inform., vol. 6, no. 6, pp. 48–53, 2009.
[170]. S. B. Mehdi, A. K. Tanwani, and M. Farooq, ‘‘IMAD: In-execution malware analysis and detection,’’ in Proc. 11th Annu. Conf. Genetic Evol. Comput. (GECCO), 2009, pp. 1553–1560.
[171]. Z. Kolter and M. A. Maloof, ‘‘Learning to detect malicious executables in the wild,’’ in Proc. ACM SIGKDD Int. Conf. Knowl. Discovery Data Mining (KDD), 2004, pp. 470–478.
[172]. S. M. Tabish, M. Z. Shafiq, and M. Farooq, ‘‘Malware detection using statistical analysis of byte-level file content,’’ in Proc. ACM SIGKDD Workshop CyberSecurity Intell. Informat. (CSI-KDD), 2009, pp. 23–31.
[173]. Mehdi, F. Ahmed, S. A. Khayyam, and M. Farooq, ‘‘Towards a the- ory of generalizing system call representation for in-execution malware detection,’’ in Proc. IEEE Int. Conf. Commun., May 2010, pp. 1–5.
[174]. Santos, J. Nieves, and P. G. Bringas, ‘‘Semi-supervised learning for unknown malware detection,’’ in Proc. Int. Symp. Distrib. Comput. Artif. Intell. Springer, 2011, pp. 415–422.
[175]. David and N. S. Netanyahu, ‘‘DeepSign: Deep learning for auto- matic malware signature generation and classification,’’ in Proc. Int. Joint Conf. Neural Netw. (IJCNN), Jul. 2015, pp. 1–8.
[176]. M. Yeo, Y. Koo, Y. Yoon, T. Hwang, J. Ryu, J. Song, and C. Park, ‘‘Flow- based malware detection using convolutional neural network,’’ in Proc. Int. Conf. Inf. Netw. (ICOIN), Jan. 2018, pp. 910–913.
[177]. A. Sharma and S. K. Sahay, ‘‘An effective approach for classification of advanced malware with high accuracy,’’ 2016, arXiv:1606.06897. [Online]. Available: http://arxiv.org/abs/1606.06897
[178]. Lashkari, A. F. A. Kadir, H. Gonzalez, K. F. Mbah, and A. Ghorbani, ‘‘Towards a network-based framework for Android mal- ware detection and characterization,’’ in Proc. 15th Annu. Conf. Privacy, Secur. Trust (PST), Aug. 2017, pp. 233–23309.
[179]. Parrend, J. Navarro, F. Guigou, A. Deruyver, and P. Collet, ‘‘Founda- tions and applications of artificial intelligence for zero-day and multi- step attack detection,’’ EURASIP J. Inf. Secur., vol. 2018, no. 1, p. 4, Dec. 2018.
[180]. Arivudainambi, V. K. Ka, and P. Visu, ‘‘Malware traffic classi- fication using principal component analysis and artificial neural net- work for extreme surveillance,’’ Comput. Commun., vol. 147, pp. 50–57, Nov. 2019.
[181]. Y. Ding, S. Chen, and J. Xu, ‘‘Application of deep belief networks for opcode based malware detection,’’ in Proc. Int. Joint Conf. Neural Netw. (IJCNN), Jul. 2016, pp. 3901–3908.
[182]. M. Nadeem, O. Marshall, S. Singh, X. Fang, and X. Yuan, ‘‘Semi- supervised deep neural network for network intrusion detection,’’ in Proc. KSU Cybersecur. Educ., Res. Pract., vol. 2, 2016. [Online]. Available: https://digitalcommons.kennesaw.edu/ccerp/2016/Practice/2
[183]. Raff, J. Barker, J. Sylvester, R. Brandon, B. Catanzaro, and C. K. Nicholas, ‘‘Malware detection by eating a whole exe,’’ in Proc. Workshops 32nd AAAI Conf. Artif. Intell., 2018, pp. 1–13.
[184]. Grosse, N. Papernot, P. Manoharan, M. Backes, and P. McDaniel, ‘‘Adversarial examples for malware detection,’’ in Proc. Eur. Symp. Res. Comput. Secur. Cham, Switzerland: Springer, 2017, pp. 62–79.
[185]. Kolosnjaji, A. Demontis, B. Biggio, D. Maiorca, G. Giacinto, C. Eckert, and F. Roli, ‘‘Adversarial malware binaries: Evading deep learning for malware detection in executables,’’ in Proc. 26th Eur. Signal Process. Conf. (EUSIPCO), Sep. 2018, pp. 533–537.
[186]. Avast Internet Security. Accessed: Feb. 16, 2020. [Online]. Available: https://www.avast.com/en-au/internet-security
[187]. 10 BEST Free Malware Removal Software Of 2020. Accessed: Feb. 16, 2020. [Online]. Available: https://www. softwaretestinghelp.com/best-malware-removal/
[188]. Malwarebytes. Accessed: Feb. 16, 2020. [Online]. Available: https:// www.malwarebytes.com/
[189]. Norton Power Eraser. Accessed: Feb. 16, 2020. [Online]. Available: https://us.norton.com/support/tools/npe.html
[190]. AVG. Accessed: Feb. 16, 2020. [Online]. Available: https:// www.avg.com/en-ww/homepage#pc
[191]. Bitdefender Antivirus. Accessed: Feb. 16, 2020. [Online]. Available: https://www.bitdefender.com/
[192]. Su, J. Tian, X. Chen, and X. Yang, ‘‘A fingerprint authentication system based on mobile phone,’’ in Proc. Int. Conf. Audio Video-Based Biometric Person Authentication. Berlin, Germany: Springer, 2005, pp. 151–159.
[193]. Jamil and M. A. Shah, ‘‘Analysis of machine learning solutions to detect malware in android,’’ in Proc. 6th Int. Conf. Innov. Comput. Technol. (INTECH), Aug. 2016, pp. 226–232.
[194]. Arora, S. Garg, and S. K. Peddoju, ‘‘Malware detection using network traffic analysis in Android based mobile devices,’’ in Proc. 8th Int. Conf. Next Gener. Mobile Apps, Services Technol., Sep. 2014, pp. 66–71.
[195]. Chen, ‘‘Deep transfer learning for static malware classification,’’ 2018, arXiv:1812.07606. [Online]. Available: http://arxiv.org/abs/1812.07606
[196]. Jadhav, D. Vidyarthi, and M. Hemavathy, ‘‘Evolution of evasive malwares: A survey,’’ in Proc. Int. Conf. Comput. Techn. Inf. Commun. Technol. (ICCTICT), Mar. 2016, pp. 641–646.
[197]. Martinelli, F. Mercaldo, A. Saracino, and C. A. Visaggio, ‘‘I find your behavior disturbing: Static and dynamic app behavioral analysis for detection of Android malware,’’ in Proc. 14th Annu. Conf. Privacy, Secur. Trust (PST), 2016, pp. 129–136.c
[198]. De Paola, S. Gaglio, G. L. Re, and M. Morana, ‘‘A hybrid system for malware detection on big data,’’ in Proc. IEEE Conf. Comput. Commun. Workshops (INFOCOM WKSHPS), Apr. 2018, pp. 45–50.
[199]. K. Bartos, M. Sofka, and V. Franc, ‘‘Optimized invariant representation of network traffic for detecting unseen malware variants,’’ in Proc. 25th USENIX Secur. Symp. (USENIX Security), 2016, pp. 807–822.
[200]. P. Wang and Y.-S. Wang, ‘‘Malware behavioural detection and vaccine development by using a support vector model classifier,’’ J. Comput. Syst. Sci., vol. 81, no. 6, pp. 1012–1026, Sep. 2015.
[201]. H.-S. Ham and M.-J. Choi, ‘‘Analysis of Android malware detection performance using machine learning classifiers,’’ in Proc. Int. Conf. ICT Converg. (ICTC), Oct. 2013, pp. 490–495.
[202]. Peiravian and X. Zhu, ‘‘Machine learning for Android malware detec- tion using permission and api calls,’’ in Proc. IEEE 25th Int. Conf. Tools Artif. Intell., Nov. 2013, pp. 300–305.c.
[203]. Bhattacharya and R. T. Goswami, ‘‘DMDAM: Data mining based detection of Android malware,’’ in Proc. 1st Int. Conf. Intell. Comput. Commun. Singapore: Springer, 2017, pp. 187–194.
[204]. O. Sahin, O. E. Kural, S. Akleylek, and E. Kilic, ‘‘New results on permission based static analysis for Android malware,’’ in Proc. 6th Int. Symp. Digit. Forensic Secur. (ISDFS), Mar. 2018, pp. 1–4.
[205]. Tam and A. Hunter, ‘‘Machine learning to identify Android malware,’’ in Proc. 9th IEEE Annu. Ubiquitous Comput., Electron. Mobile Commun. Conf. (UEMCON), Nov. 2018, pp. 1–5
[206]. L. Vaishanav, S. Chauhan, H. Vaishanav, M. S. Sankhla, and R. Kumar, ‘‘Behavioural analysis of Android malware using machine learning,’’ Int. J. Eng. Comput. Sci., vol. 6, no. 5, pp. 1–12, 2017.
[207]. S. Gates, N. Li, H. Peng, B. Sarma, Y. Qi, R. Potharaju, C. Nita-Rotaru, and I. Molloy, ‘‘Generating summary risk scores for mobile appli- cations,’’ IEEE Trans. dependable secure Comput., vol. 11, no. 3, pp. 238–251, Jan. 2014.
[208]. Y. Lu, P. Zulie, L. Jingju, and S. Yi, ‘‘Android malware detection technol- ogy based on improved Bayesian classification,’’ in Proc. 3rd Int. Conf. Instrum., Meas., Comput., Commun. Control, Sep. 2013, pp. 1338–1341.
[209]. Sanz, I. Santos, C. Laorden, X. Ugarte-Pedrero, and P. G. Bringas, ‘‘On the automatic categorisation of Android applications,’’ in Proc. IEEE Consum. Commun. Netw. Conf. (CCNC), Jan. 2012, pp. 149–153.
[210]. Odusami, O. Abayomi-Alli, S. Misra, O. Shobayo, R. Damasevicius, and R. Maskeliunas, ‘‘Android malware detection: A survey,’’ in Proc. Int. Conf. Appl. Informat. Cham, Switzerland: Springer, 2018, pp. 255–266.
[211]. Shobayo, R. Damasevicius, and R. Maskeliunas, ‘‘Android malware detection: A survey,’’ in Proc. 1st Int. Conf. Appl. Inform. (ICAI), vol. 942. Bogotá, Colombia: Springer, Nov. 2018, p. 255.
[212]. Zachariah, K. Akash, M. S. Yousef, and A. M. Chacko, ‘‘Android malware detection a survey,’’ in Proc. IEEE Int. Conf. Circuits Syst. (ICCS), Dec. 2017, pp. 238–244.
[213]. L. Wen and H. Yu, ‘‘An Android malware detection system based on machine learning,’’ in Proc. AIP Conf., vol. 1864, no. 1. Melville, NY, USA: AIP Publishing, 2017, Art. no. 020136.
[214]. Gunalakshmii and P. Ezhumalai, ‘‘Mobile keylogger detection using machine learning technique,’’ in Proc. IEEE Int. Conf. Comput. Commun. Syst. (ICCCS), Feb. 2014, pp. 51–56.
[215]. J. Sahs and L. Khan, ‘‘A machine learning approach to Android mal- ware detection,’’ in Proc. Eur. Intell. Secur. Informat. Conf., Aug. 2012, pp. 141–147.
[216]. W. Li, J. Ge, and G. Dai, ‘‘Detecting malware for Android platform: An SVM-based approach,’’ in Proc. IEEE 2nd Int. Conf. Cyber Secur. Cloud Comput., Nov. 2015, pp. 464–469.
[217]. S. Sheen, R. Anitha, and V. Natarajan, ‘‘Android based malware detection using a multifeature collaborative decision fusion approach,’’ Neurocom- puting, vol. 151, pp. 905–912, Mar. 2015.
[218]. H.-S. Ham, H.-H. Kim, M.-S. Kim, and M.-J. Choi, ‘‘Linear SVM- based Android malware detection,’’ in Frontier and Innovation in Future Computing and Communications. Dordrecht, The Netherlands: Springer, 2014, pp. 575–585.
[219]. Narayanan, L. Chen, and C. K. Chan, ‘‘AdDetect: Automated detection of Android ad libraries using semantic analysis,’’ in Proc. IEEE 9th Int. Conf. Intell. Sensors, Sensor Netw. Inf. Process. (ISSNIP), Apr. 2014, pp. 1–6.
[220]. M. Spreitzenbarth, T. Schreck, F. Echtler, D. Arp, and J. Hoffmann, ‘‘Mobile-sandbox: Combining static and dynamic analysis with machine- learning techniques,’’ Int. J. Inf. Secur., vol. 14, no. 2, pp. 141–153, Apr. 2015.
[221]. Zhu, H. Jin, Y. Yang, D. Wu, and W. Chen, ‘‘DeepFlow: Deep learning- based malware detection by mining Android application for abnormal usage of sensitive data,’’ in Proc. IEEE Symp. Comput. Commun. (ISCC), Jul. 2017, pp. 438–443.
[222]. A. Samra and O. A. Ghanem, ‘‘Analysis of clustering technique in Android malware detection,’’ in Proc. 7th Int. Conf. Innov. Mobile Internet Services Ubiquitous Comput., Jul. 2013, pp. 729–733.
[223]. S. Y. Yerima, S. Sezer, and I. Muttik, ‘‘Android malware detection using parallel machine learning classifiers,’’ in Proc. 8th Int. Conf. Next Gener. Mobile Apps, Services Technol., Sep. 2014, pp. 37–42.
[224]. Z. Yuan, Y. Lu, and Y. Xue, ‘‘Droiddetector: Android malware characteri- zation and detection using deep learning,’’ Tsinghua Sci. Technol., vol. 21, no. 1, pp. 114–123, Feb. 2016.
[225]. Ucci, L. Aniello, and R. Baldoni, ‘‘Survey of machine learning techniques for malware analysis,’’ 2017, arXiv:1710.08189. Online]. Available: http://arxiv.org/abs/1710.08189
[226]. Kaspersky Mobile Antivirus. Accessed: Feb. 16, 2020. [Online]. Avail- able: https://usa.kaspersky.com/android-security
[227]. Norton Security and Antivirus. Accessed: Feb. 16, 2020. [Online]. Avail- able: https://my.norton.com/mobile/home
[228]. Avira Antivirus Security. Accessed: Feb. 16, 2020. [Online]. Available: https://www.avira.com/
[229]. X. Deng, Q. Liu, Y. Deng, and S. Mahadevan, ‘‘An improved method to construct basic probability assignment based on the confusion matrix for classification problem,’’ Inf. Sci., vols. 340–341, pp. 250–261, May 2016.
[230]. M. Farid, N. Harbi, and M. Z. Rahman, ‘‘Combining naive Bayes and decision tree for adaptive intrusion detection,’’ 2010, arXiv:1005.4496. [Online]. Available: https://arxiv.org/abs/1005.4496
[231]. M. S. Mok, S. Y. Sohn, and Y. H. Ju, ‘‘Random effects logistic regres- sion model for anomaly detection,’’ Expert Syst. Appl., vol. 37, no. 10, pp. 7162–7166, Oct. 2010.
[232]. M. M. T. Jawhar and M. Mehrotra, ‘‘Design network intrusion detection system using hybrid fuzzy-neural network,’’ Int. J. Comput. Sci. Secur., vol. 4, no. 3, pp. 285–294, 2010.
[233]. M. Yan and Z. Liu, ‘‘A new method of transductive SVM-based net- work intrusion detection,’’ in Proc. Int. Conf. Comput. Comput. Technol. Agricult. Berlin, Germany: Springer, 2010, pp. 87–95.
[234]. Wagner, J. François, and T. Engel, ‘‘Machine learning approach for ip-flow record anomaly detection,’’ in Proc. Int. Conf. Res. Netw. Berlin, Germany: Springer, 2011, pp. 28–39.
[235]. M. Rahman, D. M. Farid, and M. Z. Rahman, ‘‘Adaptive intrusion detection based on boosting and naive Bayesian classifier,’’ Faculty Paper, 2011. [Online]. Available: http://103.109.52.4:8080/handle/52243/74
[236]. M.-Y. Su, ‘‘Real-time anomaly detection systems for denial-of-service attacks by weighted k-nearest-neighbor classifiers,’’ Expert Syst. Appl., vol. 38, no. 4, pp. 3492–3498, Apr. 2011.
[237]. S. Lee, G. Kim, and S. Kim, ‘‘Self-adaptive and dynamic clustering for online anomaly detection,’’ Expert Syst. Appl., vol. 38, no. 12, pp. 14891–14898, Nov. 2011.
[238]. M. Sheikhan, Z. Jadidi, and A. Farrokhi, ‘‘Intrusion detection using reduced-size RNN based on feature grouping,’’ Neural Comput. Appl., vol. 21, no. 6, pp. 1185–1190, Sep. 2012.
[239]. S. K. Sharma, P. Pandey, S. K. Tiwari, and M. S. Sisodia, ‘‘An improved network intrusion detection technique based on k-means clustering via Naïve Bayes classification,’’ in Proc. IEEE Int. Conf. Adv. Eng., Sci. Manage. (ICAESM), Mar. 2012, pp. 417–422.
[240]. L. Koc, T. A. Mazzuchi, and S. Sarkani, ‘‘A network intrusion detection system based on a hidden Naïve Bayes multiclass classifier,’’ Expert Syst. Appl., vol. 39, no. 18, pp. 13492–13500, Dec. 2012.
[241]. S. S. Sivatha Sindhu, S. Geetha, and A. Kannan, ‘‘Decision tree based light weight intrusion detection using a wrapper approach,’’ Expert Syst. Appl., vol. 39, no. 1, pp. 129–141, Jan. 2012.
[242]. Y. Li, J. Xia, S. Zhang, J. Yan, X. Ai, and K. Dai, ‘‘An efficient intru- sion detection system based on support vector machines and gradually feature removal method,’’ Expert Syst. Appl., vol. 39, no. 1, pp. 424–430, Jan. 2012.
[243]. Chandrashekhar and K. Raghuveer, ‘‘Fortification of hybrid intrusion detection system using variants of neural networks and support vector machines,’’ Int. J. Netw. Secur. Appl., vol. 5, no. 1, p. 71, 2013
[244]. M. M. Lisehroodi, Z. Muda, and W. Yassin, ‘‘A hybrid framework based on neural network MLP and K-means clustering for intrusion detection system,’’ in Proc. 4th Int. Conf. Comput. Inform. (ICOCI), 2013, pp. 1–7.
[245]. S. Devaraju and S. Ramakrishnan, ‘‘Detection of accuracy for intrusion detection system using neural network classifier,’’ Int. J. Emerg. Technol. Adv. Eng., vol. 3, no. 1, pp. 338–345, 2013.
[246]. W. Yassin, N. I. Udzir, Z. Muda, and M. N. Sulaiman, ‘‘Anomaly- based intrusion detection through k-means clustering and Naives Bayes classification,’’ in Proc. 4th Int. Conf. Comput. Inform. (ICOCI), vol. 49, 2013, pp. 1–6.
[247]. Z. A. Baig, S. M. Sait, and A. Shaheen, ‘‘GMDH-based networks for intelligent intrusion detection,’’ Eng. Appl. Artif. Intell., vol. 26, no. 7, pp. 1731–1740, Aug. 2013.
[248]. M. S. Pervez and D. M. Farid, ‘‘Feature selection and intrusion classifi- cation in NSL-KDD cup 99 dataset employing SVMs,’’ in Proc. 8th Int. Conf. Softw., Knowl., Inf. Manage. Appl. (SKIMA), Dec. 2014, pp. 1–6.
[249]. R. T. Kokila, S. T. Selvi, and K. Govindarajan, ‘‘DDoS detection and anal- ysis in SDN-based environment using support vector machine classifier,’’ in Proc. 6th Int. Conf. Adv. Comput. (ICoAC), Dec. 2014, pp. 205–210.
[250]. Saxena and V. Richariya, ‘‘Intrusion detection in KDD99 dataset using SVM-PSO and feature reduction with information gain,’’ Int. J. Comput. Appl., vol. 98, no. 6, pp. 25–29, Jul. 2014.
[251]. K. Shrivas and A. K. Dewangan, ‘‘An ensemble model for classifica- tion of attacks with feature selection based on KDD99 and NSL-KDD data set,’’ Int. J. Comput. Appl., vol. 99, no. 15, pp. 8–13, Aug. 2014.
[252]. R. Ranjan and G. Sahoo, ‘‘A new clustering approach for anomaly intrusion detection,’’ 2014, arXiv:1404.2772. [Online]. Available: http://arxiv.org/abs/1404.2772
[253]. W. Feng, Q. Zhang, G. Hu, and J. X. Huang, ‘‘Mining network data for intrusion detection through combining SVMs with ant colony networks,’’ Future Generat. Comput. Syst., vol. 37, pp. 127–140, Jul. 2014.
[254]. Kim, H. Yi, J. Lee, Y. Paek, and S. Yoon, ‘‘LSTM-based system- call language modeling and robust ensemble method for designing host- based intrusion detection systems,’’ 2016, arXiv:1611.01726. [Online]. Available: http://arxiv.org/abs/1611.01726
[255]. M. V. Kotpalliwar and R. Wajgi, ‘‘Classification of attacks using support vector machine (SVM) on KDDCUP’99 IDS database,’’ in Proc. 5th Int. Conf. Commun. Syst. Netw. Technol., Apr. 2015, pp. 987–990.
[256]. S. Eesa, Z. Orman, and A. M. A. Brifcani, ‘‘A novel feature-selection approach based on the cuttlefish optimization algorithm for intrusion detection systems,’’ Expert Syst. Appl., vol. 42, no. 5, pp. 2670–2679, Apr. 2015.
[257]. W. Masduki, K. Ramli, F. A. Saputra, and D. Sugiarto, ‘‘Study on implementation of machine learning methods combination for improving attacks detection accuracy on intrusion detection system (IDS),’’ in Proc. Int. Conf. Qual. Res. (QiR), Aug. 2015, pp. 56–64.
[258]. N. G. Relan and D. R. Patil, ‘‘Implementation of network intrusion detection system using variant of decision tree algorithm,’’ in Proc. Int. Conf. Nascent Technol. Eng. Field (ICNTE), Jan. 2015, pp. 1–5.
[259]. Hadri, K. Chougdali, and R. Touahni, ‘‘Intrusion detection system using PCA and fuzzy PCA techniques,’’ in Proc. Int. Conf. Adv. Commun. Syst. Inf. Secur. (ACOSIS), Oct. 2016, pp. 1–7.
[260]. Subba, S. Biswas, and S. Karmakar, ‘‘Enhancing performance of anomaly based intrusion detection systems through dimensionality reduc- tion using principal component analysis,’’ in Proc. IEEE Int. Conf. Adv. Netw. Telecommun. Syst. (ANTS), Nov. 2016, pp. 1–6.
[261]. Vivek, S. Nandan, and R. Tiwari, ‘‘Enhanced method for intrusion detection over KDD cup 99 dataset,’’ Int. J. Current Trends Eng. Technol., vol. 2, no. 2, pp. 218–224, 2016. [Online]. Available: https://www.semanticscholar.org/paper/Enhanced-Method- for-Intrusion-Detection-over-KDD-99-Tiwari-Rathore/9b2ed9b997d35839e761f8dbe2b87a0c45cb88e6
[262]. K. Sharma, H. K. Kalita, and P. Borah, ‘‘Analysis of machine learning techniques based intrusion detection systems,’’ in Proc. 3rd Int. Conf. Adv. Comput., Netw. Informat. New Delhi, India: Springer, 2016, pp. 485–493.
[263]. P. U. K. P. chandra and P. N. A. lilhore, ‘‘Network intrusion detection system based on modified Random forest classifiers for KDD cup-99 and NSL-KDD dataset,’’ Int. Res. J. Eng. Technol. (IRJET), vol. 4, no. 8, pp. 786–791, 2017.
[264]. Kwon, H. Kim, J. Kim, S. C. Suh, I. Kim, and K. J. Kim, ‘‘A survey of deep learning-based network anomaly detection,’’ Cluster Comput., vol. 22, pp. 1–13, Jan. 2017.
[265]. Kevric, S. Jukic, and A. Subasi, ‘‘An effective combining classifier approach using tree algorithms for network intrusion detection,’’ Neural Comput. Appl., vol. 28, no. S1, pp. 1051–1058, Dec. 2017.
[266]. R. Syarif and W. Gata, ‘‘Intrusion detection system using hybrid binary PSO and K-nearest neighborhood algorithm,’’ in Proc. 11th Int. Conf. Inf. Commun. Technol. Syst. (ICTS), Oct. 2017, pp. 181–186.
[267]. Xu, S. Chen, H. Zhang, and T. Wu, ‘‘Incremental k-NN SVM method in intrusion detection,’’ in Proc. 8th IEEE Int. Conf. Softw. Eng. Service Sci. (ICSESS), Nov. 2017, pp. 712–717.
[268]. T.-T.-H. Le, J. Kim, and H. Kim, ‘‘An effective intrusion detection classi- fier using long short-term memory with gradient descent optimization,’’ in Proc. Int. Conf. Platform Technol. Service (PlatCon), Feb. 2017, pp. 1–6.
[269]. J. Malik and F. A. Khan, ‘‘A hybrid technique using binary particle swarm optimization and decision tree pruning for network intrusion detection,’’ Cluster Comput., vol. 21, no. 1, pp. 667–680, Mar. 2018.
[270]. M. Al-Hawawreh, N. Moustafa, and E. Sitnikova, ‘‘Identification of malicious activities in industrial Internet of Things based on deep learning models,’’ J. Inf. Secur. Appl., vol. 41, pp. 1–11, Aug. 2018.
[271]. Zhang, Y. Qu, and A. Deng, ‘‘Network intrusion detection using kernel-based fuzzy-rough feature selection,’’ in Proc. IEEE Int. Conf. Fuzzy Syst. (FUZZ-IEEE), Jul. 2018, pp. 1–6.
[272]. J. Lee, J. Kim, I. Kim, and K. Han, ‘‘Cyber threat detection based on artificial neural networks using event profiles,’’ IEEE Access, vol. 7, pp. 165607–165626, 2019.
[273]. Mohammadi, H. Mirvaziri, M. Ghazizadeh-Ahsaee, and H. Karimipour, ‘‘Cyber intrusion detection by combined feature selection algorithm,’’ J. Inf. Secur. Appl., vol. 44, pp. 80–88, Feb. 2019.
[274]. Y. Zhou, G. Cheng, S. Jiang, and M. Dai, ‘‘Building an effi- cient intrusion detection system based on feature selection and ensemble classifier,’’ 2019, arXiv:1904.01352. [Online]. Available: http://arxiv.org/abs/1904.01352
[275]. Y. Zhang, P. Li, and X. Wang, ‘‘Intrusion detection for IoT based on improved genetic algorithm and deep belief network,’’ IEEE Access, vol. 7, pp. 31711–31722, 2019.
[276]. A.-U.-H. Qureshi, H. Larijani, N. Mtetwa, A. Javed, and J. Ahmad, ‘‘RNN-ABC: A new swarm optimization based technique for anomaly detection,’’ Computers, vol. 8, no. 3, p. 59, Aug. 2019.
[277]. O. Faker and E. Dogdu, ‘‘Intrusion detection using big data and deep learning techniques,’’ in Proc. ACM Southeast Conf. (ZZZ ACM SE), 2019, pp. 86–93.
[278]. Salo, A. B. Nassif, and A. Essex, ‘‘Dimensionality reduction with IG- PCA and ensemble classifier for network intrusion detection,’’ Comput. Netw., vol. 148, pp. 164–175, Jan. 2019.
[279]. Ghasemi, J. Esmaily, and R. Moradinezhad, ‘‘Intrusion detection sys- tem using an optimized kernel extreme learning machine and efficient features,’’ Sadhana, vol. 45, no. 1, pp. 1–9, Dec. 2020.
[280]. Sen, K. D. Gupta, and M. M. Ahsan, ‘‘Leveraging machine learning approach to setup software-defined network (SDN) controller rules dur- ing DDoS attack,’’ in Proc. Int. Joint Conf. Comput. Intell. Singapore: Springer, 2020, pp. 49–60.
[281]. Z. Liu, Y. Zhu, X. Yan, L. Wang, Z. Jiang, and J. Luo, ‘‘Deep learning approach for IDS,’’ in Proc. 4th Int. Congr. Inf. Commun. Technol. Singapore: Springer, 2020, pp. 471–479.
[282]. M. Sarnovsky and J. Paralic, ‘‘Hierarchical intrusion detection using machine learning and knowledge model,’’ Symmetry, vol. 12, no. 2, p. 203, Feb. 2020.
[283]. Anderson, D. Quist, J. Neil, C. Storlie, and T. Lane, ‘‘Graph-based malware detection using dynamic analysis,’’ J. Comput. Virol., vol. 7, no. 4, pp. 247–258, Nov. 2011.
[284]. Isohara, K. Takemori, and A. Kubota, ‘‘Kernel-based behavior analysis for Android malware detection,’’ in Proc. 7th Int. Conf. Comput. Intell. Secur., Dec. 2011, pp. 1011–1015.
[285]. Kavzoglu and I. Colkesen, ‘‘The effects of training set size for perfor- mance of support vector machines and decision trees,’’ in Proc. 10th Int. Symp. Spatial Accuracy Assessment Natural Resour. Environ. Sci., 2012, p. 1013.
[286]. Mohaisen and O. Alrawi, ‘‘Unveiling zeus: Automated classification of malware samples,’’ in Proc. 22nd Int. Conf. World Wide Web (WWW Companion), 2013, pp. 829–832.
[287]. R. Islam, R. Tian, L. M. Batten, and S. Versteeg, ‘‘Classification of mal- ware based on integrated static and dynamic features,’’ J. Netw. Comput. Appl., vol. 36, no. 2, pp. 646–656, Mar. 2013.
[288]. Liangboonprakong and O. Sornil, ‘‘Classification of malware families based on N-grams sequential pattern features,’’ in Proc. IEEE 8th Conf. Ind. Electron. Appl. (ICIEA), Jun. 2013, pp. 777–782.
[289]. Bhat, S. Patra, and D. Jena, ‘‘Machine learning approach for intrusion detection on cloud virtual machines,’’ Int. J. Appl. Innov. Eng. Manage., vol. 2, no. 6, pp. 56–66, 2013.
[290]. Z. Salehi, A. Sami, and M. Ghiasi, ‘‘Using feature generation from API calls for malware detection,’’ Comput. Fraud Secur., vol. 2014, no. 9, pp. 9–18, Sep. 2014.
[291]. M. Z. Mas’ud, S. Sahib, M. F. Abdollah, S. R. Selamat, and R. Yusof, ‘‘Analysis of features selection and machine learning classifier in Android malware detection,’’ in Proc. Int. Conf. Inf. Sci. Appl. (ICISA), May 2014, pp. 1–5.
[292]. P. V. Shijo and A. Salim, ‘‘Integrated static and dynamic analysis for mal- ware detection,’’ Procedia Comput. Sci., vol. 46, pp. 804–811, Jan. 2015.
[293]. Y. Li, R. Ma, and R. Jiao, ‘‘A hybrid malicious code detection method based on deep learning,’’ Int. J. Secur. Appl., vol. 9, no. 5, pp. 205–216, May 2015.
[294]. M. Khammas, A. Monemi, J. S. Bassi, I. Ismail, S. M. Nor, and M. N. Marsono, ‘‘Feature selection and machine learning classification for malware detection,’’ J. Teknologi, vol. 77, no. 1, pp. 1–9, Oct. 2015.
[295]. -I. Fan, H.-W. Hsiao, C.-H. Chou, and Y.-F. Tseng, ‘‘Malware detection systems based on API log data mining,’’ in Proc. IEEE 39th Annu. Comput. Softw. Appl. Conf., vol. 3, Jul. 2015, pp. 255–260.
[296]. Z. Khan and U. Qamar, ‘‘Text mining approach to detect spam in emails,’’ in Proc. Int. Conf. Innov. Intell. Syst. Comput. Technol. (ICIISCT), 2016, p. 45.
[297]. Hardy, L. Chen, S. Hou, Y. Ye, and X. Li, ‘‘DL4MD: A deep learning framework for intelligent malware detection,’’ in Proc. Int. Conf. Data Mining, Steering Committee World Congr. Comput. Sci. (DMIN), 2016, p. 61.
[298]. S. Galal, Y. B. Mahdy, and M. A. Atiea, ‘‘Behavior-based features model for malware detection,’’ J. Comput. Virol. Hacking Techn., vol. 12, no. 2, pp. 59–67, May 2016.
[299]. Karim, R. Salleh, and M. K. Khan, ‘‘SMARTbot: A behavioral analysis framework augmented with machine learning to identify mobile botnet applications,’’ PLoS ONE, vol. 11, no. 3, Mar. 2016, Art. no. e0150077.
[300]. Y. Cheng, W. Fan, W. Huang, and J. An, ‘‘A shellcode detection method based on full native API sequence and support vector machine,’’ in Proc. IOP Conf., Mater. Sci. Eng., vol. 242, no. 1. Bristol, U.K.: IOP Publishing, 2017, Art. no. 012124.
[301]. Moon, H. Im, I. Kim, and J. H. Park, ‘‘DTB-IDS: An intrusion detec- tion system based on decision tree using behavior analysis for preventing APT attacks,’’ J. Supercomput., vol. 73, no. 7, pp. 2881–2895, Jul. 2017.
[302]. R. Mosli, R. Li, B. Yuan, and Y. Pan, ‘‘A behavior-based approach for malware detection,’’ in Proc. IFIP Int. Conf. Digit. Forensics. Cham, Switzerland: Springer, 2017, pp. 187–201.
[303]. Z. Cheng, X. Chen, Y. Zhang, S. Li, and Y. Sang, ‘‘Detecting information theft based on mobile network flows for Android users,’’ in Proc. Int. Conf. Netw., Archit., Storage (NAS), Aug. 2017, pp. 1–10.
[304]. R. Nix and J. Zhang, ‘‘Classification of Android apps and malware using deep neural networks,’’ in Proc. Int. Joint Conf. Neural Netw. (IJCNN), May 2017, pp. 1871–1878.
[305]. S. Hou, A. Saas, L. Chen, Y. Ye, and T. Bourlai, ‘‘Deep neural networks for automatic Android malware detection,’’ in Proc. IEEE/ACM Int. Conf. Adv. Social Netw. Anal. Mining, Jul. 2017, pp. 803–810.
[306]. H.-J. Zhu, T.-H. Jiang, B. Ma, Z.-H. You, W.-L. Shi, and L. Cheng, ‘‘HEMD: A highly efficient random forest-based malware detection framework for android,’’ Neural Comput. Appl., vol. 30, no. 11, pp. 3353–3361, Dec. 2018.
[307]. P. Feng, J. Ma, C. Sun, X. Xu, and Y. Ma, ‘‘A novel dynamic Android malware detection system with ensemble learning,’’ IEEE Access, vol. 6, pp. 30996–31011, 2018.
[308]. P. Yan and Z. J. S. Q. J. Yan, ‘‘A survey on dynamic mobile malware detection,’’ Softw. Qual. J., vol. 26, no. 3, pp. 891–919, 2018.
[309]. T. D. Phan and N. Zincir-Heywood, ‘‘User identification via neural network based language models,’’ Int. J. Netw. Manage., vol. 29, no. 3, p. e2049, May 2019.
[310]. S. Arshad, M. A. Shah, A. Wahid, A. Mehmood, H. Song, and H. Yu, ‘‘SAMADroid: A novel 3-Level hybrid malware detection model for Android operating system,’’ IEEE Access, vol. 6, pp. 4321–4339, 2018.
[311]. B. Karbab, M. Debbabi, A. Derhab, and D. Mouheb, ‘‘MalDozer: Automatic framework for Android malware detection using deep learn- ing,’’ Digit. Invest., vol. 24, pp. S48–S59, Mar. 2018.
[312]. Hasegawa and H. Iyatomi, ‘‘One-dimensional convolutional neural networks for Android malware detection,’’ in Proc. IEEE 14th Int. Colloq. Signal Process. Appl. (CSPA), Mar. 2018, pp. 99–102.
[313]. Alshahrani, H. Mansourt, S. Thorn, A. Alshehri, A. Alzahrani, and H. Fu, ‘‘DDefender: Android application threat detection using static and dynamic analysis,’’ in Proc. IEEE Int. Conf. Consum. Electron. (ICCE), Jan. 2018, pp. 1–6.
[314]. S. Naz and D. K. Singh, ‘‘Review of machine learning methods for windows malware detection,’’ in Proc. 10th Int. Conf. Comput., Commun. Netw. Technol. (ICCCNT), Jul. 2019, pp. 1–6.
[315]. Sethi, R. Kumar, L. Sethi, P. Bera, and P. K. Patra, ‘‘A novel machine learning based malware detection and classification framework,’’ in Proc. Int. Conf. Cyber Secur. Protection Digit. Services (Cyber Secur.), Jun. 2019, pp. 1–4.
[316]. Sayadi, H. M. Makrani, S. M. Pudukotai Dinakarrao, T. Mohsenin, Sasan, S. Rafatirad, and H. Homayoun, ‘‘2SMaRT: A two-stage machine learning-based approach for run-time specialized hardware- assisted malware detection,’’ in Proc. Design, Autom. Test Eur. Conf. Exhib. (DATE), Mar. 2019, pp. 728–733.
[317]. Mehtab, W. B. Shahid, T. Yaqoob, M. F. Amjad, H. Abbas, H. Afzal, and M. N. Saqib, ‘‘AdDroid: Rule-based machine learning framework for Android malware analysis,’’ Mobile Netw. Appl., vol. 25, no. 1, pp. 180–192, Feb. 2020.
[318]. Mercaldo and A. Santone, ‘‘Deep learning for image-based mobile malware detection,’’ J. Comput. Virol. Hacking Techn., vol. 16, pp. 1–15, Jan. 2020.
[319]. Z. Ma, H. Ge, Z. Wang, Y. Liu, and X. Liu, ‘‘Droidetec: Android malware detection and malicious code localization through deep learning,’’ 2020, arXiv:2002.03594. [Online]. Available: http://arxiv.org/abs/2002.03594
[320]. Naeem, F. Ullah, M. R. Naeem, S. Khalid, D. Vasan, S. Jabbar, and S. Saeed, ‘‘Malware detection in industrial Internet of Things based on hybrid image visualization and deep learning model,’’ Ad Hoc Netw., vol. 105, Aug. 2020, Art. no. 102154.
[321]. Pei, L. Yu, and S. Tian, ‘‘AMalNet: A deep learning framework based on graph convolutional networks for malware detection,’’ Comput. Secur., vol. 93, Jun. 2020, Art. no. 101792.